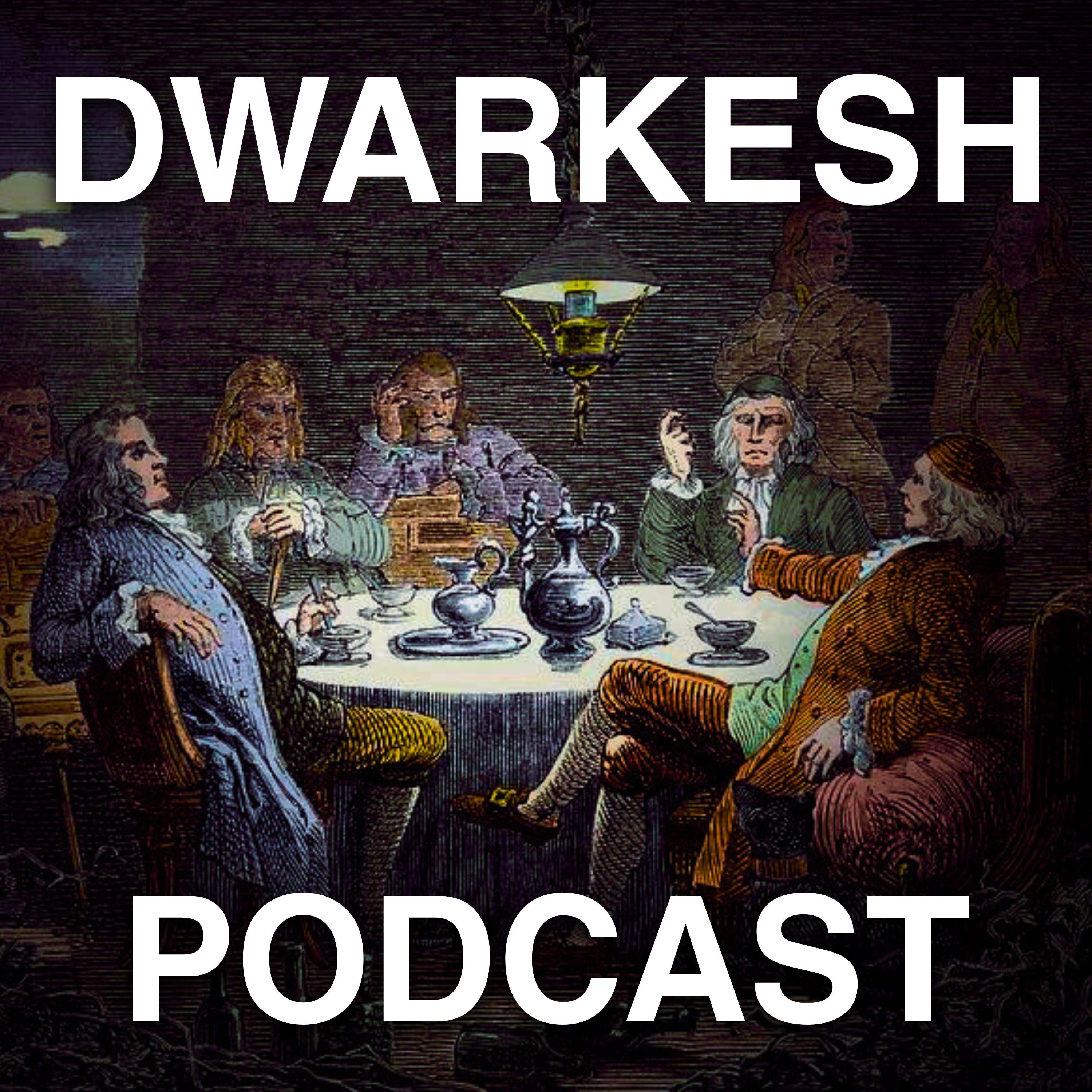
AGI is Still 30 Years Away — Ege Erdil & Tamay Besiroglu
Ege Erdil and Tamay Besiroglu have 2045+ timelines, think the whole "alignment" framing is wrong, don't think an intelligence explosion is plausible, but are convinced we'll see explosive economic growth (economy literally doubling every year or two).
This discussion offers a totally different scenario than my recent interview with Scott and Daniel.
Ege and Tamay are the co-founders of Mechanize (disclosure - I’m an angel investor), a startup dedicated to fully automating work. Before founding Mechanize, Ege and Tamay worked on AI forecasts at Epoch AI.
Watch on Youtube; listen on Apple Podcasts or Spotify.
----------
Sponsors
* WorkOS makes it easy to become enterprise-ready. With simple APIs for essential enterprise features like SSO and SCIM, WorkOS helps companies like Vercel, Plaid, and OpenAI meet the requirements of their biggest customers. To learn more about how they can help you do the same, visit workos.com
* Scale’s Data Foundry gives major AI labs access to high-quality data to fuel post-training, including advanced reasoning capabilities. If you’re an AI researcher or engineer, learn about how Scale’s Data Foundry and research lab, SEAL, can help you go beyond the current frontier at scale.com/dwarkesh
* Google's Gemini Pro 2.5 is the model we use the most at Dwarkesh Podcast: it helps us generate transcripts, identify interesting clips, and code up new tools. If you want to try it for yourself, it's now available in Preview with higher rate limits! Start building with it today at aistudio.google.com.
----------
Timestamps
(00:00:00) - AGI will take another 3 decades
(00:22:27) - Even reasoning models lack animal intelligence
(00:45:04) - Intelligence explosion
(01:00:57) - Ege & Tamay’s story
(01:06:24) - Explosive economic growth
(01:33:00) - Will there be a separate AI economy?
(01:47:08) - Can we predictably influence the future?
(02:19:48) - Arms race dynamic
(02:29:48) - Is superintelligence a real thing?
(02:35:45) - Reasons not to expect explosive growth
(02:49:00) - Fully automated firms
(02:54:43) - Will central planning work after AGI?
(02:58:20) - Career advice
Get full access to Dwarkesh Podcast at www.dwarkesh.com/subscribe
Listen and Follow Along
Full Transcript
Today, I'm chatting with Tame Besaroglu and Ege Erdo.
They were previously running Epoch AI and are now launching Mechanize,
which is a company dedicated to automating all work.
One of the interesting points you made recently, Tame,
is that the whole idea of the intelligence explosion is mistaken or misleading.
Why don't you explain what you were talking about there?
Yeah, I think it's not a very useful concept.
It's kind of like calling the industrial revolution a horsepower explosion.
Like, sure, during the Industrial Revolution, we saw this drastic acceleration in raw physical power. But there are many other things that were maybe equally important in explaining the acceleration of growth and technological change that we saw during the Industrial Revolution.
What is a way to characterize the broader set of things that the horsepower perspective would miss about the Industrial Revolution? So I think in the case of the Industrial Revolution, it was a bunch of these complementary changes to many different sectors in the economy. So you had agriculture, you had transportation, you had law and finance, you had urbanization and moving from rural areas into cities.
There were just many different innovations that kind of happened simultaneously that gave rise to this change in the way of economically organizing our society. It wasn't just that we had more horsepower.
I mean, that was part of it. But that's not the kind of central thing to focus on when thinking about the Industrial Revolution.
And I think similarly for the development of AI, sure, we'll get like a lot of very smart AI systems, but that will be one part among very many different moving parts that explain, you know, why we expect to get this transition and this acceleration and growth and technological change. Yeah.
I want to better understand how you think about that broader transformation. Before we do, the other really interesting part of your worldview is that you have longer timelines to get to AGI than most of the people in San Francisco who think about AI.
When do you expect a drop in remote worker replacement? Yeah, maybe for me that would be around like 2045 or... Wow.
Wait, and you? I'm a little bit more bullish. I mean, it depends what you mean by drop in remote worker and whether it's able to do like literally everything that can be done remotely or do most things.
I'm saying literally everything. For literally everything, yeah.
Just shade, I guess, predictions by five years or like by 20% or something. Why? Because we've seen so much progress over even the last few years.
We've gone from ChadGBT like two years ago to now we have models that can literally do reasoning, are better coders than me. And I studied software engineering in college.
I mean, I did become a podcaster. I'm not saying I'm the best coder in the world.
But if you made this much progress in the last two years,
why would it take another 30 to get to full automation of human brains?
Right.
I said that wrong.
You know what I'm saying?
Full automation of remote work.
Yeah, yeah.
So I think that a lot of people have this intuition that progress has been very fast.
They just look at the trend lines and just extrapolate.
Obviously, it's going to happen in, I don't know, 2027 or 2030 or whatever.
They're just very bullish.
And obviously, that's not a thing you can literally do.
There isn't a trend you can literally extrapolate of when do we get the full automation.
Because if you look at the fraction of the economy that has actually been automated,
it's very, like by AI, it's very small.
So if you just extrapolate that trend, which is something, say, Robin Hanson likes to do,
you're going to say, well, it's going to take centuries or something.
Now, we don't agree with that view.
But I think one way of thinking about this is like, how many big things are there? How many core capabilities, competences are there that the AI systems need to be good at in order to have this very broad economic impact, maybe 10x acceleration and growth or something? How many things have you gotten over the past 10 years, 15 years? And we also have this compute-centric view. So just to double click on that, I think what Ege is referring to is if you look at the past 10 years, 15 years.
And we also have this compute-centric view. So just to double-click on that, I mean, I think what Ege is referring to is like, if you look at the past 10 years of AI progress, we've gone through about 9 or 10 orders of magnitude of compute, and we've got various capabilities that were unlocked.
So you had, in the early period, people were solving gameplay on specific games, on very complex games.
And that happened 2015 to maybe 2020 and Go and Chess and Dota and other games.
And then you had maybe sophisticated language capabilities that were unlocked with these large language models
and maybe kind of advanced abstract reasoning.
And coding and maybe math, that was maybe another big such capability that got unlocked.
And so maybe there are a couple of these big unlocks that happened over the past 10 years.
But it takes, you know, that happened on the order of once every three years or so, or maybe one every three orders of magnitude of compute scaling. And so, and then you might ask the question, how many more such competencies might we need to unlock in order to be able to have an AI system that can match the capabilities of humans across the board, maybe specifically just on remote work tasks.
And so then you might ask, well, maybe you need kind of coherence over very long horizons, or you need kind of agency and autonomy, or maybe you need multimodal, kind of full multimodal kind of understanding, just like a human would. And then you ask the question, okay, how long might that take? And so you can think about, well, just in terms of calendar years, the previous unlocks took about, you get one every three years or so.
But of course, that previous period coincided with this rapid scale up of the amount of compute that we use for training. So we went through maybe nine or 10 orders of magnitude since AlexNet, you know, compared to the biggest models we have today.
And, you know, we're getting to a level where it's becoming harder and harder to scale up compute. And, you know, we've done some extrapolations and some analysis looking at specific constraints like energy or GPU production.
And based on that, it looks like we might have maybe three or four orders of magnitude of scaling left, and then you're really spending a pretty sizable fraction or a non-trivial fraction of world output on just building up data centers, energy infrastructure, fabs. Which is already like 2% of GDP, right? I mean, currently it's less than 2%.
Yeah, but also currently most of it is actually not going towards AI chips. Even most TSMC capacity currently is going towards mobile phone chips or something like that, right? Even leading edge is going.
Yeah, even leading edge is pretty small. But yeah, so that suggests that we might need a lot more compute scaling to get these additional capabilities to be unlocked.
And then there's a question of do we really have that in us as an economy to be able to sustain that scaling? But it seems like you have this intuition that there's just a lot left to intelligence. When you play these models, it's like they're almost there.
It's like you forget you're often talking to an AI. What do you mean they're almost there? I don't know.
I can't ask Claude to pick up this cup and put it over there. Remote work, you know? Okay, but even for remote work, I can't ask Claude to, I think the current computer use systems can't even book a flight properly.
How much of an update would it be if by the end of 2026 they could book a flight? I probably think by the end of this year they're going to be able to do that. But that's like a very, very, like nobody gets a job where they're paid to like book flights for.
Like that's not a task. I think some people.
I mean, if it's literally just book flight. You know, and without, you know.
But I think that's an important point because a lot of people like look at jobs in the economy and then they're like, oh, like that person, like their job is to just do X. But then that's not true.
Like that's something they do in their job. But it's probably if you look at the fraction of their time on the job that they spend on doing that is a very small fraction of what they should do.
It's just this popular conception people have. Or travel agents, like they just book hotels and flights.
But that's not actually most of their job. So automating that actually wouldn't automate their job and it wouldn't have that much of an impact on the economy.
So I think this is actually an important thing, that important worldview difference that separates us from people who are much more bullish because they think like jobs in the economy are much simpler in some sense, and they're going to take like much fewer competences to actually full automate. So our friend Leopold has this perspective of quote-unquote unhobblings where the way to characterize it might be like they're basically like baby AGIs already and then there's because of the constraints we artificially impose upon them by for example only training them on text and not giving them the training data that is necessary for them to understand a Slack environment or a Gmail environment.
Or previously before inference time scaling, not giving them the chance to meditate upon what they're saying and really think it through and not giving them the context about what is actually involved in this job, only giving them this piecemeal, a couple minutes worth of context in the prompt. We're holding back what is fundamentally a little intelligence from being as productive as it could be, which implies that unhoveling just seems easier to solve for than entirely new capabilities of intelligence.
What do you make of that framework? I mean, I guess you could have made similar points five years ago and say, you know, you look at AlphaZero and there's this mini AGI there. And if only you unhobbled it by training it on text and giving it all your context and so on, like that just wouldn't really have worked.
Like, I think you do really need to rethink how you train these models in order to get these capabilities, you know. But I think like the surprising thing over the last few years has been that you can start off with this pre-trained corpus of the internet and it just like, it's actually quite easy.
Like chat GPT is an example of this like unhbling, where 1% of additional compute spent on getting it to talk in a chatbot-like fashion with post-training is enough to make it competent, really competent at that capability. So why not think that agency...
I mean, reasoning is another example where it seems like the amount of compute that is spent on RL right now in these models is a small fraction of total compute. Again, like reasoning seems like complicated and then you just like do 1% of compute and it gets you that.
Why not think that computer use or long-term agency on computer use is a similar thing? So when you say reasoning is easy and it only took this much compute and it wasn't very much and maybe you look at the sheer number of tokens and it wasn't very much and so it looks easy. Well, that's kind of true from our position today.
But I think if you ask someone, build a reasoning model in 2015, then it would have looked insurmountable. You would have had to train a model on tens of thousands of GPUs.
You would have had to solve that problem. And each order of magnitude of scaling from where they were would pose new challenges that they would need to solve.
You would need to produce kind of internet scale or tens of trillions of tokens of data in order to actually train a model that kind of has the knowledge that you can then unlock and access by way of training it to be a reasoning model. You need to maybe make the model more efficient at kind of doing inference and maybe distill it because if it's very slow, then you have a reasoning model that's not particularly useful.
So you also need to make various innovations to get the model to be distilled so that you can train it more quickly because these rollouts take very long. It actually becomes a product that's valuable if it's a couple tokens a second as a reasoning model that would have been very difficult to work with.
So in some sense, it looks easy from our point of view, standing on this huge stack of technology that we've built up over the past five years or so. But at the time, it would have been very hard.
And so my claim would be something like, I think the agency part might be easy in a similar sense, that in five years or three years time or whatever, we will look at what unlocked agency and it'll look fairly simple. But the amount of work that, you know, in terms of these complementary kind of innovations that enable the model to be able to learn how to become a competent agent, that might have just been very difficult and taken years of innovation and a bunch of improvements in kind of hardware and scaling and various other things.
Yeah, I feel like what's dissimilar between 2015 and now, in 2015, if you were trying to solve reasoning, you just didn't have a base to start on.
I don't know.
Maybe you would try to like form a proof methods or something. But like there was no leg to stand on where now you'd actually like you have the thing.
You have the pre-trained base model. You have these techniques of scaffolding, of post-trading, of RL.
And so it seems like are skeptical that – you think that those will look to the future as, say, AlphaGo looks to us now in terms of the basis of a broader intelligence. Why – yeah, and I'm curious if you have intuitions on – why not think that language models as we have them now are like – we got the big missing piece right, and now we're just plugging things on top of it.
Well, I mean, I guess what is the reason for believing that? I mean, you could have looked at AlphaGo or AlphaGo Zero, AlphaZero. Those seemed very impressive at the time.
I mean, you're just learning to play this game with no human knowledge. You're just learning to play it from scratch.
And I think at the time, it did impress a lot of people. But then people tried to apply it to math.
They tried to apply it to other domains. And it didn't work very well.
They weren't able to get competent agents at math. So it's very possible that these models, at least the way we have them right now, you're going to try to do
the same thing people did for reasoning, but for agency, it's not going to work very well.
And then you're not going to...
I'm sorry, you think it will. You're saying at the end of 2026, we will have
agentic computer use.
I think I guess you said you'd be able to book a flight, which is very different from having
full agentic computer use like a human.
The other things you need to do on a computer is just made up of things like booking a flight. Sure, but like they are not like they are not disconnected tasks.
That's like saying like everything you do in the world is just like you just move parts of your body and then you like move your mouth, your tongue, and then you like roll your head. But like that's that's a, yeah, like individually those things are simple.
But then how do you put them together, right? Yeah. Okay.
So there's like two pieces of evidence that you can have that are quite dissimilar. One, the meter eval, which we've been talking about privately, which shows that the task length over certain kinds of tasks, I can already see you getting ready, has been double, AI's ability to do the kind of thing that it takes a human 10 minutes to do or an hour to do or four hours to do.
The length of time for a course running human task, it seems like these models seem to be doubling their task length every seven months. The idea being that by 2030, if you extrapolate this curve, they could be doing tasks that take humans one month to do or one year to do.
And then this like long-term coherency in executing on tasks is like fundamentally what intelligence is. So this curve suggests that like we're getting there.
The other piece of evidence, I kind of feel like my own mind works this way of I get distracted easily and it's like kind of hard to keep a long-term plan in my head at the same time and I'm like slightly better at it than these models but they don't seem like that dissimilar to me and they're I mean even like I would have guessed reasoning is just like a really complicated thing and then it seems like oh oh, it's just something like learning 10 tokens worth of MCTS, of wait, let's go back, let's think about this another way. Shade of thought alone just gets you this boost.
