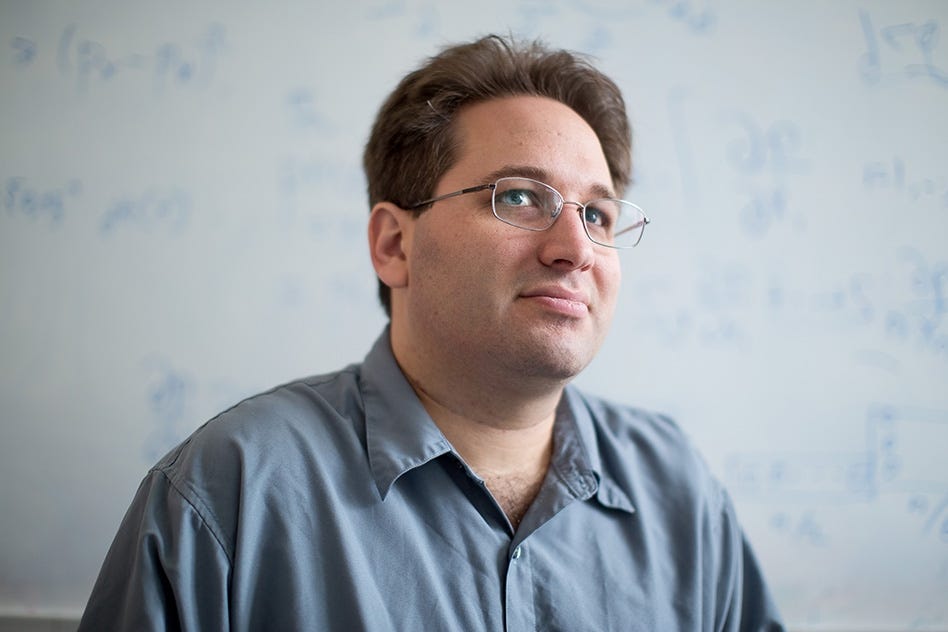
Scott Aaronson - Quantum Computing, Complexity, and Creativity
Scott Aaronson is a Professor of Computer Science at The University of Texas at Austin, and director of its Quantum Information Center.
He's the author of one of the most interesting blogs on the internet: https://www.scottaaronson.com/blog/ and the book “Quantum Computing since Democritus”.
He was also my professor for a class on quantum computing.
Watch on YouTube. Listen on Apple Podcasts, Spotify, or any other podcast platform.
Episode website here.
Follow me on Twitter to get updates on future episodes and guests.
Timestamps
(0:00) - Intro
(0:33) - Journey through high school and college
(12:37) - Early work
(19:15) - Why quantum computing took so long
(33:30) - Contributions from outside academia
(38:18) - Busy beaver function
(53:50) - New quantum algorithms
(1:03:30) - Clusters
(1:06:23) - Complexity and economics
(1:13:26) - Creativity
(1:24:07) - Advice to young people
Get full access to Dwarkesh Podcast at www.dwarkesh.com/subscribe
Listen and Follow Along
Full Transcript
You know, it might take, you know, years or decades to become, you know, an expert, like in a whole field,
you know, and you might be very far from that, but it really doesn't take that long to become
the world expert on one particular tiny little problem, right? And, you know, so try to, you
know, become the world expert on something, you know, even something very, very narrow. So, Professor Aronson, can you tell us a bit about your early journey? You were a young prodigy.
You graduated high school at the age of 15, got your Ph.D. at 22.
Can you tell us about that? Yeah, well, I didn't really graduate high school
when I was 15. I got a GED from New York State.
But I was not happy in high school for several reasons, I mean, socially, academically, and I wanted to get out. And I mean, I was in a weird situation because I went to public school in the U.S.
for junior high, and then in Pennsylvania, actually. And then my parents moved to Hong Kong.
I lived in Hong Kong for one year because my dad was working there. And because of the...
I went to an American international school there, but because of a mismatch between the way they did things in the US and in Hong Kong, you know, I was not able to do math that was appropriate, I guess. I, you know, I had always been sort of ahead in math.
And the only way to deal with that was for me to skip a grade and skip and go to the high school. And once I well, once, once I had done that, that was, that was sort of a, you know, something, something flipped for me, right.
That, you know, I could actually do this. I could get out of this environment, you know, where, where, where I really wanted to be was college, right.
I wanted to be in a, in a, in a, in a place where, and of course, I somewhat had an idealistic view of what college was, but a place where ideas would matter more than popularity and where you would be able to choose what to study and advance at your own pace and, you know, all of these wonderful things. And, you know, so then I returned from Hong Kong to the U.S.
and was in a public high school for a year. And, you know, as I said, I didn't like it.
And, you ran out of math to take. I took the AP calculus.
And then my parents basically suggested to the school, well, why doesn't he just do online learning, right, and do like
differential equations or whatever with the, you know, Stanford has this EPGY program, right, where you can do these things. And, you know, my parents said they would pay for it.
The school said no. that that's you know uh um and uh so i i sort of seized on that as my excuse I I think I just seen a brochure for a place called the Clarkson School in upstate New York which is a part of Clarkson University but you can live there for a year and take college courses, you know, but it's, it's, it's for high school students, right? So I said, you know, I, you know, you know, even knowing very little about this, I think, you know, I want, I want to give this a try.
And my, my parents, you know, allowed me to do that. You know, we had the car all, you know, packed up to drive there.
And while, you know, when we were about to leave, then finally, you know, there was one, you know, actually math teacher at my old high school who was very, very good and who was, you know, trying to advocate for me.
And he was like, okay, guess what?
You know, I just got it so that Scott can take the EPGY program. And we said, too late, sorry.
And so I went to Clarkson, and I, you know, and I generally had a very good experience there. I mean, you know, I