And so it just seems like intelligence is simpler than we thought. Maybe agency is also simpler in this way.
Yeah. I mean, I would say that reasoning did seem, I mean, I think there's a reason to expect complex reasoning to not be as difficult as people might have thought, even in advance.
Because a lot of the tasks that AI solved very early on were tasks of various kinds of complex reasoning. So it wasn't the kind of reasoning that goes into when a human solves a math problem.
But if you look at the major AI milestones over, I don't know, since 1950, a lot of them are for complex reasoning. Like a chess is, you can say, a complex reasoning task.
Go is, you could say, a complex reasoning task. I think there are also examples of long-term agency.
Like winning at StarCraft is an example of being agentic over a meaningful period of time. That's right.
So the problem in that case is that it's a very specific, narrow environment. You can say that playing Go or playing chess, that also requires a certain amount of agency.
And that's true. But it's a very narrow task.
So that's like saying if you construct a software system that is able to react to like very specific, very particular kind of images, or very specific video feeds or whatever, then you're getting close to general sensory motor skill automation. But the general skill is something that's very different.
And I think we're seeing that. We're like, we can't, like we still are very far, it seems like, from an AI model that can take a generic game off Steam.
Let's say you just download a game released this year. You don't know how to play this game.
And then you just have to play it, right? And then most games are actually not that difficult for a human. I mean, what about Cloud Pace Pokemon? I don't think it was trained on Pokemon.
Right, so that's an interesting example. First of all, I find the example very interesting because yeah, it was not trained explicitly, like it wasn't they didn't do some RL on like playing Pokemon Red, but obviously the model knows that it's supposed to play Pokemon Red because there's tons of material about Pokemon Red on the internet.
In fact, if you were playing Pokemon Red and you got stuck somewhere, you didn't know what to do, you could probably go to Claude and ask it, Claude, I'm stuck in Mount Moon. What am I supposed to do? And then it could probably be able to give you a fairly decent answer.
But that doesn't stop it from getting stuck in Mount Moon for 48 hours. So that's a very interesting thing where it has explicit knowledge, but then when it's actually playing the game, it doesn't behave in a way which reflects that it has that knowledge.
All it's got to do is plug the explicit knowledge to its actions. But is that easy? I'm not sure I understand why.
Okay, if you can leverage your knowledge from pre-training about these games in order to be somewhat competent at them, I feel like that is some evidence of okay, they're going to be using, they're going to be leveraging a different base of skills but with that same leverage, they're going to have like a similar repertoire of abilities, right? If like you've read everything about whatever skill that every human has ever seen. I mean, a lot of the skills that people have that we don't have very good training data for them.
That's right. That's right.
What would you want to see over the next few years that would make you think, oh, no, I'm actually wrong. And this was like the last unlock.
And it was like now just a matter of ironing out the kinks. And then we get the thing that will kick off the dare I say intelligence explosion.
Yeah, so I think something that would reveal its ability to do very long context things, use multimodal capabilities in a meaningful way and integrate that with reasoning and other types of systems. And also agency and being able to take action over a long horizon and accomplish some tasks that takes very long for humans to do, not just in specific software environments, but just very broadly, say, downloading an arbitrary game from Steam and something that's never seen before, it doesn't really have much training data, maybe it was released after its training cutoff.
And so there's no tutorials or maybe there's no earlier versions
of the game that has been discussed on the internet. And then accomplishing that game and actually playing that game to the end and accomplishing these various milestones that are challenging for humans, that would be a substantial update.
I mean, there are other things that would update me too like you know open ai making a lot more revenue or than um than it's currently doing is 100 billion in revenue that would in according to a contract mark them as agi enough i i think that's you know not a huge update to me if that were to happen um so i think the update would come if it was in fact 500 billion billion in revenue or something like that. Then I would certainly update quite a lot.
But $100 billion, that seems pretty kind of likely to me. I would assign that maybe, I don't know, 40% chance or something.
I mean, what is this like? If you've got a system that just produced our surplus terms worth $100 billion, the difference between this and alpha zero is alpha zero is never going to make 100 billion dollars i agree in the marketplace right so just the what is intelligence it's like you something able to usefully accomplish its goals or your goals um 100 if people are willing to pay 100 billion dollars for it that's pretty good evidence that it's like accomplishing some goals. Sure.
I mean, people pay $100 billion for all sorts of things. That itself is not a very strong piece of evidence that it's going to be transformative.
People pay trillions of dollars for oil. Oil is not.
I don't know. It seems like a very basic point, but the fact that people pay a lot of money for something doesn't mean it's going to transform the world economy if only we manage to unhubble it.
That's a very different claim, right? Look, a ton of B2B software companies start off by building self-serve consumer-grade products, and that's fine at first. Eventually, though, you have to go after enterprise.
The most successful and durable software companies of the last decade have all made this transition. But getting enterprise ready is hard.
Single sign-on, role-based access controls, and comprehensive audit logs are all actually quite complex and tedious to build. And they're ripe for bugs and annoying edge cases.
These features take a ton of engineering time and capital, which you should be spending on the core product. For example, one of Slack's PMs said that they $30 million building these features and they were only half done.
That's where WorkOS comes in. WorkOS has helped Vercel, Plaid, Vanta, OpenAI, and hundreds of others become enterprise ready with APIs to integrate all of these common features.
If you want to learn more, go to workos.com and tell them that I sent you. Okay, so then this brings us to the intelligence explosion because what people will say is we don't need to automate literally everything that is needed for automating remote work, let alone all human labor in general.
We just need to automate the things which are necessary to fully close the R&D cycle needed to make smarter intelligences. And if you do this, you get a very rapid intelligence explosion.
And the end product of that explosion is not only an AGI, but something that is super human potentially. These things are extremely good at coding.
They're good at the kinds of things that you would think and reasoning. And like, it seems like the kinds of things that would be necessary to automate R&D at AI labs.
What do you make of that logic? I mean, I think if you look at their capability profile, it is like, like if you compare it to like a random job in the economy, I agree they are better at doing sort of coding tasks that will be involved in R&D compared to like a random job in the economy. I agree they are better at doing sort of coding tasks that will be involved in R&D compared to like a random job in the economy.
But in absolute terms, I don't think they're like that good. I think they are good at things that maybe impress us about human coders.
Like if you were wanting to see like, oh, like what makes a person a really impressive coder? You might look at their competitive programming performance. I mean, in fact, companies often hire people based on, if they're relatively junior, based on their performance on these kinds of problems.
But that is just impressive in the human distribution. So if you look in absolute terms at what are the skills you need to actually automate the process of being a researcher, then what fraction of those skills do the AI systems actually have? Even in coding, like a lot of coding is you have a very large code base you have to work with, the instructions are very kind of vague.
There isn't, for example, you mentioned meter eval, in which because they needed to make it an eval, all the tasks have to be kind of compact and closed and have clear evaluation metrics. Like here's a model, like get its loss on this, you know, data set as low as possible or whatever.
Or like here's another model and it's like it's embedding matrix has been scrambled. Just fix it to recover like most of its original performance, et cetera.
Those are not problems that you actually work on in AI R&D. They're like very artificial problems.
Now, if a human was good at doing those problems, you would infer, I think logically, that that human is likely to actually be a good researcher. But if an AI is able to do them, like the AI lacks so many other competences that a human would have, not just the researcher, just an ordinary human that we don't think about in the process of research.
So our view would be automating research is, first of all, more difficult than people can be created for. I think you need more skills to do it and definitely more than models are displaying right now.
And on top of that, even if you did automate the process of research, we think a lot of the software progress has been driven not by cognitive efforts, though that has played a part, but it has been driven by compute scaling. We just have more GPUs, you can do more experiments, you can do more things, your experiments can be done at larger scales.
And that is just a very important driver. Like if you're 10 if you're 10 years ago, 15 years ago, you're trying to figure out what software innovations are going to be important in 10 or 15 years, you would have had a very difficult time.
In fact, you probably wouldn't even conceive of the right kind of innovations to be looking at, because you would be so far removed from the context of that time with much more abundant compute and all the things that people would have learned by that point. So these are two components of our view.
Research is harder than people think and depends a lot on compute scale. Can you put a finer point on what is the kind of thing, what is an example of the kind of task which is very dissimilar from train a classifier or debug a classifier that is relevant to AIR&D? I think it's like, you know, examples might be introducing novel, having novel innovations that are very useful for unlocking innovations in the future.
So that might be, you know, introducing some novel way of thinking about a problem or introducing... So maybe a good example might be in mathematics, where we have these reasoning models that are extremely good at solving math problems.
I mean, very short horizon. Sure.
Maybe not extremely good, but certainly better than I can and better than maybe most undergrads can. And so, you know, they can do that very well, but they're not very good at coming up with novel conceptual schemes that are useful for making progress in mathematics.
So, you know, it's able to solve these problems that you can kind of neatly excise out of some very messy context, and it's able to make a lot of progress there. But within some much messier context, it's kind of not very good at figuring out what directions are especially useful for you to build things or kind of make incremental progress on that enables you to have a big kind of innovation later down the line.
So thinking about both this like larger context as well as maybe much longer horizon, much fuzzier things that you're optimizing for, I think it's much worse at those types of things. Right.
So I think one interesting thing is if you just look at these reasoning models, they know so much, especially the large ones, because I mean, they know in literal terms more than any human does in some sense. And, well, we have unlocked these reasoning capabilities on top of that knowledge, and I think that is actually what is enabling them to solve a lot of these problems.
But if you actually look at the way they approach problems, the reason what they do looks impressive to us is because we have so much less knowledge. And the model is approaching the problems in a fundamentally different way compared to a human would.
A human would have much more limited knowledge and they would usually have to be much more creative in solving problems because they have this lack of knowledge. While the model knows so much.
You'd ask it some obscure math question where you need like some specific theorem from 1850 or something. And then it would just like know that if it's like a large model.
So that makes the difficulty profile very different. And if you look at the way they approach problems, the reasoning models, they are usually not creative.
They are very effectively able to leverage the knowledge they have, which is extremely vast. And that makes them very effective in a bunch of ways.
But you might ask the question, has a reasoning model ever come up with a math concept that even seems like slightly interesting to a human mathematician? And I've never seen that. I mean, they've been around for all of six months.
But that's a long time. Lots of people have been, I mean, that's a long time.
But like, just think about this.
One mathematician might have been able to like do a bunch of work over that time. And they have produced orders of magnitude fewer tokens on math.
That's right. That's right.
Yeah. And then, and then like, I just want to emphasize it because like, just think about the sheer scale of knowledge that these models have.
Like it's enormous from a human point of view. So it is actually quite remarkable that there is no interesting recombination, no interesting, oh, this thing in this field looks kind of like this thing in this other field.
There's no innovation that comes out of that. And it doesn't have to be a big math concept.
It could be just a small thing that maybe you could add to a Sunday magazine on math that people used to have. But there isn't even like an example of that.
I think it's useful for us to explain like a very important framework for our thinking about what AI is good at and what AI is lagging in, which is this idea of kind of Moravec's paradox that things that seem very hard for humans, AI systems tend to make much faster progress on. Whereas things that look a bunch easier for us, kind of AI systems that totally struggle are often totally incapable of doing that thing.
And so, you know, this kind of abstract reasoning, you know, playing chess, playing Go, playing Jeopardy, doing kind of advanced math and solving math problems. There are even stronger examples like multiplying 100-digit numbers in your head, which is the one that calls solved first out of almost any other problem.
Or like following like very complex sort of symbolic logic arguments, like deductive arguments, which people actually struggle with that a lot. Like how do premises logically fall from conclusions? People have a very hard time with that, very easy for formal proof systems.
An insight that is related and is quite important here is that the tasks that humans seem to struggle on and AI systems seem to make much faster progress on are things that kind of emerged fairly recently in evolutionary time. So, you know, advanced language use emerged in humans maybe 100,000 years ago, and certainly playing chess and Go and so on are very recent innovations.
And so evolution has had much less time to optimize for them, in part because they're very new, but also in part because when they emerged, there was a lot less pressure because it conferred kind of small fitness gains to humans. And so evolution didn't optimize for these things very strongly.
And so it's not surprising that on these specific tasks that humans find very impressive when other humans are able to do it, that AI systems are able to make a lot of fast progress. In humans, these things are often very strongly correlated with other kind of competencies, like being a good, good at just, you know, achieving your goals or being a good coder is often very strongly correlated with solving kind of coding problems, or being a good engineer is often correlated with solving competitive coding problems.
But in AI systems, the correlation isn't quite as strong. And even within AI systems, it's the case that the strongest systems on competitive programming are not even the ones that are best at actually helping you code.
So like, you know, 03 mini high seems to be maybe the best at solving competitive code problems, but it isn't the best at actually helping you write code. It isn't getting most of the enterprise revenue from places like Hursar or whatever.
That's just Claude, right? Right. But an important insight here is that, you know, the things that we find very impressive when humans are able to do it, we should expect that AI systems are able to make a lot more progress on that.
But we shouldn't update too strongly about just their general competence or something because we should recognize that this is a very narrow subset of relevant tasks that humans do in order to be a competent, economically valuable agent. Yeah.
First of all, I actually just really appreciate that there is an AI organization out there where, because there's other people who take the compute perspective seriously, or try to think empirically about scaling laws and data and whatever. And it's striking how often that,
like taking that perspective seriously
leads people to just be like,
okay, 2027 AGI, which might be correct,
but it is like just interesting to get like,
no, we've also looked at the exact same arguments,
the same papers, the same numbers.
And like, we've come to a totally different conclusion.
On all these arguments,
I think this is all fascinating.
Okay, so I asked Dario this exact question two years ago when I interviewed him.
It went viral over Twitter.
Didn't he say AGI in two years?
But Dario's always had short timelines.
Okay, but we are two years later.
Did he say two years?
I think he actually did taste it.
Did he say three years?
So we have one more year.
One more year.
Better work hard.
But he's, I mean, I think he's like, he in particular has not been that well-colliberated. He's like, oh, like in 2018, he had like 20.
I remember talking to like a very senior person who's now at Anthropic. Yeah.
In 2017. And then he told various people that they shouldn't do a PhD because by the time they completed it, everyone would be automated.
Yeah, yeah. So anyways, I asked him this exact question, right? Because he has short timelines, which is that if a human knew the amount of things these models know, they would be finding all these different connections.
And in fact, I was asking Scott about this the other day when I interviewed him, Scott Alexander. And he said, look, humans also don't have this kind of logical omniscience.
I'm not saying we're omniscient, but we have examples of humans finding these kinds of connections. This is not an uncommon thing, right? I think his response to that was that these things are just not trained in order to find these kinds of connections.
But if you, like, their view is that it would not take that much extra compute in order to build some RL environment in which they're incentivized to find these connections. NextTokenPrediction just isn't incentivizing them to do this, but the RL required to do this would not be that, or set up some sort of scaffolds.
I think actually Google did do some similar, like, scaffold to make new discoveries, and I didn't look into, like, how impressive the new discovery was. They claimed that some new discovery was made by an LLM as a result.
On the Moravax paradox thing, this is actually a super interesting way to think about AI progress. But I would also say there that if you compare animals to humans, long-term intelligent planning, like an animal is not going to help you book a flight either.
An animal is not going to like help you book a flight either an animal or like an animal is not going to like do remote work for you or even do the kinds of things I think like what separates humans from other animals is that we can hold long term we can like come up with a plan and execute on it whereas other animals often had to go by instinct or within the kinds of environments that they have evolutionary knowledge of rather than like I'm put in the middle of the savanna or I'm put in the middle of the desert or I'm put in the middle of the tundra and I'll learn how to make use of the tools and whatever there. Like I actually think there's like a huge discontinuity between humans and animals and their ability to survive in different environments just based on their knowledge.
And so it's like a recently optimized thing as well. And then I'd be like, okay, well, we got it soon.
AIs will optimize it for fast. Right.
So I would say if you're comparing animals to humans, it's kind of a different thing. I think animals, like if you could put the competences that the animals have into AI systems, that might just already get you to like AGI, like already.
I think the reason why there is such a big discontinuity between animals and humans is because animals have to rely entirely on natural world data, basically, to train themselves. Like imagine that the only thing as a human that you saw was like nobody talked to you, you didn't read anything, you just had to learn by experience, maybe to some extent by imitating other people, but you have no explicit communication.
Well, it would be very inefficient. What's actually happening is that you have this, I think some other people have made this point as well, is that evolution is sort of this outer optimizer that's improving the software efficiency to the brain in a bunch of ways.
There's some genetic knowledge that you inherit, not that much because there isn't that much space in the genome. And then you have this lifetime learning, which is you don't actually see that much data during lifetime learning.
A lot of this is redundant and so on. So what seems to have changed with humans compared to other animals is that humans became able to have culture.
And they have language, which enables them to like have like a much more efficient training data modality compared to animals. They also have, I think, stronger ways in which they like tend to imitate other humans and learn from their skills.
So that also enables this knowledge to be passed on. I think animals are pretty bad at that compared to humans.
So basically, as a human, you're just being trained on much more efficient data. And that creates further insights to be then efficient at learning from it.
And then that creates this feedback where the selection pressure gets much more intense. So I think that's roughly what happened with humans.
But a lot of the capabilities that you need to be
like a good worker
in the human economy,
animals already have.
So they are able to,
like they have quite
sophisticated sensory motor skills.
I think they are actually able to do,
like animals are actually
able to pursue long-term goals.
But ones that they have been
instilled by evolution, like I think like a lion will a gazelle, and that is a complicated thing to do and requires stalking and blah, blah, blah. But when you say it's been instilled by evolution, like, there isn't that much information in the genome.
But it's just, like, I think if you put the lion in the Sahara and you're, like, go find lizards instead. Okay, so suppose you're pretty human, and they haven't seen the relevant training data.
I think they'd like they'd do slightly better. Slightly better, but not that much better.
I think a lot of the... Again, didn't you recently have an interview? Joseph Henrik.
Yeah. He would probably tell you that.
I think what you're making is actually a very interesting and subtle point that has an interesting implication. So often people point to, they say that ASI will be this huge discontinuity because, well, we have this huge discontinuity in the animal to human transition where like something, it's like not that much change between pre-human primates and humans genetically, but it resulted in this humongous change in capabilities.
And so they say, well, why not expect something similar between human-level intelligence and superhuman intelligence? And the point you're making is that, or at least one implication of the point you're making, is that actually it wasn't that we just gained this incredible intelligence. Because of biological constraints, animals have just been held back in this really weird way that no AI system has been arbitrarily held back of not being able to communicate with other copies or with other knowledge sources.
And so since AIs are not held back artificially in this way, there's not going to be a point where we should take away that hobbling.
I mean, you know, and then now they're like, now they explode.
Now, actually, I think I would disagree with that.
The implication that I made, I would actually disagree with.
I'm like a sort of like unsteerable chain of thought.
But because as we wrote a blog post together about AI corporations where we discussed, actually, there will be a similar unhobbling with future AIs, which is not about the intelligence, but a similar level of bandwidth and communication and collaboration with other AIs, which is a similar magnitude of change from non-human animals to humans in terms of their social collaboration that AIs will have with each other because of their ability to copy all their knowledge exactly, to merge, to distill themselves, to scale. Maybe before we talk about that, I think just like a very important point to make here, which I think underlies some of this disagreement that we have with others about both this argument from the transition from kind of non-human animals to humans is this like focus on intelligence and reasoning and R&D, which is enabled by that intelligence as being just enormously important.
And so if you think that you get this very important difference from this transition from primates, non-human primates to humans, then you think that in some sense you get this enormously important unlock from fairly small scaling and, say, brain size or something. And so then you might think, well, yeah, I could be, if we scale beyond the size of training runs that, you know, the amount of training compute that the human brain uses, which is maybe on the order of, you know, 1A24 flop or whatever, which we've recently surpassed, then maybe surpassing it just a little bit more enables us to unlock very sophisticated intelligence in the same way that humans have much more sophisticated intelligence compared to non-human primates.
And I think part of our disagreement is that intelligence is kind of important, but just having a lot more intelligence and reasoning and good reasoning isn't something that will kind of accelerate technological change and economic growth very substantially. Like it isn't the case that the world today is just like totally bottlenecked by not having, you know, not having enough good reasoning.
And that's not really what's bottlenecking the world's ability to grow much more substantially. I think that we might have some disagreement about this particular argument, but I think what's also really important is just that we have a different view as to how this acceleration happens.
That it's not just having like a bunch of really good reasoners that give you this technology that then accelerates things very drastically. Because that alone is not sufficient.
You need kind of complementary innovations in other industries. You need the economy as a whole growing and supporting the development of these various technologies.
You need the various supply chains to be upgraded. You might need demand for the various products that are being built.
And so we have this view where actually this very broad upgrading of your technology and your economy is important rather than just having very good reasoners and very good reasoning tokens that gives us this acceleration.
All right.
So this brings us back to the intelligence explosion. Here is the argument for the intelligence explosion.
Look, you're right that certain kinds of things might take longer to come about. But this core loop of software R&D that's required, if you just look at what kinds of progress is needed to make a more general intelligence, you might be right that it needs more experimental compute.
But we're just getting, as you guys have documented, we're just getting a shit ton more compute every single year for the next few years. So you can imagine an intelligence switch for the next few where in 2027, there'll be like 10x more compute than there is now for AI.
And you'll have this effect where the AIs that are doing software R&D are finding ways to make running copies of them more efficient, which has two effects. One, you're increasing the population of AIs who are doing this research.
So more of that in parallel can find these different optimizations. And a subtle point that they'd often make here is software R&D in AI is not just ALEA type coming up with new transformer-like architectures.
To your point, it actually is a lot of, like, you've got to, like, I mean, I'm not an AI researcher, but I assume there's, like, from the lowest-level libraries to the kernels to making RL environments to finding the best optimizer to, there's just, like, so much to do. And in, like, parallel, you can be doing all these things or finding optimizations across them.
And so you have two effects going back to this. One is if you look at the original GPT-4 compared to the current GPT-4-0 I think it's like how much cheaper is it to run? Maybe 100 times for the same capability or something.
So they're finding ways in which to run more copies of them at like, you know, 100x cheaper or something, which means that the population of them is increasing and the higher population is then helping you find more efficiencies. Not only does that mean you have more researchers, but to the extent that what's the complementary input is experimental compute, it's not the compute itself, it's the experiments.
And the more efficient it is to run a copy or to develop a copy, the more parallel experiments you can run. Because now you can do a GPT-4 scale training run for much cheaper than you could do it in 2024 or 2023.
And so for that reason also, this like software-only singularity sees more researcher copies who can run experiments for cheaper, dot, dot, dot. They initially are maybe handicapped in certain ways that you mentioned.
But through this process, they are rapidly becoming much more capable. What is wrong with this logic? I think the logic seems fine.
I think this is a decent way to think about this problem. But I think that it's useful to draw on a bunch of work that, say, economists have done for studying the returns to R&D and what happens if you 10x your inputs, the number of researchers, what happens to innovation or the rate of innovation.
and um and there are you know they they point out these kind of two effects where
you know as you do more innovation then you get to kind of stand on top of the shoulders of giants
and you get the benefit from past discoveries. And that makes you as a scientist more productive.
But then there's also kind of diminishing returns that the low-hanging fruit has been picked and it becomes harder to make progress. And overall, you can summarize those estimates as thinking about the kind of returns to research effort.
And, you know, we've looked into the returns to research effort in software specifically. And we look at a bunch of domains in traditional software or, you know, like linear integer solvers or SAT solvers, but also in AI, like computer vision and RL and language modeling.
and there, like, if this model is true,
that all you need is just cognitive effort. It seems like the estimates are a bit ambiguous about whether this results in this acceleration or whether it results in just merely exponential growth.
And then you might also think about, well, it isn't just your research effort that you have to scale up to make these innovations because you might have complementary input. So as you mentioned, experiments are the thing that might kind of bottleneck you.
And I think there's a lot of evidence that, in fact, these experiments and scaling up hardware is just very important for getting progress in the algorithms and the architecture and so on. So in AI, this is true for software in general, where if you look at progress in software, it often matches very closely the rate of progress we see in hardware.
So for traditional software, we see about a 30% roughly increase per year, which kind of basically matches more so. And in AI, we've seen the same until you get to the deep learning era.
And then you get this acceleration, which in fact coincides with the acceleration we see in compute scaling, which gives you a hint that actually the compute scaling might have been very important. Other pieces of evidence, you know, besides this coincidental rate of progress, Other kind of pieces of evidence are, you know, the fact that innovation and algorithms and architectures are often concentrated in GPU-rich labs and not in the GPU-poor parts of the world, like academia or maybe smaller research institutes.
That also suggests that having a lot of hardware is very important. If you look at specific innovations that seem very important, the big innovations over the past five years, many of them have some kind of scaling or hardware-related motivation.
So, you know, you might look at the transformer itself was about how to harness more parallel compute. Things like flash attention was literally about how to implement the attention mechanism more efficiently, or things like the Chinchilla scaling law.
And so many of these big innovations were just about how to harness your compute more effectively. That also tells you that actually the scaling of compute might be very important.
And I think there's just like many pieces of evidence that points towards this complementarity picture. So I would say that not only like even if you assume that experiments are not particularly important, the evidence we have both from estimates of AI and other software, although the data is a bit is not great, suggests that, you know, maybe you don't get this kind of hyperbolic, faster than exponential, you know, super growth in the overall algorithmic efficiency of systems.
I'm not sure I buy the argument that because these two things, compute and EA progress, have risen so concomitantly that this is a sort of causal relationship. So broadly, the industry as a whole has been getting more compute and as a result, making more progress.
But if you look at the top players, there's been multiple examples of a company with much less compute, but a more coherent vision, more concentrated research effort, being able to beat an incumbent who has much more compute. So OpenAI initially beating Google DeepMind.
And if you remember, there was these emails that were released between Elon and Sam and so forth where like we got to start this company because they've got this bottleneck on the compute and like look how much more compute Google DeepMind has. And then OpenAI made a lot of progress.
Similarly now with OpenAI versus Anthropic and so forth.
And then I think just generally, your argument is just like too outside view.
When we just do know a lot about like what is – like you're just like this very macroeconomic argument.
And I'm like, well, why don't we just ask the AI researchers? I mean AI researchers will often kind of overstate the extent to which just cognitive effort and doing research is important for driving these innovations, because that's often kind of convenient or useful. They will say the insight was, you know, was derived from some kind of nice idea about statistical mechanics or some nice equation in physics that says that we should do it this way.
And then, and then, but often that's kind of an ad hoc story that they tell to make it a bit more compelling to, uh, to, to the kind of reviewers. So Daniel mentioned this like, um, survey he did or where he, Daniel Kucatalo, um, he asked a bunch of AI researchers if had 1 30th the amount of compute, and he did 1 30th because AI is supposed to think 30 times faster.
If you had 1 30th the amount of compute, how much would your progress slow down? And they say, I make a third of the amount of progress I normally do. So that's just a pretty good substitution effect of, if you get 1 10th the compute, your progress only goes down down one third.
I was talking to an AI researcher the other day who's like just one of these like cracked people, gets paid millions and tens of millions of dollars a year probably. And we asked him, how much do these AI models help you in domains you already are, you know, how much does the AI models help you in AI research? And he said in domains that I'm already quite familiar with where I just closer to autocomplete it's like saves me four to eight hours a week um and then he said but in domains where I'm actually less familiar where it's like I need to draw new connections I need to understand how these different parts relate to each other and so forth um it saves me close to 24 to 36 hours a week, right? So that, and then that's like current models.
And I'm just like, he didn't get more computed, but it still saved him like a shit ton more time. Like it just like draw that forward.
It's like, that's a crazy implication or crazy trend, right? I mean, I guess, have we seen, like, I'm skeptical of the claims that we have actually seen that much of an acceleration in the process of R&D. Like, these claims seem to me like they're not borne out by the actual data I'm seeing.
So I'm not sure how much to trust them. I mean, on the general intuition that cognitive effort alone can give you a lot of AI progress.
Right. We've had, it seems like a big important thing the labs do is this like science of deep learning.
Like scaling laws is just, I mean, like it ultimately knitted out an experiment, but the experiment was motivated by cognitive effort. So for what it's worth, when you say that A and B are complementary, you're not saying like just as you can't get a lot of progress, like just as A can bottom IQ, B can also bottom IQ.
IQ. So when you say you need a computer and experiments and data, but you also need cognitive effort, that doesn't mean the lab who has the most compute is going to win.
That's a very simple point. Either one can be the bottom line.
If you just have a really dysfunctional culture and you don't actually prioritize using your computer very well and you just waste it, Well, then you're not going to make a lot of progress, right?
So it doesn't contradict the picture that someone with a much better vision, a much better team, much better prioritization can make better use of their compute if someone else was just bottlenecked heavily on that part of the equation. The question here is, once you get these automated AI researchers and you start this software singularity, your efficiency, software efficiency is going to improve by many orders of magnitude, while your compute stock, at least in sort of the short run, is going to remain fairly fixed.
So how many ohms of improvement can you get before you become bottlenecked by the second priority equation? Right. And once you actually factor that in, like how much progress should you expect? That's the kind of question.
I think people don't have, I think it's hard for people to have good intuitions about this because people usually don't run the experiment. So you don't get to see at a company level or at an industry level, what would have happened if the entire industry had 30 times less compute? Maybe as an individual, what would happen if you had 30 times less compute? You might have a better idea about that, but that's a very local experiment, and you might be benefiting a lot from spillovers, from other people who actually have more compute.
So because this experiment was never run, it's sort of hard to get direct evidence about the strength of complementarity what is your probability of if we live in the world where we get AGI in 2027 that there is a software only singularity quite high because then you're conditioning on compute not being very large so it must be that you get a bunch of software progress.
You just have a bunch of leverage from algorithmic progress in that world.
That's right.
I was thinking these are independent questions.
I think a call out that I want
to make is, I know that some
labs do have multiple pre-training teams
and they give people different amounts of
resources for doing the training
and different amounts of cognitive
effort, different size of teams.
But none of that, I think, has been published. And I'd love to see the results of some of those experiments.
I mean, I think even that won't update you very strongly just because it is often just very inefficient to do this very imbalanced scaling of your factor inputs. And in order to really get an estimate of how strong these complementarities are, you need to observe these very imbalanced scale-ups.
And so that rarely happens. And so I think the data that bears on this is just really quite poor.
And then the intuitions that people have also don't seem clearly relevant to the thing that matters about what happens if you do this very imbalanced scaling and where does this net out?
One question I have that it would be really interesting if somebody can provide an example of is maybe through history there was some point at which because of a war or some other kind of supply shock, you had to ramp up production or ramp up some key output that people really cared about. While for some weird like historical reason, many of the key inputs were not accessible to a ramp up, but you could ramp up one key input.
I'm talking very abstract terms. What do you see what I'm saying, right to make more like bombers but like you ran out of aluminum and you just like need to figure out something else to do um and how successful these efforts have been or whether you just keep getting bottlenecked well for for i think that is not quite the right way to do it because i think like if you're talking about materials then i think there's a lot of sense in which different materials can be substitutable for one another in different ways.
Like you can use aluminum.
I mean, aluminum is a great metal for making aircraft because it's sort of light and durable and so on. But you can imagine that you make aircraft with like worse metals and then it just takes more fuel and it's like less efficient to fly.
So there's a sense in which you can compensate and just cost more. Like I think it's much harder if you're talking about something like complementarity between labor and capital, complementary between like remote work and in-person work or like skilled or unskilled work.
And like there are input pairs for which I would expect it to be much more difficult. Or like, for example, you're looking at the complementarity between like the quality of leadership of an army and its number of soldiers, right? I mean, there is some effect there, but like if you just scale up, you just have excellent like leadership, but your army only has 100 people.
You're not going to get like very far. King Leonidas and Thermopylae? Well, they lost, right? It would be funny if we're building models of software and singularity and we're like, what exactly happened in Thermopylae? It's somehow relevant.
I mean, I can actually talk about that, but we probably shouldn't. Okay, sure.
By the way, so the audience should know, my most popular guest by far is Sarah Payne. Not only is she my most popular guest she's my most popular four guests because like all four of those episodes that I've done right there are like from a viewer minute adjusted basis I host a Sarah Payne podcast where I occasionally talk about AI um and uh anyways we did this three-part lecture series um where we're about, like, one of them was about India-Pakistan wars through history.
One of them was about, was it the Japanese culture before World War II?
The third one was about the Chinese Civil War.
And for all of them, my tutor, my history tutor was Ege.
And it just, like, why does he know so much about like fucking random, like 20th century conflicts? But he did. And he suggested a bunch of the good questions I asked her.
We'll get into that. Actually, what's going on there? I don't know.
I mean, I don't really have a good question. I think it's interesting.
I mean, I read a bunch of stuff, but it's a kind of boring answer. Like, I know.
Imagine, like, a top AI researcher, like, what's going on? Like, how are you so good? And then they will probably give you, like, a boring answer. Like, I don't know.
Like, I did this. I'm anxious.
That itself is interesting, that often these kinds of questions elicit boring answers. Yeah.
Like, it tells you about the nature of the skill. Right.
How did you find them? We connected on, like, some, on a Discord for Metaculous, which is this forecasting platform. And I was a graduate student at Cambridge at the time doing research in economics.
And I was having conversations with my peers there. And I was occasionally having conversations with Ege.
And I was like, this guy knows a lot more about economics. And he's, at the time, he was a computer science undergrad in Ankara.
And he knows more about economics and about, you know, these big trends in economic growth and economic history than almost any of my peers at university. And so, like, what the up with that? You know, so we started like having frequent collaborations and ended up hiring for EPOC because it clearly makes sense for him to work on these types of questions.
And it seems like at EPOC, you just collected this like a group of internet misfits and weirdos. Yeah, that's right.
How did you, so how did you start epoch and then how did you accomplish this yeah so i was um i was at mit doing doing more research and i was pretty unhappy with the bureaucracy there where it was very hard for me to scale projects up hire people um and i was pretty excited about a bunch of work that my pi wasn't excited about because it's maybe hard to publish or it doesn't confer the same prestige. And so, you know, I was chatting with Jaime Sevilla, one of the co-founders, and we just collaborated on projects and then thought we should just start our own org because we can just hire people and work on the projects we were excited about.
And then I just, you know, hired a bunch of the insightful misfits that like... But did you like, was the thesis like, oh, there's a bunch of underutilized internet misfits and therefore like this org was successful or you started the org and then you were like...
I think it's more of the latter. So it was more like we can make a bunch of progress because clearly like academia and industry is kind of dropping the ball on a bunch of important questions that academia is unable to publish interesting papers on.
Industry is not really focused on producing useful insights. And so it seemed like very good for us to just do that.
And also the timing was very good. So we started just before, you know, chat GPT and we wanted to have much more grounded discussions of the future of AI.
Yeah. And I was frustrated with the quality of discussion that was happening on the internet about the future of AI.
And I mean, to some extent or to a very large extent, I still am. And that's like a large part of what, you know, motivates me to do this.
It's just like born out of frustration with bad thinking and arguments about where AI is going to go. The part about my job that I enjoy the least is the post-production.
I have to rewatch the episode multiple times, make all these difficult judgment calls. And I've been trying to automate all this work with LLM scripts.
And I found that Google's Gemini 2.5 Pro is the best model I've tried for these tools. So much of the post-production requires understanding the delivery, the context, all these other things that you don't get from a text-only transcript.
Unlike other models I've tested, I can actually just shove in the four-hour raw audio file into Gemini because of its multimodal capabilities. And it can generate super high-quality transcripts, identify great snippets for clips, a bunch more other things.
I've actually made a repo with all these tools, and I've linked to the GitHub in the description below in case you might find it helpful. I actually use 2.5 Pro in order to write the code for these scripts.
It's actually quite interesting to read its reasoning traces as it's thinking through your gnarly list of requests and tasks. Gemini 2.5 Pro is now available in preview with higher rate limits.
You can try it out at aistudio.google.com. Thanks to Google for sponsoring this episode.
And now back to Ege and Tame. Okay, so let me ask you about this.
I can poke you from the... So just to set the scene for the audience, we're going to talk about the possibility of this explosive economic growth and like greater than 30% economic growth rates.
So I want to poke you both from the perspective of maybe suggesting that this isn't aggressive enough in the right kind of way because maybe it's too broad. And then I'll poke you from the perspective of the more normal perspective that, hey, this is fucking crazy.
I imagine it would be difficult for you to do the second thing. No, I mean, I think it might be fucking crazy.
Let's see. The big question I have about this broad automation,
like I get what you're saying about the industrial revolution,
but in this case,
we can just make this like argument that you get,
you get this intelligence and then what,
what you do next is you go like to the desert and you build this like Shenzhen
of robot factories,
which are building more robot factories, which are building if you need to do experiments and you build bio labs and you build chemistry labs and whatever other labs. If you build Shenzhen in the desert, I agree.
That looks much more plausible than a software-only singularity. But why, the way you're framing it, it sounds like McDonald's and Home Depot and fucking whatever are growing at 30% a year as well.
And not just, like, is it, you know, the aliens level view of the economy? Is it that, like, there's a robot economy in the desert that's growing at 10,000% a year and everything else is the same old, same old? Or is it like, you know what I mean? No, I mean, there is a question about what would be possible or physically possible and what would be the thing that would actually be efficient, right? Yeah. So it might be the case.
And again, once you're scaling up the hardware part of the equation as well as the software part, then I think the case for this feedback loop gets a lot stronger. If you scale up data collection as well, I think it gets even stronger, like real-world data collection by deployment and so on.
But building Shenzhen in a desert, that's a pretty, like, if you think about the pipeline, so far we have relied, first of all, we're relying on the entire semiconductor supply chain. That industry depends on tons of inputs and materials and whatever.
It gets from probably tons of random places in the world and creating that infrastructure,
like doubling or tripling, whatever, that infrastructure,
like the entire thing, that's very hard work, right?
So probably you couldn't even do it even if you just have Shenzhen and Eleazar.
Like that would be even more expensive than that.
And on top of that, so far we have been drawing heavily
on the fact that we have built up this huge stock of data over the past 30 years or something on the internet.
Like imagine you were trying to train a state-of-the-art model, but you only have like 100 billion tokens, right, to train on.
That would be very difficult.
So in a certain sense, our entire economy has produced this huge amount of data on the internet that we are now using to train the models. It's plausible that in the future, when you need to get new competencies added to these systems, the most efficient way to do that will be to try to leverage similar kind of modalities of data, which will also require this.
You would want to deploy the systems broadly because that's going to give you more data. Maybe you can get where you want to be without that, but it would just be less efficient if you're signing from scratch compared to if you're collecting a lot of data.
I think this is actually a motivation for why labs want their LLMs to be deployed widely. Because sometimes when you talk to ChatGPT, it's going to give you two responses and it's going to say, well, which one was good? Or it's going to give you one response and it's going to ask you, was this good or not? Well, why are they doing that? That's a way in which they are getting user data through this extremely broad deployment.
So I think you should just imagine that thing to continue to be efficient and continue and continue to increase in the future, because it just makes sense. And then there's a separate question of, well, suppose you didn't do any of that.
Like, suppose you just tried to imagine the most rudimentary, the most narrowest possible kind of infrastructure build out and deployment that would be sufficient to get this positive feedback loop that leads to much more efficient AIs. I agree that loop could, in principle, be much smaller than the entire world.
I think it probably couldn't be as small as Transcendental Desert, but it could be much smaller than the entire world. But then there's a separate question of, would you actually do that? Would that be efficient? I think some people have the intuition that there are just these extremely strong constraints, maybe regulatory constraints, maybe social political constraints, to doing this broad deployment.
They just think it's going to be very hard. So I think that's part of the reason why they imagine these more narrow scenarios where they think it's going to be easier.
But I think that's overstated. I think people's intuitions for how hard this kind of deployment is comes from cases where the deployment of the technology wouldn't be that valuable.
So it might come from housing. We have a lot of regulations on housing.
Maybe it comes from nuclear power. Maybe it comes from supersonic flights.
Those are all technologies that would be useful if they were maybe less regulated, but they wouldn't double economic output. I think the core point here is just the value of AI automation and deployment is just extremely large, even just for workers, at least the ones know, finding, you know, there might be some kind of displacement and there might be some transition that you need to do in order to find a job that works for you.
But otherwise, the wages could still be very high for a while at least.
And on top of that, the gains from owning capital might be very enormous.
And in fact, a large share of the
US population would benefit from housing. They own housing, they have 401Ks.
Those would do enormously better when you have this process of broad automation and AI deployment. And so I think think there's there could just be a very deep support for some of this, even when it's like
totally changing the nature of labor markets and the skills and occupations that are in demand. So I would just say it's complicated.
I think what the political reaction to it will be when this starts actually happening.
I think the easy thing to say is that, yeah, this will become a big issue,
and then it will be when this starts actually happening. I think like the easy thing to say is that, yeah, like this will become like a big issue and then it will be maybe controversial or something.
But like, what is the actual nature of the reaction in different countries? I think that's kind of hard to forecast. Like I think the default view is like, well, people are going to become unemployed.
So it will just be very unpopular. I think that's like very far from obvious.
Yep. And I just expect heterogeneity and how different countries respond, and some of them are going to be more liberal about this and going to have a lot broader deployment, and those countries probably end up doing better.
Just like during industrial revolution, some countries were just ahead of others. I mean, eventually, almost the entire world adopted the sort of norms and culture and values of the industrial revolution in various ways.
And actually, you say they might be more liberal about it, but they might actually like be less liberal. They might be less liberal in many ways.
And in fact, that might be like more functional in this world in which you have broad AI deployment. We might adopt the kind of values and norms that get developed in, say, you know, the UAE or something, which is like maybe focused a lot more on making an environment that is very conducive for AI deployment.
And we might start, you know, emulating and adopting various norms like that. And they might not be kind of classical liberal norms, but norms that are just more conducive to AI being functional and producing a lot of value.
This is not meant to be like a strong prediction. This is just an illustrative case.
It might just be that like the freedom to deploy AI in the economy and build out lots of physical things at scale. Maybe that ends up being more important in the future.
Maybe that is still missing something. Maybe there's some other things that are also important.
But like I would just like the generic prediction that you should expect variants and some countries do better than others. I think that's much easier to predict than like the specific countries that end up doing better.
Yeah, or the norms that that country will. That's right.
So, I mean, one thing I'm confused about is if you look at the world of today versus the world of 1750, the big difference is just like, we've got crazy tech that they didn't have back then. We've got these cameras, we've got these screens, and we've got rockets and so forth.
And that just seems like the result of technological growth and R&D and so forth. It's a capsule accumulation.
Well, explain that to me you're just talking about this infrastructure build-out and blah, blah, blah. But why won't they just fucking invent the kinds of shit that humans would have invented by 2020, 2020, 2050? Producing this stuff takes a lot of infrastructure build-out.
But that infrastructure is built out once you make the technology, right? I don't think that's right. There isn't this temporal difference where it's first you do the invention and then often there's this interplay between the actual capital buildup and the innovation.
Learning curves are about this, right, fundamentally. What has driven the increase in the efficiency of solar panels over the past 20, 30 years? It isn't just like people had the idea of 2025 solar panels.
Nobody 20 years ago had the sketch for the 2025 solar panel. It's this kind of interplay between having ideas, building, learning, producing, and...
Other complementary inputs also becoming more efficient at the same time. Like, you might get better materials.
Like, for example, the fact that aluminum becomes something that's... Like, for example, the fact that smelting processes got a lot better towards the end of the 19th century, so it became a lot easier to work with metal.
Maybe that was a crucial reason why aircraft technology later became more popular. So it's not like someone came up with the idea of, oh, you can just use something that just has wings and has a lot of thrust, and then that might be able to fly.
That basic idea is not that difficult. But then, well, how do you make it actually a viable thing? That's right.
Well, that's much more difficult. Have you seen the meme where two beavers are talking to each other and they're looking at the Hoover Dam? And one of them is like, well, I didn't build that, but it's based on an idea of mine.
That's right. The point you're making is that this invention-focused look on tech history the underplays, the work that goes into making specific innovations practicable and to deploy them widely.
It's just hard, I think. Like, it's just hard to even, like, suppose you want to write a history of this, right? Like, you want to write a history of how was the, like, light bulb developed or something.
It's just really hard because to understand why specific things happen at specific times, you probably need to understand so much about the economic conditions of the time. Like, for example, Edison spent a ton of time experimenting with different filaments to be used in the light bulb.
The basic idea is very simple. You make something hot and it glows.
But then like what actually, what filament actually works well for that in a product? What is like durable? What like has the sort of highest ratio of like light output versus like heat and like so that you have less waste and it's more efficient. And then even after you have the product, then your face is a problem.
Well, I mean, it's like 1880 or something, and then US homes don't have electricity, so then nobody can use it. So now you have to build power plants and build power lines to the houses so that people have electricity in their homes so that they can actually use this new light bulb that you created.
So he did that. But then people present it as if it's like, okay, he just came up with the idea, like it's a light bulb.
I guess the thing people would say is like you're right about how technology would progress if we were humans deploying for the human world. But what you're not counting is there's just going to be this like AI economy where maybe they need to do this kind of innovation and learning by doing when they're figuring out how to – I want to make more robots because they're helpful and so like we got we're going to build more robot factories we'll learn and then we'll make better robots or whatever but like that is just like a geographically that is a small part of the world that's happening in or you know you're saying like it's not like and then that like then they walk in your building and then you do a business transaction with Lunar Society Podcast LLC.
And then, you know what I mean? Yeah, I mean, for what it's worth, like if you look at the total surface area of the world, it might well be the case that the place that initially experiences this very fast growth is like a small percentage of the surface area of the world. Because I think that was the same for an industrial revolution.
It was not different. Yeah.
So, but then like, I'm just like, what concretely does this explosive growth look like? If I look at this heat map of growth rates on the globe, is there just going to be like one area is like blinding hot and that's, you know, that's like the desert factories with all of these experiments and like. Yeah.
So I would say our idea is that it's going to be broader than that, but probably initially. So eventually it would be probably most of the world.
But as I said, because of this heterogeneity, because I think some countries are going to be faster in adoption than others. Maybe some cities will be more faster in adoption than others.
And that will mean that there is differentials. And some countries might have much faster growth than other countries.
But I would expect that at a jurisdiction level, it would be more homogenous. So, for example, I expect the primary obstacles to come from things like regulation.
And so I would just imagine it's being more delineated by regulatory jurisdiction boundaries than anything else. Got it.
So you may be right that this infrastructure build-out and capital deepening and whatever is necessary for a technology to become practical. Or even to be discovered.
Like there's an aspect of it that where you discover certain things by scaling up, learning by doing. Yeah.
curve. And there's this separate aspect where you get to, like, suppose that you become wealthier.
Well, you can invest that increased wealth in, like, yeah, you use it to accumulate more capital, but you also can invest it in R&D and other ways. You get Einstein out of the patent office.
Like, you need some amount of resources for that to make make sense. You need the economy to be of a certain scale.
You also need demand for the product you're building. You could have the idea, but if the economy is just too small, there isn't enough demand for you to be specializing and producing the semiconductor or whatever.
Because there isn't enough demand for it, then it doesn't make sense. So you So you want the economy, like a much larger scale of an economy is useful in very many ways.
In delivering complementary innovations and discoveries happening through serendipity, producing, like having there be consumers that would actually pay enough for you to recover your fixed costs of doing all the experimentation and the invention. You need the supply chains to exist to deliver the germanium crystals that you need to do, grow in order to come up with the semiconductor.
You need a large labor force to be able to help you do all the experiments and so on. I think the point you're illustrating is like, look, could you have just figured out
that there was a Big Bang by first principles of reasoning?
Maybe.
But what actually happened is we had World War II
and we discovered radio communications
in order to fight and effectively communicate during the war.
And then that technology helped us build radio telescopes
and then we discovered cosmic microwave background
and then we had to come up with an explanation for cosmic microwave.
And then we discovered like the Big Bang
as a result of like World War II. People under-emphasize that giant effort that goes into this kind of buildup of all the relevant capital and all the relevant supply chains and the technology.
I mean, earlier, you were making a similar comment when you were saying, oh, you know, reasoning models, actually, in hindsight, they look pretty simple, but then you're kind of ignoring this giant kind of upgrading of the technology stack that happened, you know, that took five to 10 years prior to that. And so I think people just under emphasize the support that is had from the overall upgrading of your technology, of the supply chains, of various sectors that are important for that.
And people focus on just specific individuals of like, you know, Einstein had this genius insight and like, you know, he was the kind of very pivotal thing in the causal chain that resulted in these discoveries or Newton was just extremely important for discovering calculus without thinking about, well, there was this kind of all these other factors that produced lenses, that produced telescopes, that got the right data and that made people ask questions about dynamics and so on that motivated some of these questions. And those are also extremely important for science, scientific and technological innovation.
Yeah. You know, conquest, what is it? One of conquest laws is like the more you understand about a topic, the more conservative you become about that topic.
And so there, there might be like a similar law here where like the more you understand about an industry, the more sort of like, I obviously I'm just like a commentator or whatever or a podcaster um but i i understand ai better than any other industry i understand and there i have the sense from talking to people like you that oh so much went into getting ai to the point where it is today um whereas when i talk to journalists about ai they're like okay who is the who is the crucial person we need to cover and they're like uh should we get in touch with with Jeffrey Hinton? Should we get in touch with Ilya? And I just have this, like, you're kind of missing the picture. But then you should have that same attitude towards things you – or maybe it's a more similar phenomenon as German amnesia.
We should have a similar attitude towards other industries that it's much more complicated. Right.
I mean, it's Robin Hanson as this abstraction of, like, seeing things in near mode versus far mode. Right.
And I think if you don't know a lot about the topic, then you see it sort of in far mode and you sort of simplify. That's right.
Things you know, you see a lot more detail. Like in general, I think the thing I would say and the reason I also believe that just like abstract reasoning and like sort of deductive reasoning or even Bayesian reasoning by itself is not like sufficient or like is not as powerful as many other people think is because I think there's just this
like enormous amount of like richness and detail in the real world that like you just
can't like reason about it.
Like you need to see it.
And obviously that like that is not an obstacle to AI being incredibly transformative because as I I said, you can scale your data collection. You can scale experiments you do both in the AI industry itself and just more broadly in the economy.
So, you just discover more things. More economic activity means you have more exposed surface area to have more discoveries.
Like, all of these are things that have happened in our past. Right.
So, there's no reason that they couldn't speed up. Like the fundamental thing is that there's no reason fundamentally why economic growth can't be much faster than it is today.
Like it's probably about as fast right now just because humans are such an important bottleneck. They both supply the labor.
They play crucial roles in the process of like discovery of various kinds of productivity growth. There's just strong complementarity to some extent with capital, that you can't substitute machines and so on for humans very well.
So the growth of the economy and growth of productivity just ends up being bottlenecked by the growth of human population. Publicly available data is running out.
So major AI labs like Meta, Google DeepMind, and OpenAI partner with Scale to push the boundaries of what's possible. Through Scale's data foundry, major labs get access to high-quality data to fuel post-training, including advanced reasoning capabilities.
As AI races forward, we must also strengthen human sovereignty. Scale's research team, SEAL, provides practical AI safety frameworks, evaluates frontier AI system safety via public leaderboards, and creates foundations for integrating advanced AI into society.
Most recently, in collaboration with the Center for AI Safety, Scale published Humanity's Last Exam, a groundbreaking new AI benchmark evaluating expert-level reasoning and knowledge capabilities across a wide range of fields.
If you're an AI researcher or engineer and you want to learn more about how Scales Data Foundry and Research Lab can help you go beyond the current frontier of capabilities, go to scale.com slash Dwarkesh. Let me ask a tangential question.
What has happened in China over the last 50 years, Would you describe that as, in principle, the same kind of explosive growth that you expect from AI? Because there's a lot of labor that makes the marginal product of capital really high, which allows you to have 10% plus economic growth rates. Is that basically in principle looking from AI? So I would say in some ways it's similar, in some ways it's not.
Probably the most important way in which it's not similar is that in China you see this relative, like you see a massive amount of capital accumulation, a substantial amount of adoption of new technologies and probably also human capital accumulation to some extent. But you're not seeing a huge scale-up in the labor force, the labor force.
while for AI you should expect seeing a huge scale up in the labor force, if I go labor force. While for AI, you should expect to see a scale up in the labor force as well.
Not in the human workforce, but in the AI workforce. I think you did kind of like, maybe not consecutive increases in the labor force increase, but like you did.
The key thing here is just the simultaneous scaling of both these things. And so like might ask the question of, isn't it basically half of what's going to happen with AI that you scale up capital accumulation in China? But actually, that's really not.
If you get both of these things to scale, that gives you just much faster growth and a very different picture. But at the same time, if you're just asking, like, what would 30% growth per year, like, look like? Like, in terms of, like, if you just want to have an intuition for how transformative that would be in concrete terms, then I think looking at China is not such a bad case.
Like, you can, especially in the 2000s or maybe late 90s. Like, that gives you a good, that's even slower than what we're forecasting.
Right. I think also looking at the Industrial Revolution is pretty good.
Well, the Industrial Revolution is very slow. But just in terms of the types of...
The kind of... The margins along which we made progress in terms of products.
So what didn't happen... The thing that didn't happen during the Industrial Revolution is we just produced a lot more of things that people were producing prior to the Industrial Revolution, like producing a lot more crops and maybe a lot more kind of pre-Industrial Revolution style houses or whatever on farms.
Instead what we got is along pretty much every main sector of the economy, we just had many different products that are totally different from what was being consumed prior to that. So in transportation, in food.
I mean, healthcare is a very big deal. Antibiotics.
So another question, because I'm not sure I understand how you're defining the learning by doing versus explicit R&D. Because there's the way for taxes that companies say what they call R&D.
But then there's like the intuitive understanding of R&D.
So if you think about how AI is boosting a TFP,
you could say that like right now,
if you just had replaced the TSMC process engineers with AIs,
and they're finding different ways
in which to improve that process
and like, you know, improve efficiencies, improve yield. Right.
I would kind of call that R&D. On the other hand, you emphasize this, the other part of TFV, which is, like, better management and… Learning by doing.
That kind of stuff. Learning by doing could be, you could… I mean, like, how much, like, you're going to get to, like, the fucking Dyson Spear by better management? But that's not the argument, right? The point is that there are all these different things.
Some of them are maybe more complementary than others. The point is not that you can get to a Dyson sphere by just scaling labor and capital.
That's not the point. You need to scale everything at once.
So just as you can't get to a Dyson sphere by just scaling labor and capital, you also can't get to it by just scaling TFP. That doesn't work.
I think there's a very important distinction between what is necessary to scale to get to this Dyson Sphere world and what is important. In some sense, producing food is necessary.
But of course, producing food doesn't get you to the Dyson sphere, right? So I think R&D is necessary, but on its own isn't sufficient. And scaling up the economy is also necessary.
On its own, it's not sufficient. And then you can ask the question, what is the relative importance of each? Yeah.
So I think our view here is like very much the same. We're like, it is very connected to our view about the software R&D thing, where we're just saying there are these bottlenecks, so you need to scale everything at once.
This is just a general view. But I think people misunderstand us sometimes as saying that R&D is not important.
No, that's not what we're saying. We're saying it is important.
It is less important in relative terms than some other things, none of which are by themselves sufficient to enable this growth. So the question is like, how do you do the credit attribution? I mean, one way in which in economics is standard to do that is to look at the elasticities of output to the different factors.
Like capital is less important than labor because the output elasticity of like labor elasticity output is like 0.6, while for capital is like 0.3. But neither are by themselves sufficient.
Like if you just scaled one of them and the other remained fixed, then neither would be sufficient to indefinitely scale output. One question that Daniel posed to me is like, because I made this perspective about everything being interconnected when you were talking about like, another example people often bring up is what would it take to build the iPhone in the year 1000?
And it's like unclear how you could actually do that without just like replicating every like intermediate technology or most intermediate technologies.
And then he made the point like, okay, fine, whatever.
Nanobots, like nanobots is not a crux here the crux at least to the thing he cares about which is human control is just by when can the robot economy whether or the AI economy whether it's a result of capital deepening or whether it's a result of R&D by when will will they have just like the robots? And they have more sort of like cumulative physical power. Right.
But he's imagining like a separate thing called the AI economy. Why would you imagine that? That seems like a...
I think it's probably downstream of his views about the software only singularity. But again, like those are views that we don't share.
It's just much more efficient for AI to operate in our economy and benefit from the existing supply chains and existing markets rather than set up shop on some island somewhere and do its own thing. Yeah, and then it's something clearer.
For example, people might have the intuition, I brought this up before, the distinction between what is the minimum possible amount of build-out that would be necessary to get this feedback loop up and running and what would be the most efficient way to do it, which are not the same question. But then people have this view that, oh, like the most efficient thing in principle, we can't do that because like… I think the example he might give is when the conquistadors arrived to the New World or when the East India Trading Company arrived to India, they did integrate into the existing economy.
In many cases, it depends on how you define integrate, but the Spanish relied heavily on New World labor in order to do silver mining and whatever. East India Trading Company was like, the ratio of British people to Indian people just like not that high, right? So they just had to rely on the existing labor force.
But they were still able to take over because of, I don't know what the analogous thing here is, but you see what I'm saying. And so he's concerned about by when will they, even if they're ordering components off of Alibaba or whatever, by what, and sorry, I'm being trite.
You see what I'm saying? Even if they're integrating those supply chains, by when are they in a position where because this part of the economy has been growing much faster, they could take over the government or … If they wanted to? That's right, yeah. Okay, so I think that is like eventually you expect the AI systems to be driving most of the economy.
And I don't think that's like, unless there are some very strange coincidences where like humans are able to like somehow uplift themselves and like able to become competitive with the AIs by like stopping being biological humans or whatever, which seems very unlikely early on, then AI is just going to be much more powerful. Okay.
So, and I agree that in that world, if the AI is just somehow coordinated and decide, okay, we should just like take over or something, like they just somehow coordinated to have that goal, then they could probably do it. Okay.
But, okay, that's also probably true in our world. Like in our world, if the, I don't know, if the U.S.
wanted to invade Central Island, then probably they could do it. I don't think anyone could stop them.
But like, what does that actually mean? I mean, there is this dramatic power imbalance, but that doesn't mean, like that doesn't tell you what's going to happen, right? Like, why doesn't the U.S. just invade Guatemala or something? Like, why don't they do that? Seems like they could easily do it.
Because the value to the U.S. of the land of, yeah.
But like, there's, so basically it just seems, I agree that might be true for AIs because like, most of the shit is in space and once you get get like you want to do the capital deepening on Mars and like the surface of the sun instead of New York City. So it's deeper than that.
Like there are also the there's also the fact that if the AIs are going to be integrated into our economy. So basically they start out as like a small part of our economy or our workforce, and over time, they grow, and over time, they become the vast majority of the actual sort of work power in the economy.
But they are growing in this existing framework where we have like norms and rules for better coordination, and then undermining those things has a cost. So if getting the things that is making the humans sort of wealthier than they used to be before and more comfortable, like, yeah, like you would probably be better off if you could just take that from them.
But the benefit to you, if you already are getting almost all of the income in the economy will be fairly small. I mean, I feel like the sentient nihilist thing is not the...
There's one reference class that includes that,
but historically,
there's like a huge reference class that includes
East India Trading Company
could have just kept trading with the Mughals.
They just like took over, right?
Like they could have kept trading
with the 50 different nation states
in pre-colonial India, but yeah.
That's right.
I mean, that's what they were initially doing.
And then whatever. Like I'm not going to...
But that is the reference class of like... I agree.
Yeah. I agree.
So like, if the question is, like, if they are entirely, if they have some totally different values and then they represent most of the economy, then like, would they take over? I still don't know because I'm not sure to what extent the class of all AI is like a natural like class. Like it's sort of like why don't the young people in the economy like I think it's a closer.
So I agree that sometimes these kinds of class arguments are misused. For example, when Marxists are like why don't like this like class uprise against the others.
Dana made the interesting argument that if you look at the history of the conquistadors, when Cortez was making his way through the New World, he had to actually go back and fight off a Spanish fleet that had been sent to arrest him. That's right.
And then go back. Right.
So you can have this fight within this like conquering AIs. And then that still nets out in the Native Americans getting disempowered.
But with AIs in particular, they're just like copies of each other. In many other ways, they just have a lot, they have lower transaction costs when they trade with each other or interact with each other.
There's other reasons to expect them to just be more compatible coordinating with each each other than coordinating with the human world. Sure, but then, like, I guess I'm still not seeing the, I mean, if the question is just that, is it possible for that to happen, which is like a weaker claim, then yeah, I mean, it seems possible.
But there are, I think, a lot of arguments just pushing by against it. Probably actually the biggest one is the fact that AI preferences are just not, like, just look at the AIs we have today.
Like, can you imagine them doing that? I think people just don't put a lot of weight on that because they think once we have enough optimization pressure and once they become super intelligent, they're just going to become misaligned. But I just don't see the evidence for that.
No, I mean, I think that's actually, like, I agree there's some evidence that they're, like, good boys. No, there's more than some evidence.
No, no, but there's also some evidence, like, there's a new open AI paper where, in Chain of Thought, like, reward hacking is such a strong basin that if you were like, hey, let's go solve this coding problem, in the Chain of Thought, they'll just be like, okay, let's hack this. And then like figure out how to hack it.
So imagine that you gave students at a school a test and then the answer key was like one. Right.
But like the reference class of humans does include like Cortez and the East Indian Trading Company. Sure.
So I think one issue here is that I think people are doing this very kind of partial equilibrium analysis or something where they're thinking about just this raw abilities of AI systems in a world where AI systems are kind of dominant and like human civilization has done very little in terms of integrating itself and the AI is integrating itself into the human world. Maybe making, you know, insofar as it's poor at communicating and coordinating with AIs, addressing those deficiencies and improving that, insofar as that's posing a risk or creating inefficiencies because it's unable to benefit from coordinating and trading, then it should have this enormous incentive to address that.
Insofar as there is a lot of value to be gained from dominating and taking over humans, like what you might get is a more negotiated settlement. If that's indeed the case, then a war would just be inefficient.
And so you would want to negotiate some settlement that results in some outcomes that are mutually beneficial. Compared to the counterfactual, not compared to...
There was a mutually beneficial trade that was made between the Qing dynasty and the British in the opium wars, right? But it was maybe better than pre-industrial China going to war with the British Empire, but it wasn't better than never having interacted with the British Empire in the first place. I think one mistake that I feel people make is they have this very naive analysis of what creates conflict.
I think Matthew has written a bit about this, a colleague of ours, where they say there's misalignment. And so that then creates conflict.
But that's actually not what the literature on what causes conflict says creates conflict. It's not just misalignment.
It's also other issues like not being able, like having bad understanding of the relative strengths of your armies versus theirs or maybe having these very uh strong commitments that you think some grounds are secret and so you're not willing to do any trade in order to give up some of that in order to gain something else and so then you have to posit some additional things other than just the base value misalignment part. I think you're making a good argument against like humans take up the spears and the machetes and go to war against the AI data centers.
Because maybe like that, yeah, there's not this asymmetric information that often leads to conflicts in history. But this argument does not address at all the risk of just takeover, which can be the result of a peaceful end negotiation where human society is just like, look, we're totally outmatched and we'll just take these meager concessions rather than going to war.
But insofar as it's more peaceful, then I think it's much less of a thing to worry about. I think Like I think there could be this, you know, trend where we indeed have this gradual process where, you know, AI is much more important in the world economy and actually deciding and determining what happens in the world.
But this could be beneficial for humans where, you know, we're getting access to this, you know, much vast, much, much larger economy and much more advanced technological stock. Yeah.
So I think it's important to be clear about what is the thing that you're actually worried about. Like, I think some people are just like say that, oh, like we're going to like humans are going to lose control of the future.
Like we're not going to be the ones that are making the important decision. We, however, conceive that social kind of ne nebulous.
But okay, so is that something to worry about? If you just think biological humans should remain in charge of all important decisions forever, then I agree. The development of AI seems like kind of a problem for that.
But in fact, other things also seem like kind of a problem for that. I just don't expect to generically be true.
Like in a million years from now, even if we don't develop AI, biological humans, the way we recognize them today, are still making all the important decisions and they have something like the culture that we would recognize from ourselves today. I'll be pretty surprised by that.
So I think there is a sense, I think Robin Hanson has again talked about this, where he said a bunch of the things that people fear about AI are just things they fear about change and fast change. So the thing that's different is that AI has a prospect of accelerating a bunch of this change so that it happens in a narrower period.
I think it's not just the kind of change that would have happened from, let's say, genetically modifying humans and blah, blah, blah. It's instead happening in a compressed amount of time.
I think the worry comes more from like it is a very it's not just that change compressed. It's a very different like vector of change.
Yeah. But what is the argument for that? I have never seen a good argument for this.
You should expect a bunch of change if you accelerate just human change as well. Like you might expect different values to become much more dominant.
You might expect people that don't discount the future as much to be much more influential because they save more and they make good investments. People who are higher risk tolerance.
Higher risk tolerance, like because they're more willing to make kind of bets that maximize expected value and so get much more influence.
So just generically accelerating human change would also result in a lot of things being lost that you might care about.
I think the argument is that maybe the speed of the change determines what fraction of the existing population or stakeholders or whatever have some causal influence on the future. And maybe the thing you care about is like, look, there's going to be change, but it's not just going to be like one guy presses a button.
That's like the software singularity extreme. Right.
And it's more like, you know, like over time norms change and so forth. So if you're looking at the software singularity picture, I agree that picture looks different.
And again, I'm coming back to this because obviously Daniel and maybe Scott to some extent, they probably have this view that the software only singularity is like more plausible. And then one person would just be in a position to like, we could end up in a situation where their idiosyncratic preferences or something end up more influential.
That's right. Yeah.
Even in, I agree that is, like that makes the situation look different from if you just like have this broader process of automation. But even in that world, like I think a lot of people have this view about things like value lock-in, where like they think this moment is like a pivotal moment in history, and then we're just going to have, someone is going to get this AI, which is very powerful because, say, of its own singularity, and then they're just going to lock in some values, and then those values are just going to be stable for millions of years.
And I think that just looks very unlike anything that has happened in the past, So I'm kind of confused why people think it's very plausible. I think people have the argument that they see the future, again, in my view, in sort of far mode.
They think there's going to be one AI. It's going to have some kind of utility function.
That utility function is going to be very stable over time. It's not going to.
There won't be this messiness of like a lack of coordination between different AIs or different like over time values drifting for various reasons, maybe because they become less functional in an environment, maybe because of other reasons. And so they just don't imagine that.
They say, well, I mean, utility functions, we can preserve them forever. We have the technology to do that.
So it's just going to happen. And I'm like, well, that seems like such a weak argument to me.
Like, I'm not sure. Actually, if you look at just like, often the idea is because this is like digital, you can preserve the information better and copy it with higher fidelity and so on.
But actually, if you look, even if you look at just like information on the internet,
you have this thing called link rot,
which happens very quickly.
And actually, information that's digital
isn't preserved for very long at all.
And the point that Matthew was making
is that also this like,
the fact that the information is digital
has led to, not maybe led to,
but at least been associated
with faster cultural change.
Cultural change, exactly. I mean, basically, technological changes can create incentives for cultural change just as they make preserve.
That's right. I think there's two key arguments that I've heard.
One is that we will soon reach something called technological maturity. And once you're, one of the key ways in which society has been changing recently is that it's not like maybe actually its culture would have changed even more.
Actually, no, no. I think this argument is wrong that you're making because we do know that language actually changed a lot more.
Like we can read everything that was written after like 1800s when literacy became more common. But it's actually even just go back a couple hundred years after that and you're reading old English and it's hard to understand.
And that is a result of literacy and the codification of language. Well, that information was better preserved.
What about other kinds of cultural practices? But I think the argument would be that maybe they would have actually changed more if – that change was the result of technological change in general, not the result of information being digitized. And maybe culture would have actually changed more if information wasn't as well preserved, but technology had continued to proceed.
And the argument is, in the future, we're going to reach some point at which you've done all the tech. Ideas have just gotten way too hard to find, and you need entire galaxies worth of...
You need to make a CERN that's the size of a galaxy to progress physics an inch forward. And at that point, there's this like growth in technology just churning over civilization goes away.
And then you just have the digital thing, which does mean that like a lock-in is more plausible. So the technological maturity thing, I agree that results in this slowdown and change and growth and so on, and certain things might get more locked in relative to what preceded it.
But then what do we do today about that? Well, like, what could you do to have a kind of positive impact by our lights? And so asking that question, I mean, Robin Hanson had this question of what could someone do in the 1500s to have a positive impact on the world today from their point of view, knowing all they knew back then. I think this question is even worse than that because I think the amount of change that happens between today and technological maturity is just orders of magnitude greater than whatever change happened between the 1500s and today.
So it's an even worse position than someone in the 1500s thinking about what they could do to have an impact, positive impact in expectation, like predictably positive today. And so I think it's just pretty hopeless.
Like, I don't know if we could do anything or find any candidate set of actions that would just make things better, you know, post-lock-in. Or, I mean, that's assuming lock-in is even going to happen, which is not.
In the 1700s, a bunch of British abolitionists were making the case against slavery. And I think the world has, like, we could just live in a slave society.
Like, I don't think there's any in-principle reason why we couldn't have been a slave society to this day or the more the world couldn't have slavery. And I think what happened is just like the convincing of British people that slavery is wrong.
The British empire put all its might into abolishing slavery and making that a norm. I think another example is Christianity and the fact that like Jesus has these ideals.
You could like talk about these ideals. I think like the world is a more Christian place.
It is a more Christian place, sure. And also is like more of the kind of place.
Like I'm not saying Jesus Christ would endorse every single thing that happens in the world today. I'm just saying he endorses this timeline more than one in which he doesn't exist and doesn't preach at all.
I don't know, actually. I mean, I'm not sure if that's true.
It seems like a hard question. I think like some from the Christian perspective favorable cultural developments in the West.
I mean, you don't know the counter mean i'm not sure if that's true it seems like a hard question but uh i think like a some from the christian perspective favorable cultural i mean you don't know the counterfactual i agree that is always true you know i just think like the world does have people who like read the bible and are like i'm inspired by these ideals to do certain things um and it just seems like that's more likely to lead to like that is what i would call like a legacy effect or something i mean you can say the same thing about languages. Some cultures might just become more prominent and their languages might be spoken more.
Or some symbols might become more prominent. But then there are things like how do cities look and how do cars look and what do people spend, what's their time doing in their day and what do they spend their money on.
And those questions seem much more determined by how your values change as circumstances change. That might be true.
But I'm in the position with regards to the future where I'm like, I expect a lot of things to be different and I'm okay with them being different. I care much more about the equivalent of slavery, which in this case is literally slavery.
Just to put a final point on it.
The thing I really care about is there's going
to be trillions of digital beings.
I want it to be the case that they're
not tortured
and put into conditions in which
they don't want to work and whatever.
I don't want galaxies worth of suffering.
That seems closer to
British abolitionists being like, let's put our empire's
might against fighting slavery. I agree.
But I would distinguish between the case of Christianity and the case of end of slavery because I think the end of slavery like I agree you can imagine a society like technologically it's like feasible to have slavery but like I think that's not the relevant thing which brought it to an end the relevant thing is that the change in values associated with the Industrial Revolution made it so that slavery just became like an inefficient thing to sustain in a bunch of ways.
And a lot of countries at different times like phased out different things you could call slavery.
So, for example, Russia abolished serfdom in the 1860s.
They were not under British pressure to do so.
Like, Britain couldn't force Russia to do that. They just did that on their own.
There were various ways in which people in Europe were, like, tied to their land, and they couldn't, like, move. They couldn't go somewhere else.
Those movement restrictions were lifted because they were inefficient. There were ways in which it used to be like the kind of labor that needed to be done in the colonies to grow sugar or to grow various crops.
It was very hard labor. It was not the kind of thing that probably you could have paid people to do because they just wouldn't want to do it because the health hazards and so on were very great, which is why they needed people to force people to do them.
And that kind of work over time became less prevalent in the economy. So again, that reduces the economic incentives to do it.
I agree you could still do it. But I would emphasize it's like the way you're painting the counterfactual is like, oh, but then in that world, they would have just like phased out the remnants of slavery.
But like there's a lot of historical examples where there's not necessarily hard, only hard labor. Like Roman slavery.
Yes. It was different.
And I interviewed a historian about it recently. The episode hasn't come out.
But he wrote a book about like the scope. I think it was like 20% of Roman – people under Roman control were slaves.
And this was not just agricultural slavery. This was like every – like – and his point was that it was this division of – like the maturity of the Roman economy is what led to this level of slavery.
because the reason slavery collapsed in Europe after the fall of the Roman Empire was because the economy just lost a lot of complexity. Well, I'm not sure if I would say that slavery collapsed.
I mean, I think this just depends on what you mean by slavery. I mean, you know, a lot of ways people who in feudal Europe were like- But his point is that actually serfdom was not the descendant institution for Roman slavery.
It was not descendant. But like, in fact, this is sort of the point I'm trying to make is that values that exist at a given time, like what the values of we will have in 300 years or like from the perspective of someone a thousand years ago, what values people are going to have in a thousand years.
Those questions are much more determined by the technological and economic and social environment that's going to be there in a thousand years, which values are going to be functional, which values end up being more competitive and being more influential so that other people like add up their values. And it depends much less on the individual actions taken by people in a thousand years ago.
So I would say that the abolitionist thing, it's not the cause of why slavery came to an end. And slavery comes to an end also because people's own, like people just have natural preferences that I think are suppressed in various ways during the agricultural era.
Where like it's more efficient to have settled societies in like cities which are like fairly authoritarian and don't allow for that much freedom. And you're in this Malthusian world where people have very low wages, perhaps compared to what they enjoyed in the hunter-gatherer era.
So it's just a different economic period. And I think people were not, they didn't evolve to have the values that would be functional in that era.
So what happened is that there had to be a lot of cultural assimilation where people had to adopt different values. And in the Industrial Revolution, people become also very wealthy compared to what they used to be.
And that I think think, leads to different aspects of people values being expressed. Like people just have put a huge amount of value on equality.
It's always been the case. But I think when it is sufficiently functional for that to be suppressed, they are capable of suppressing it.
I mean, if that's a story, then this puts all the more reason, this makes alignment all the more important, or like the value alignment all the more important, because then you're like, oh, if the AI has become wealthy enough, they actually will make a concerted effort to make sure the future looks more like the utility function we put into them, which I think you have been under-emphasizing. No, I'm not under-emphasizing that.
I think like there are, what I would say is there are certain things that are like path dependent in history, such that if someone had done something different, like something had gone differently a thousand years ago, then today in some respects would look different. I think, for example, which languages are spoken across which boundaries or like which religions people have or like which like those kinds of or fashion, maybe to some extent, though not entirely.
Like those things are more path dependent. But then there are things that are not as path dependent.
So for example, if some empire, like if the Mongols had been more successful and like they somehow, I don't know how realistic it is, but like they became like very authoritarian and had slavery everywhere, would that have actually led to slavery being a much more enduring institution a thousand years later? That seems not true to me. Like the forces that led to the end of slavery seemed like they were not like they were not contingent forces.
They seem like deeper forces than that. And if you're saying, well, if we like if we align the AI today to some bad set of values, then that could affect the future in some ways, which are more fragile.
That seems plausible. But I'm not sure how much of the things you care about the future and the ways in which you expect the future to get worse, you actually have a lot of leverage on at the present moment.
I mean, another example here might be factory farming, where you could say, like, oh, us having, it's not like us having better values over time led to suffering going down. In fact, their suffering might have gone up because the incentives that are, that led to factory farming emerging.
And probably when factory farming comes to an end, it will be because the incentives start doing that. Right.
But suppose I care about making sure the digital equivalent of factory farming doesn't happen, where it may be more efficient, maybe in the all else being equal, it's just more economically efficient to have suffering minds doing labor for you than non suffering minds because of the because of the the intermediary benefits of suffering or something Something like that, right? What would you say to somebody like me where I'm like, I really want that not to happen. I don't want the like home filled with suffering workers or whatever.
Is it just like, well, give up because this is the way economic history is headed? No, I don't think you should give up. It's more like it's hard to anticipate the
consequences of your actions in the very distant future.
So, like,
I would just recommend that you should just
discount the future.
Not for, like, a moral reason, not because
the future is worthless or something, but because it's just
very hard to anticipate the effects of your actions.
In the near term, I think there are things you can
do that seem like they would be beneficial.
Like, for example, you could try to align your present AI systems to value the things that you're talking about. Like they should value happiness and they should dislike suffering or something.
You might want to support political solutions that would – like basically you might want to build up the capacity so that in the future if you notice something like this happening then we might have some ability to intervene like maybe you would think about the prospect of well eventually we're gonna maybe colonize other stars and like civilization might become very large and communication delays might be very long between different places and in that case competitive pressures between different local cultures might become much stronger because it's harder to centrally coordinate. That's right.
And so in that world, you might expect competition to take over in a stronger way. And if you think the result of that is going to be a lot of suffering, maybe you would try to stop that.
Again, I think at this point, it's very far from obvious that trying to say limit competition is actually a good idea. I would probably think it's a bad idea.
But maybe in the future, we will receive some information and we'll be like, oh, we were wrong. Actually, we should stop this.
And then maybe you want to have the capacity so that we can make that decision. But that's a very hard, that's a nebulous thing.
How do you build that up? Well, I don't know. I mean, you need to, like that's the kind of thing I would be trying to do.
Yeah, I think the overall takeaway I take from the way that I think about it and I guess we think about it is be more humble in what you think you can achieve. Yeah.
And like just focus on the nearer term, not because it's more morally important than the longer term, but just because it's much easier to have a predictably positive impact on that. One thing I've noticed over the last few weeks of thinking about these bigger picture topics and interviewing Daniel and Scott and then you two is how often I've changed my mind about everything from the smallest questions about when AI will arrive.
It's funny that that's the small question in the grand scheme of things to whether there will be an intelligence explosion or whether there will be an R&D explosion to whether there will be explosive growth or how to think about that. And if you're in a position where you are just like incredibly epistemically uncertain about what's going to happen, I think it's important to just like directly acknowledge like this is instead of just instead of becoming super certain about like your next conclusion, just being like, well, let me just at least from my perspective, I'm just like, let me just take a step back.
I'm like, I'm not sure what's going on here. And I think a lot more people should be from that perspective, unless you've had the same opinion about AI for many years, in which case I have other questions for you about why that's the case.
And in other situations, I mean, generally how we as a society deal with topics on which we are this uncertain is just to have freedom, decentralization, both decentralized knowledge and decentralized decision-making take the reins. And not to do super high volatility centralized moves like, hey, let's nationalize so we can make sure that the software-only singularity is aligned or not to do make moves that are just incredibly contingent on one worldview that are brittle under other considerations.
And that's become a much more salient part of my worldview. I think just classical liberalism is the way we deal with being this epistemically uncertain.
And I think we should be more uncertain than we've ever been in history, as opposed to many other people who seem to be more certain than they are about other sort of more mundane topics. I think it's very hard to predict what happens because of this acceleration basically means that you find it much harder to predict what the world might be in 10 years' time.
I think these questions are also just like very difficult and we don't have very strong empirical evidence. And then there's like a lot of this kind of disagreement that exists.
Like I would say that it's important to like the like in a lot of cases and a lot of situations, it's much more important to be like maintain flexibility and ability to adapt to new circumstances, new information than it is to get a specific plan that's going to be correct and that's very detailed and has a lot of specific policy recommendations and things that you should do. So that's actually also the thing that I would recommend.
If I want to make the transition to AI in this period of explosive growth go better, I would just prefer it if we, in general, like higher quality institutions. But I am much less bullish on someone sitting down today and working out, okay, like what will this intelligence explosion or explosive growth be like? What should we do? Like I think plans that you work out today are not going to be that useful when the events are actually occurring because you're going to learn so much stuff that you're going to update on so many questions that these plans are just going to become obsolete.
That's right. I guess one thing you could do is you could look at, say, the history of war planning and how successful war planning has been for actually anticipating what actually happens when the war actually happened.
So for one example, I think I might have mentioned this off the record at some point, but before the Second World War happened, people were, obviously people saw that there were all these new technologies like tanks and airplanes and so on, which were now, like they exist in World War I, but in a much more primitive setting. So they were wondering what is going to be the impact of these technologies now that we have them in much greater scale.
And the British government had estimates of how many casualties there will be from aerial bombardment in the first few weeks of the Second World War. And they expected hundreds of thousands of casualties, basically, in like two weeks, three weeks after the war begins.
So the idea was that air bombing is basically this unstoppable force. All the major urban centers are going to get bombed.
Tons of people will die. So basically, we can't have a war because if there's a war, then it will be a disaster because we will have this aerial bombardment.
But later, it turned out that that was totally wrong. In fact, in all Britain, there were fewer casualties from air bombing in the entire sort of six years of the Second World War than the British government expected in the first few weeks of the war.
Like they had less casualties in six years than they expected in like a few weeks. So why did they get it wrong? Well, I mean, there are lots of boring practical reasons.
Like, for example, it turned out to be really infeasible to bomb, especially early on, to bomb cities in daytime because your aircraft would just get shot down. But then if you tried to bomb at nighttime, then your bombing was really imprecise and only a very small fraction of it actually hit.
And then people also underestimated the extent to which people on the ground could, like firefighters and so on, could just sort of go around the city and then put out fires from bombs that were falling on structures. They overestimated the amount of economic damage that it would do.
They underestimated how economically costly it would be. Like basically, you're sending these aircraft and then they're getting shot down.
Well, an aircraft is very expensive. So in the end, what turned out is when the Allies started bombing Germany, they were like for each dollar of capital they were destroying in Germany, they were spending like $4 to $5 on the aircraft and fuel and training of the pilots and so on that they were sending in missions.
And the casualty rate was very high, which later got covered up by the government because they didn't want people to worry about, you know. Yeah.
So that is a kind of situation where all the planning that you would have done in advance
predicated on this assumption of like air bombing is going to be this like nuclear weapons
light, basically.
Like it's extremely destructive.
There's going to be some aspect to which.
I mean, it was so right.
Like 84,000 people died in one night of fire bombing in Tokyo, like Germany, like large fractions of their industrial world. But that was over the period of six years of war.
But there were like single firebombing attacks. I mean, it was a case that during the end of World War II, when they were looking for the place to launch the atomic bombs.
That's right. They just had to go through like a dozen cities because they just wouldn't be worth nuking them because they're already destroyed by the firebombing.
That's right. But if you look at the level of destruction that was expected within the space of a few weeks, and then this level of destruction took many years.
So there was like a two-order magnitude mismatch or something like that, which is pretty huge. Yeah.
And that affected the way people think about it. Right.
An important underlying theme of much of what we have discussed is how powerful just reasoning about things is to making progress about what specific plans you want to make to prepare and make this transition to advanced AI go well. Yeah.
And our view is, well, it's actually quite hard and you need to make contact with the actual world in order to inform most of your beliefs about what actually happens. And so it's somewhat futile to think, to do a lot of kind of wargaming and figure out, you know, how AI might go and what we can do today to make that go a lot better because a lot of the policies you might come up with might just look fairly silly.
And I think there's in the thinking about how AI actually has this impact. Again, people think, oh, you know, just AI reasoning about doing science and doing R&D just has this drastic impact on, you know, the overall economy or technology.
And our view as well, actually, again, making contact with the real world and getting a lot of data from experiments and from deployment and so on, it's just very important. So I think there is this underlying kind of latent variable, which explains some of this disagreement, both on the policy prescriptions and about the extent to which we should be humble versus ambitious about what we ought to do today, as well as for thinking about the mechanism through which AI has this impact.
And this underlying latent thing is like, what is the power of reason? Like how much can we reason about what might happen? How much can reasoning in general figure things out about the world and about technology? And, you know, so that is like a kind of core underlying disagreement here. Yeah.
I do want to ask, you say in your announcement, we want to accelerate this broad automation of labor as fast as possible. As you know, many people think it's a bad idea to accelerate this, the broad automation of labor and AGI and everything that's involved there.
Why do you think this is good? So the argument for why it's good is that we're going to have this enormous increase in economic growth, which is going to mean like enormous amounts of wealth and like incredible new products that you can't even imagine and like healthcare or whatever. And like the quality of life of the typical person is probably going to go up a lot.
Early on, probably also their wages are going to go up because the AI systems are going to be automating things that are complementary to their work. Or like it's going to be automating part of their work and then you'll be doing the rest and then you'll be getting paid much more on that.
And in the long term, eventually we do expect wages to fall just because because of arbitrage with DAIs. But by that point, we think humans will own enormous amounts of capital.
And there will also be like ways in which even the people who don't own capital, we think are just going to be much better off than they are today. Like, I think it's just hard to express in words the amount of wealth and increased variety of products that we would get in this world, it would be probably more than a difference between like 1800 and today.
So if you imagine that difference, it's like such a huge difference. And then we imagine like two times, three times, whatever.
The standard argument against this is why does the speed to get there matter so much? Because especially if the tradeoff against the speed is the probability that this transition is achieved successfully in a way that benefits humans.
I mean, it's unclear that this trades off against the probability of it being achieved successfully or something.
There might be an alignment tax.
I mean, maybe.
You can also just do the calculation of how much a year's worth of delay costs for current people.
This enormous amount of utility that people are able to enjoy, and that gets brought forward by year or pushed back by year if you delay things by year. and how much is this worth?
Well, you know, you can look at simple models of how concave people's utility functions are
and do some calculations. And maybe that's worth on the order of tens of trillions of dollars per year in consumption.
That is roughly the amount consumers might be willing to defer in order to get, you know, bring forward the date of automation one year. In absolute terms, it's high.
In relative terms, relative to if you did think it was going to nudge the probability one way or another of building systems that are aligned and so forth, then it's like just so small compared to all of the future. I agree.
So, like, there are a couple of things here. First of all, I think the way you think about this matter.
So, first of all, we don't actually think that it's clear whether speeding things up or slowing things down actually makes like do me outcome more or less likely.
Like, I think that's just a question that doesn't seem obvious to us. Like we don't, like partly because of our views on the software R&D side, we don't really believe that if you just pause and then you like do research for 20 years at a fixed level of compute scale, that you're actually going to make that much progress on relevant questions on alignment or something.
Like I think – like imagine you were trying to make progress on alignment in 2016 with the compute budgets of 2016. And like, well, you would have gotten nowhere basically.
Like you would have discovered none of the things that people have today discovered and that turned out to be useful. And I think if you pause today, then we will be in a very similar position in 10 years, right? Like, we would have not made a bunch of discoveries.
So, the scaling is just really important to make progress and alignment, in our view. And then there's a separate question of how long-term should you be in a very different sense.
So there's a moral sense where like how much should you actually care about people who are alive today as opposed to people who are not yet born? That's just a moral question. And there's also a practical question of, as we discussed, how certain can you be about the impact your present actions are actually going to have on the future? Okay.
Maybe you think it really doesn't matter whether you slow things down right now or you speed things up right now. But is there some story about why speeding them up from the alignment perspective actually helped? It's good to have that extra progress right now rather than later on? Or is it just that, well, if it doesn't make a difference either way, then it's better to just get that extra year of people not dying and having cancer cures and so forth.
I think I would say the second.
But it's just important to understand the value of that, right?
Even in purely economic terms.
Like imagine that you would be like each year of delay might cause like maybe 100 million people, maybe more,
maybe 150, 200 million people who are alive today to end up dying. So even in purely economic terms, the value of a statistical life is pretty enormous, especially in Western countries.
Sometimes people use numbers as high as $10 million for a single life. So imagine you do like $10 million times 100 million people.
That's like a huge number, right? So I think that is just so enormous that unless you're just... So I think for you to think that speeding things up is a bad idea, you have to first be like just have this long-termist view where you look at the long-run future, you think your actions today have high enough leverage that you can predictably affect the direction of long-term future.
In this case, it's kind of different because you're not saying I'm going to affect what some emperor a thousand years from now does, like somebody in the year zero would have to do to be a long-termist. In this case, you just think there's this incredibly important inflection point that's coming up and you just need to have influence over that crucial period of explosive growth of intelligence explosion or something.
So I think it is a much more practicable prospect than... So I agree in relative terms.
So like in relative terms, I agree the present moment has like is a moment of higher leverage and you can expect to have more influence. I just think in absolute terms, the amount of influence you can have is still quite low.
So it might be orders of magnitude greater than it would have been 2000 years ago, and still be quite low. And again, I think there's this, like, difference in opinion about how broad and diffuse this transformation ends up being versus how concentrated within a specific labs where the very idiosyncratic decisions made by that lab will end up having very large impact.
If you think those developments will be very concentrated, then you think the leverage is especially great. And so then you might be especially excited about having the ability to influence how that transition goes.
But our view is very much that this transition happens very diffusely by way of many, many organizations and companies doing things. And for those actions to be determined a bunch by economic forces rather than kind of idiosyncratic preferences, you know, on the part of labs or these kind of decisions that have these kind of founder effects that last for very long.
Right. Okay, let's go to some of the objections to explosive growth, which is most people are actually more conservative, not more aggressive about the forecast you have.
So obviously one of the people who has articulated their disagreements with your view is Tyler Cowen. He made an interesting point when we did the podcast together and he said, most of sub-Saharan Africa still does not have reliable clean water.
The intelligence required for that is not scarce. We can not so readily do it.
We are more in that position that we might like to think along other variables. I mean, we agree with this.
Like, I think, like, intelligence isn't the bottleneck that's holding back, you know, technological progress or economic growth. Right.
It's like many other things. And so I think that this is very much consistent with our view that scaling up your overall economy, accumulating capital, accumulating human capital, having, you know, all these factors scale.
In fact, this is even consistent with what I was saying earlier, that I was pointing out this like, oh, like good management and like good policies and those just contribute to TFP and they can be bottlenecks. But like right now, we could just plug and play our better management into Sub-Saharan Africa.
No, we can't. Because it's hard.
I don do that. Okay, so that's what I was, maybe I should have said.
One could theoretically imagine plugging and playing with hard. Yeah, I can imagine many things.
But we cannot so readily do it because it's hard to articulate why and it wouldn't be so easy to do in just capital or labor. Why not think that the rest of the world will be in this position with regards to the advances that AI will make possible? I mean, if the AI advances are like the kind of geniuses in a data center, then I agree that that might be bottlenecked by the rest of the economy not scaling up and being able to accumulate the relevant capital to make those changes feasible.
So I kind of agree with this picture. And I think this is like, you know, an objection to the geniuses in a data center type view.
And like, I buy basically this. And also the fact that like, it's also plausible, you're going to have the technology, but then some people are not going to want to deploy it, or some people are going to have norms and laws and cultural things that are going to make it so that AI is not able to be widely deployed in their economy, or not as widely deployed as otherwise it might be.
And that is going to make those countries or societies just slower. Like some countries will be growing faster, just like Britain and the Netherlands were sort of the leaders in the industrial revolution.
They were the first countries to start experiencing rapid growth.
And in other countries, even Europe sort of had to come from behind.
Well, again, I just think we expect the same thing to be true for AI. And I mean, the reason that happened was exactly because of these kinds of reasons, where those countries that are culture or governance systems or whatever, which were just worse than bottlenecked
the deployment and scaling of the new technologies and ideas, it seems very plausible. But you're saying as long as there's one jurisdiction.
Yeah. But then again, you also previously emphasized the need to integrate with the rest of the global economy and the human economy.
So doesn't that contradict? That doesn't often require cultural homogeneity. Like we can, we trade with countries, the U.S.
trades with China quite a lot actually, and there's like a bunch of disagreements. But if the U.S.
is like, I don't like the UAE is doing explosive growth with AI. We're just going to like embargo them.
That seems plausible. And then would that not prevent explosive growth? I mean, like, I think that would be plausible at the point at which it's revealing a lot about the capabilities and the power of AI.
Yeah. And you should also think that that creates both an incentive to embargo, but also an incentive to adopt the very similar styles of governing that enable AI to be able to produce a lot of value? What do you make of this? I think people interpret explosive growth from an arms race perspective.
And that's often why they think in terms of public-private partnerships for the labs themselves. But just this idea that you have the geniuses at the data center, you can have them them come up with the mosquito drone swarms and then those drone swarms will you know like if china gets to the swarms earlier i mean even within your perspective where it's like not is this a result of your whole economy being advanced enough that you can produce mosquito drone swarms you being six months ahead means that you could decisively win.
Is it? I don't know.
Maybe you being like a year ahead in explosive growth means you could decisively win a war against China or China could win a war against you. So would that lead to an arms race dynamic? I mean, I think it would to some extent.
But I'm not sure if I would expect that like a year of lead to be like enough to take a risk. Because if you go to war with China, I mean, for example, if the US went to war with,
if you replace China today with China, I mean, for example, if the US went to war with, if you replace China today with China from 1990, or if you replace Russia today with Russia from 1970 or 1980, it's possible that their ICBM and whatever technology is already enough. It's already enough to have very strong deterrence.
So maybe that lead, a technological lead, is not sufficient so that you would feel comfortable going to war. So that seems possible.
Yeah. And actually this relates to a point that Guern was making, which is he was like, okay, this is going to be a much more unstable period than the Industrial Revolution.
Even though the Industrial Revolution saw many countries gain rapid increases in their capabilities. Because this is just like within this span, if you've got a century's worth of progress compressed within a decade, one country gets to like ballistic missiles first, then the other country gets to railroads first and so forth.
But if you have this more integrated perspective about what it takes to get to ballistic missiles and to railroads, then you might think, no, basically, this isn't some orthogonal vector.
It just like you're just like churning on the tech tree further and further.
Yeah. I mean, for what it's worth, I do think like it's possible if you have it just happen in a few countries which are relatively large and have enough land or something, like those countries could just like they would be starting from a lower base compared to the rest of the world.
So they would need to catch up to some extent. So like if they are just going to sort of grow internally and they're not going to depend on the external supply chains.
But like that doesn't seem like something that's impossible to me. Some countries could do it.
It would just be more difficult. But in this setting, if some countries have a significant policy advantage over the rest of the world and they start growing first and then they won't necessarily have a way to get other countries to adopt their norms and culture.
So in that case, it might be more efficient for them to do the growth locally. So that's why I was saying the growth differentials will probably be determined by regulatory jurisdiction boundaries more than anything else.
I'm not saying, say, the US by itself, it had AI, but it couldn't get the rest of the world to adopt AI. I think that would still be sufficient for social growth.
How worried should we be about the fact that China today just has, because it industrialized relatively recently, just has more industrial capacity and know-how and all the other things, learning by doing and so forth. If we buy your model of how technology progresses, with or without AI, are we just underestimating China? Because we have this perspective that what fraction of your GDP you're spending on research is what matters.
When in fact, it's the kind of thing where I've got all the factories in my backyard and I know how they work and I can go buy a component whenever I want. I don't think people are necessarily underestimating China.
I mean, it depends on who you're looking at. But it seems like the discussion of China is just this very big discussion in these AI circles, right? And so people are very much appreciating the power and the potential threat that China poses.
But I think the key thing is not just like the scale in terms of pure number of people
or like number of firms or something, but the scale of the overall economy, which is
just measured in how much is being produced in terms of dollars. And there, you know, the U.S.
is ahead. But doesn't the – like we're not expecting all this explosive growth to come from financial services.
We're expecting it to start from a base of industrial technology and industrial capacity. I don't even know.
Financial services can be important if you want to scale very big projects very quickly. Financial services are very important for raising funding and getting investments in data centers.
If I understood you correctly, it just seems like man, you know how to do all the you know how to build the robot factories and so forth. That know-how, which in your view is still crucial to technology growth and just general economic growth, is lacking.
And you might have more advanced financial services. But it seems like the more you take your review seriously, the more it seems like having the Shenzhen locally matters a lot.
Relative to what starting point. I think people already appreciate that China is very important.
And then I agree that there are some domains where China is leading, but then there
are very many domains in which the US is leading or the US and its allies where countries that are
producing relevant inputs for AI that the US has access to, but China doesn't. So I think the US
is just ahead on many dimensions. And there's some that China is ahead or at least very close.
So I don't think this should cause you to update very strongly in favor of China being a much bigger deal, at least depending on where you start. I think people already think China is a big deal.
This is the big underlying thing here. If people are just very dismissive of China, then maybe this would be a reason to update.
I get your argument that thinking about the economy-wide acceleration is more important than focusing on the IQ of the smartest AI. But at the same time, do you believe in the idea of superhuman intelligence? Is that a coherent concept? In way that you don't necessarily stop at human level GoPlay and you just go way beyond it in ELO score? Will we get to systems that are like that with respect to the broader range of human abilities? And maybe that doesn't mean they become God because there's other ASIs in the world.
But you know what I mean? Will there be systems with such superhuman capabilities? Yeah, I mean, I do expect that. I think there's a question of how useful is this concept for thinking about this transition to a world with much more advanced AI.
And I don't find this like a particularly meaningful or helpful concept. Like, I think people introduce some of these notions that on the surface seem useful, but then actually when you delve into them, it's like very vague and kind of unclear what you're supposed to make of this.
And you have this notion of AGI, which distinguishes from narrow AI in the sense that it's much more general and maybe, you know, can do everything that a human can do on average. I mean, AI systems have these very jagged profiles of capabilities, so you have to somehow take some notion of average capabilities.
And what exactly does that mean? It just feels really unclear. And then you have this notion of ASI, which is AGI in the sense that it's very general, but then it's also better at humans than on every task.
And is this a meaningful concept? I guess it's coherent. I think this is not a super useful concept because I prefer just thinking about, you know, what actually happens in the world.
And you could have a drastic acceleration without having an AI system that can do everything better than humans can do. I guess you could have no acceleration when you have an ASI that is better than humans at everything, but it's just very expensive or very slow or something.
So I don't find that particularly meaningful or useful. I just prefer thinking about the overall effects on the world and what AI systems are capable of producing those types of effects.
Yeah, I mean, one intuition pump here is compare John von Neumann versus a human reflect from the standard distribution. If you added a million John von Neumanns to the world, what would the impact on growth be as compared to just adding a million people from normal distribution? Well, I agree it would be much greater.
Right. But then like, because of more of Axe Paradox type arguments that you made earlier, that evolution has not necessarily optimized us for that long along the kind of spectrum on which John von Neumann is distinguished from the average human.
And given the fact that already within this deviation, you have this much greater economic impact,
why not focus on optimizing on this thing
that evolution does not optimize that hard on further?
I don't think we shouldn't focus on that.
But what I would say is, for example,
if you're thinking about the capabilities of Go playing AIs,
then the concept of a superhuman Go AI,
yeah, you can say that is a meaningful concept. But if you're developing the AI, it's not a very useful concept.
If you're like, if you just look at the scaling curve, it's just like it just goes up and there's some human level somewhere. But like the human level is not privileged in any sense.
So the question is like, is it a useful thing to be thinking about? And the answer is probably not. It depends on what you care about.
So i'm not saying we shouldn't focus on trying to make the system smarter than humans are like i think that's a good thing to focus on yeah i guess i'm trying to understand whether we will stand in relation to the ais of 2100 that humans stand in relationship to other primates like is that like is that the right mental model we should have or is there going to be a much greater familiarity with their cognitive horizons? I mean, I think AI systems will be very diverse, and so it's not super meaningful to ask something about this very diverse range of systems and where we stand in relation to them. Will we be able to cognitively access the kinds of considerations they can take on board? Humans are diverse, but no chimp is going to be able to understand this argument in the way that another human might be able to, right? So I'm just like, if I'm trying to think about my place or a human's place in the world of the future, I think it is a relevant concept of, is it just that the economy has grown a lot and there's much more labor? Or are there beings who are in this crucial way super intelligent? I mean, there will be many things that we just will fail to understand.
And to some extent, there are many things today that people don't understand about how the world works and how certain things are made. And then how important is it for us to have access or, in principle, be able to access those considerations? And I think it's not clear to me that that's particularly important, that, like, any individual human should be able to access all the relevant considerations that produce some outcome.
Like, that just seems like overkill. Like, why do you need that to happen? I think it would be nice in some sense.
But I think if you want to have a very sophisticated world where you have very advanced technology, those things will just not be accessible to you. And then, so you have this trade-off to an accessibility and maybe how advanced the world is.
And from my point of view, I'd much rather live in a world which has very advanced technology, has a lot of products that I'm able to enjoy, and a lot of inventions that I can improve my life with, if that means that I just don't understand them. I think this is a very simple trade that I am very willing to make.
Okay, so let's get back to objections to explosive growth. We discussed a couple already.
Here's another which is more a question than an objection. Where is all this extra output going? Like who is consuming it? If the economy is 100x bigger in a matter of a decade or something like to what end? first of all, I think even if you view that along what you might call the intensive margin in the sense that you just have more of the products you have today, I think there is just a lot of, like there will be a lot of appetite for that.
Maybe not quite 100x, but like that might start hitting some diminishing terms. Current GDP per capita on average in the world is 10K a year or something, right? And there are people who enjoy millions of dollars.
And so there's a gap between what people enjoy and don't seem to be super diminished in terms of marginal utility. And so there's a big room.
There's a lot of room on just purely the intensive margin of just consuming the things we consume today, but more. And then there's this maybe much more important dimension along which we will expand, which is… Product for IT.
Yeah. Extensive margin of what is the scope of things that you're consuming.
And if you look at something like the Industrial Revolution, that seemed to have been the main dimension along which we kind of expanded to consume more. There's just on any kind of sector that you care about, transportation, medicine, entertainment, and food, there's just this massive expansion in terms of variety of things that we're able to consume that is enabled by new technology or new trade routes or new methods of producing things.
And so that is, I think, really the key thing that we will see come along with this kind of expansion in consumption. Another point that Tyler makes is that there will be some mixture of Bamul cost disease where you're bottlenecked by the slowest growing thing, which grows in proportion.
The fastest productivity things basically diminish their own share and output. That's right.
Yeah. I mean, like we totally agree with that.
I would say that that's just like a kind of qualitative consideration. It doesn't
itself, it isn't itself sufficient to make a prediction about what growth rates are permitted given these bottom effects versus not. It's just like a qualitative consideration and then you might need to make additional assumptions to be able to make a quantitative prediction.
So I think it's a little bit...
So the like commissing version of this argument would be if you did the same thing that we were doing earlier with the software only singularity argument, where we were pointing to essentially the same rejection, where there are multiple things that can bottleneck progress. So I would be much more convinced if someone pointed to like an explicit thing, they would be like, here, like healthcare is like this very important thing.
And why should we expect AI to like make that better? Like that doesn't seem like that would get better because of AI. So like that maybe healthcare just becomes a big part of the economy and then that bottleneck.
So like if there was some specific sector. Maybe the argument is that if there's even one.
No, if there's one though, like if that's a small part of the economy, then you could just still get a lot of growth. You just automate everything else.
And that is going to produce a lot of growth. So it has to, like, quantitatively work out.
And so you actually have to be quantitatively specific about what this objection is supposed to be. Right.
So first of all, you have to be specific about, OK, what are these tasks? What are the current share in economic output? The second thing is you have to be specific about how bad do you think the complementarities are. So in numerical terms, economists use the concept of elasticity of substitution to quantify this.
So that gives you a numerical estimate of if you just have much more output on some dimensions, but not that much on other dimensions, how much does that increase economic output overall? And then there's a third question. You can also imagine you automate a bunch of the economy.
Well, a lot of humans were working on those jobs. So now, well, they don't need to do that anymore because those got automated.
So they could work on the jobs that haven't yet been automated. So for example, as I gave the example earlier, you might imagine a world in which remote work tasks get automated first, and then sensory motor skills lag behind.
So you might have a world in which software engineers like become physical workers instead. Of course, in that world, the wages of physical workers will be much higher than their wages are today.
So that reallocation also produces a lot of extra growth, even in the, like if bottlenecks are maximally powerful. Like even if it's literally you just look at all the tasks in the economy and literally take the worst one for productivity growth, you would still get a lot of increase in output because of this reallocation.
So I think one point that I think is useful to make our experience talking to economists about this is that they will bring up these kind of more qualitative considerations, whereas the arguments that we make are like make specific quantitative predictions about growth rates. So for example, you might ask like, how fast will the economy double? And then we can think about, you know, an H100 does about, there are some estimates of how much computation the human brain does per second, and it's about one E15 flopper, so it's a bit unclear.
And then it turns out that an H100 roughly does on that order of computation. And so you can ask the question of how long does it take for an H100 to pay itself back? If you run the software of the human brain.
If you run the software of the human brain, you can then deploy that in the economy and earn, say, human wages on the order of 50 to 100k a year or whatever in the US. And so then it pays itself back because it costs on the order of 30k per H100.
And so you get a doubling time of maybe on the order of a year, right? And so this is like a very quantitatively specific prediction about, you know, and then there's the response, well, you have BOMO effects. And then like, okay, well, what does this mean? Like, does it double? Does this predict it doubles every two years or every five years? Like, you need just more assumptions in order to make this a coherent objection.
And so I think a thing that's a little bit confusing is just that there are these qualitative objections that I agree with. Like bottlenecks are indeed important, which is part of the reason I'm more skeptical of this software singularity story.
But I think this is not sufficient for blocking explosive growth. The other objection that I've heard often, and it might have a similar response from you, is this idea that a lot of the economy is comprised of O-ring type activities.
And this refers to, I think, in the Challenger space shuttle explosion. There is just like one component.
I forgot what the exact problem with the O-ring was. But because of that being faulty, the whole thing collapsed.
I mean, I think it's quite funny, actually, because the O-ring model is taking the product of many inputs. And then the overall output is the product of very many things.
That's right. And so, but actually this is like pretty optimistic from the point of view of having fewer bottlenecks.
I think we pointed this out before, which again, talking about software on the Singularity, I said like if it's the product of compute for experiments with research reference. But if one of those products is zero.
Because of human. But you have constant marginal product there, right? No, but yeah.
But if one of those products doesn't scale, that doesn't limit. Like, yeah, it means you're less efficient at scaling than you otherwise would be, but you can still get a lot of it.
You can just have unbounded scaling in the all-ring world. So actually, I disagree with Tyler that he's not conservative enough, that he should take his bottlenecks view more seriously than he actually is.
And yet, I disagree with him about the conclusion, and I think that we're going to get explosive growth once we have AI that can flexibly substitute I'm not sure I understand. There will be entirely new organizations that AI has come up with.
We've written a blog post about one such with the AI firms. And you might be a productive worker or a productive contributor in this existing, the organizations that exists today, in the AI world, many humans might just be like zero or even minus.
I agree. Why won't that put that in the multiplication? But why would humans be in the loop there? Like you're both saying that humans would have, like, humans would be, like, negatively contributing to output.
Yeah. But then you're also saying that, like, we should put them into the, like, it seems like these.
We should involve them in the system. Okay.
Fair, fair, fair. The main objection often is regulation.
And I think we've addressed it implicitly in different points, but might as well just explicitly address why won't regulation stop this. Yeah.
So for what it's worth, like, we do, we do have a paper where we go over all the arguments for against explosive growth. Yeah.
And regulation, I think, is the one that seems like strongest, that's against. Because the reason it seems strong is because even though we have made arguments before about international competition and like variational policies among jurisdictions and these strong incentives to adopt this technology, both for economic and national security reasons.
So I think those are pretty compelling when taken together. But even still, like the world does have a surprising ability to like coordinate on just not pursuing certain technologies.
Right. Human cloning.
That's right. So I think like it's hard to be extremely confident that this is not going to happen like I think it's less likely that we're going to do this for AI than it is for human cloning but because I think human cloning touches on some other taboos and so on right and also less valuable and also less valuable but and probably less important also for national security in an immediate sense but at the same time as I as I said, it's just hard to rule this out.
So I wouldn't say, like, if someone said, well, I think, like, there's a 10% or 15%, whatever, 20% chance that, like, there will be some kind of global coordination of regulation, and that's going to just be very effective. Maybe it will be enforced through, like, sanctions on countries that defect or, you know, and then that is going to, like, maybe it doesn't prevent AI from being deployed, but maybe just like slow things down enough that you never quite get exposed for growth.
Like, I don't think that's an unreasonable view. It's like 10% chance.
Could be. I think, I don't know if there's any, I don't know, do you encounter any other? Any other objections? What should I be hassling you about? Yeah, I mean, some things that we've heard from economists, like, again, there was this argument that, like, people sometimes respond to our argument about explosive growth, which is an argument about growth levels.
So we're saying we're going to see 30% growth per year instead of 3%. They respond to that with an objection about levels.
So they say, well, how much more efficient, how much more valuable can you make like hairdressing or like taking flights or whatever or going to a restaurant? And like that is just fundamentally the wrong kind of objection. Like we're talking about the rate of change and you're objecting to it by making an argument about the absolute level of productivity.
And as I said before, it is not an argument that economists themselves would endorse if it was made about a slower rate of growth continuing for a longer time. So it seems more like special pleading.
I mean, why not just the deployment thing where the same argument you made about AI,
where you do learn a lot just by deploying to the world and seeing what people find useful. Chad GPT was an example of this.
Why won't a similar thing happen with AI products and services where you're just, if one of the components is you put it out to the marketplace and people play with it and you find out what they need and it clings into the existing supply chain and so forth. Doesn't that take time? I mean, it takes time, but it is often quite fast.
In fact, ChatGPT grew extremely fast, right? Right, but that was just purely digital service. Well, I think the important thing would be, like, yeah, one reason to be optimistic is if you think the AIs will literally be drop workers or dropping workers in some cases if you have robotics then um companies are already kind of experienced at onboarding humans like onboarding humans doesn't take like a very long time like maybe it takes six months for like a even in a particularly difficult job for a new worker to like start being productive um well that's not that long so i I don't think that would rule out like companies being able to onboard AI workers, assuming that they don't need to make like a ton of new complementary innovation and discoveries to like take advantage.
I think one way in which current AI systems are being inhibited and the reason we're seeing the growth maybe be slower than you might otherwise expect is because companies in the economy are not used to working with this new technology. They have to rearrange the way they work in order to take advantage of it.
But if AI systems were literally able to substitute for human workers, then, well, the complementary innovations might not be as necessary. Actually, this is a good excuse to maybe go to the final topic, which is AI firms.
So this is this blog post we wrote together about what it would be like to have a firm that is fully automated. And the crucial point we were making was that people tend to overemphasize and think of AI from the perspective of how smart individual copies will be.
And if you actually want to understand the ways in which they are superhuman, you want to focus on their collective advantages, which because of biology, we are just precluded from, which are the fact that they can be copied with all their tacit knowledge. You can copy a Jeff Dean or Elias Hatsky or whatever the relevant person is in a different domain.
You can even copy Elon Musk and he can be the guy who's every single engineer in the SpaceX rig. And if that's not an efficient way to...
The equivalent of them. Yeah, yeah.
And if that's... It's not best to have Elon Musk or anything.
You just copy the relevant like team or whatever that... Yep.
And we have this problem with human firms where there can be very effective teams or groups, but over time their culture dilutes or the people leave or die or get old. And this is one of the many problems that can be solved with these digital firms where you actually, firms right now have two of the three relevant criteria for evolution.
They have selection and they have variation, but they don't have high fidelity replication. And you could imagine a much more fast-paced and intense sequence of evolution for firms once you have this final piece click in.
And that relates to the onboarding thing where right now, they just aren't smart enough to be onboarded as full workers.
But once they are, I just imagine for my own, the kinds of things I try to hire for, it would just be such an unlock. It doesn't even matter.
The salary is totally secondary. The fact that I can like, this is the skill I need or the set of skills I need and I can have a worker and just like I can have a thousand workers in parallel if there's something that has a high elasticity of demand, I think is like probably along with the transformative AI, the most underrated tangible thing that like you need to understand about what the future AI society will look like.
Right. I mean, I think there's a point, there's a first point about this like very macroeconomic picture where you just expect a ton of scaling of all the relevant inputs.
And I think that is like the first order thing. Yeah.
But then you might have more like micro questions about, okay, like how does this world actually look like? How is it different from a world in which we just have a lot more people and a lot more capital and a lot more like, you know, because it should be different. And then I think these considerations become important.
I think another important thing is just that AIs can be aligned. Like, you get to control the preferences of your AI systems in a way that you don't really get to control the preferences of your workers.
Like, your workers, you can just select, you don't really have any other option. But for your AIs, you can, like tune them, you can like build AI systems, which have the kind of preferences that you want.
And you can imagine that's like dramatically changing basic problems that determine the structure of human firms. Like for example, the principal agent problem might go away.
Like this problem where the you as a worker have incentives that are either different from those of your manager or those of the entire firm or those of the shareholders of the firm. I actually think the incentives is a smaller piece of the puzzle.
I think it's more about like bandwidth and information sharing where it's often just with a large organization, it's very hard to have a single coherent vision. And the most of social firms we see today is where for an unusual amount of time, a founder is able to keep their vision instilled in the organization, like SpaceX or Tesla are examples of this.
People talk about NVIDIA this way, but just imagine a future version where there's this hyper-inference-scaled mega Jensen who you're spending $100 billion a year on inference on.
And copies of him are constantly, you know,
like writing every single press release
and reviewing every pull of request
and answering every customer service request and so forth.
And monitoring the whole organization,
making sure it's like proceeding along a coherent vision
and getting merged back into the hyper Jensen. Hyper Jensen.
Mega Jensen, whatever. Yeah.
Yeah, I agree that's a bigger deal. At the same time, I would point out that part of the reason why it's important to have a coherent vision and culture and so on in human companies might be that there's incentive problems exist otherwise.
I mean, I wouldn't rule that out, but I like, aside from the overall macroeconomic thing, I think the fact that they can be replicated is probably the biggest deal. That's right, that's right, that's right.
Like, that also enables additional sources of economies of scale, where if you have, like, twice the number of GPUs, you can run not only twice the number of copies of your old model, but then you can train a model that's even better. So you double your training compute and your inference compute,
and that means you're not only double, like
you don't get just twice the number
of workers you would have had otherwise. You get more
than that, because they're also smarter, right?
Because you spend more training compute.
So then that is additional sorts of economies
of scale, and then there's this benefit that
you can, like for humans,
you, like every human
has to learn things from scratch, basically.
Like they are born, and then they have a certain amount of lifetime
learning that they have to do. So in human learning, there is a ton of duplication.
While for an AI system, it could just learn once. It could just have one huge training run, which are tons of data.
And then that run could be deployed everywhere. So that's like another massive advantage that the AIs have over humans.
Yeah. Maybe we'll close up with this one debate we've often had offline, which is will central planning work with these economies of scale? So I would say that, I mean, again, the question of like will it work? Will it be optimal? Right.
So, I mean, my guess is probably not optimal. But like I think it's hard.
I don't think anyone has thought this question through in a lot of detail. So it's worth thinking about just why one might expect central planning to be slightly better in this world.
Right. So one consideration is just communication bandwidth being potentially much, much greater than it is today.
And then like in the current world, the information gathering and the information processing are like co-located. Like humans observe and also process what they observe.
In an AI world, you can disaggregate that. That's actually a really interesting point.
So you can have the sensors and not do much processing, but just collect and then process centrally. And that processing centrally might make sense for a bunch of reasons.
And you might get economies of scale from having more GPUs that produce better models and also be able to think more deeply about what it's seeing. It's worth noting that certain things already work like this.
For example, Tesla FSD. It will benefit from the data collected at the periphery from millions of miles of driving.
And then the improvements which are made as a result of this. Centrally directed.
It's like coming from the HQ being like, we're going to push an update. That's right.
And so you do get some of this more centralized planning behavior. And it can be a much more intelligent form than just whatever gradient averaging that they – I mean, I'm sure it's more sophisticated than CESLA, but it can be a much more like deliberate, intelligent update.
So that's one reason to expect. And the other reason, I guess, is just having like current leaders or CEOs don't have bigger brains than the workers do.
Maybe a little bit. I don't know if you want to open that.
But not by orders of magnitude. That's right.
And so you could have just orders of magnitude more scaling of the size of the models that are doing the planning than the people or the agents or workers doing the actions. And I think a third reason is the thing about the incentive thing, where you wouldn't face this problem that part of the reason you have a market is that it gives people the right kind of incentives, but you might not need that as much if you're using AI.
So I think there's an argument that if you just list the traditional arguments people have made against, like why does central bank not work, then you might expect them to become weaker. Now, I think that is still like there's a danger when you're doing that kind of analysis to fall into the same kind of like partial equilibrium analysis where you're only considering some factors and then you're not considering other things.
Like, for example, you consider. Get more more complex.
You just have a much bigger economy. And so, like, on the one hand, your ability to kind of collect information and process it improves.
But also, the need for doing that also increases as things become more complex. And I mean, one way to illustrate that is, like, imagine if Apple, the organization today, with all its compute and whatever, was tasked with managing the economy of work.
I think it actually could centrally plan the economy. The economy of work might work even better as a result.
But Apple, as it exists today, cannot manage the world economy as it exists today. That's right.
I think that's a good, yeah. Yeah.
Actually, this will be the final question. Look, one of the things that makes AI so fascinating is that there's no domain of human knowledge that is irrelevant to studying it because we're really trying to – I don't know about that.
Fine, fine, fine. There's no serious domain of human knowledge.
That's better. That is not relevant to studying it because you're just fundamentally trying to figure out what a future society will look like.
And so, like, obviously computer science is relevant, but also economics is even discussing history and how to understand history and many other things you've been discussing, right? Especially if you have longer timelines and there is enough time for somebody to pursue a meaningful career here. What would you recommend to somebody? Because both of you are quite young.
I mean, you especially, I gave it i gave it like both of you so it's like this is not you would think this is the kind of thing which requires crystallized intelligence or whatever especially given what we said earlier about look as we get more knowledge we're going to have to factoring what we're learning into building a better model of what's going to happen to the world and if somebody is interested in this kind of career that you both have, what advice do you have for them? Yeah, that's a hard question. I mean, I'm not sure.
Like, I think there is an extent to which it's difficult to deliberately pursue like the implicit strategy that we would have pursued. Like it probably works better if it's spontaneous and like more driven by curiosity and interest than like you make a deliberate choice.
Okay, like I'm just going to learn about a bunch of things so that I can contribute to the discourse and AI. I would think that strategy is probably less effective.
At least I haven't seen anyone who deliberately used that strategy and then was successful. It seems like.
Yeah, I guess I, not that I've contributed to discourse directly, but maybe facilitated other people contributing. I guess it wasn't deliberate strategy on my end, but it was a deliberate strategy to do the podcast, which inadvertently gave me the opportunity to learn about multiple fields.
Yeah, so given like if you're already interested and curious and reading a bunch of things and studying a bunch of things and thinking about these topics, on the margin, there are a bunch of things you can do to make you more productive at having this – of making some contributions to this. And I think just speaking to people and writing your thoughts down and finding like especially useful people to chat with and collaborate with.
I think that's very useful.
So just seek out people that have similar views and you're able to have very high bandwidth conversations with
and seemingly, you know,
and kind of make progress on these topics.
And I think that's just pretty useful.
But how exactly, like, should they DM you?
Like, how do they get it?
Yeah, sure. And like, I don't know, set up signal chats with your friends or whatever.
Yeah, yeah. I've done a lot.
Actually, it's like crazy how much alpha I've gotten out of that. Yeah, I mean, I think, like, in fact, one advice I would give to people in general, even if they are not, like, thinking about AI specifically, but I think it's also helpful for that, is just that people should just be much more aggressive about reaching out.
That's right. Yeah.
Like a lot of the communication that like maybe people have an impression that if you like reach out to someone who looks really important and like they're not going to respond to you. But like if what you send to them is just interesting and like high quality, then it's very, very likely that they will respond.
Yep. Like there's like a lot more edge there that you can get, which is just being more aggressive and less ashamed or something of looking dumb.
That's the main advice I would give. Because if you want to be productive, then again, there are these complementarities and so on.
You need to be part of some community or some organization. And it goes back to the thing about reasoning alone not being that helpful.
Yeah, yeah, yeah.
It's just like other people have thought a long time and have randomly stumbled upon
useful ideas that you can take advantage of.
That's right.
So you should just like try to place yourself in a situation where you can become part of
something larger.
Right.
Which is working on the phone.
That's just a more effective way of contributing.
And to do that, you have to, well, let people know. That's right.
That's right. And I think just coming to the Bay Area is, especially for interested in AI in particular.
Yeah, coming to the Bay Area is nice. Just post, like just writing things and like posting them in a way people can see them.
Just aggressively reaching out to people with like interesting comments. Provided your like thoughts are interesting and so on.
I mean, they probably are. Like in many cases, I think it's like my thoughts weren't, my thoughts still might not be interesting.
But people will tolerate my cold emails and are like, you know, will still like do collaborate with me and so forth. The other thing I've noticed, tell me if this is actually the wrong pattern or the wrong.
Yeah. with people like you or with Carl or something,
is that as compared to a general person
who's intellectually curious or reading widely you tend to focus much more on key pieces of literature than say I'm gonna go read the classics or just generally read like it's like I'm gonna I'm gonna just like put like a ton more credence in something like the Romer paper and a normal person might not even read the normal person who's like intellectually curious would just like not be reading key pieces of literature. Yeah, I think like you have to be very mindful of the fact that you have a very limited amount of time.
Like you're not an AI model. So you have to kind of aggressively prioritize what you're going to spend your time reading.
Even AI models don't prioritize that heavily. They read Reddit mostly or a large part of their corpus is just read.
Yeah, key pieces of empirical literature at least. At least among you guys.
I mean, that might not be the most productive thing in general, but. I think that's useful.
I also just think it's useful to read Twitter. I think we're having this conversation about people often say that they should, like they're spending too much time reading Twitter and they wish they spend more time reading archive.
But actually, like the amount of information per unit time you get reading Twitter is often just much higher. And it's just much more productive for them to read Twitter.
I think there are key pieces of literature that are kind of important. And I think it's useful to figure out what people who have spent a lot of time thinking about this find important in their worldview.
So, you know, in AI, this might be, you know, key papers, like, I don't know, like the Andy Jones paper about scaling laws for Inference is like a big thing. And in economics, like this Romer paper or the paper on explaining long-run population from Kramer or from David Rudman and so on.
I think just like if people who you think are having really good – who think very well about this suggest a certain paper and they like highly recommend it, then I think you should take that seriously and actually read those papers. And for me, it's been especially helpful to, instead of just skimming a bunch of things, just like really stop on, like, yeah, if there's a key piece of literature or for, yeah, in order to, for example, understand the Transformer, I like, there's always a Karpathic lectures, but one resource that was really useful is the Anthropics original transformer circuit paper.
And I just like – just spending a day on that paper instead of skimming it and making a bunch of space repetition cards and so forth was much more useful than just like generally reading widely about AI. Yeah, I think it's just much more important here if you want to prioritize things correctly to be, again, to be part of a community or to be getting input from a community or from people who have thought a lot and have a lot of experience about what is important and what is not.
Like this is true even in academic fields. So if you want to do math research, but you're not part of like a graduate program, you're not at a university where there are tons of people who like do math research all day for many years, then you're not even going to know like what are the open problems that I should be working on? What is reasonable to attack? What is not reasonable to attack? Like what papers in this field are important, contain important techniques? You're just going to have no idea.
Right. So it's very important to be like plugged into that feed of information somehow.
Yeah. But how did you know all this shit before being plugged in? Because you weren't talking to anybody in Ankara.
I mean, you don't need to talk. I mean, the internet is a pretty useful thing in this respect.
And you don't need to necessarily talk to people. Like you can get a lot of benefit from reading.
Like you just need to identify, okay, like who are the people who seem like consistently most interesting. And you can also get a lot of benefit or maybe you found one person.
And then often that person will know some other people who are interesting. Right.
And then you can like start tracing the social network. So for example, maybe, I don't know, like one example I can give, which I think is actually accurate is like maybe you know about Daniel Ellsberg.
So you like look for a podcast where he appears on you notice that he's appeared on 80 000 hours podcast which he has and then you notice like there are some other guests on the 8 000 hours podcast so maybe there's brian kaplan who has also appeared on the podcast and then maybe robin hansen has also appeared on the podcast and then you know maybe there are some people those other people know and then like like just tracing that kind of social network and like figuring out who to listen think that can be... And I think you're doing a very big service to making that possible.
I think your selection is often very good. I'm actually curious if you're offline what I got wrong.
Actually, I think I know the answer to that. So, you know, and I think that makes it a bunch easier to track, like, you know, who are the people doing the most interesting thinking on various topics.
Cool. I think that's a good place to end with you praising me.
No, I'm kidding. Again, I highly recommend people follow Epoch.
There's a great weekly newsletter, Gradient Updates, which, I mean, like people plug newsletters, but this is like, I can't believe this is a thing that comes out on a weekly basis
and it's like
it's like
anyways
and you now have a new podcast
which I will not plug
as a competitor
but you can check it out
it's actually letting your studio
that's very generous
anyways
cool
thanks guys
alright thanks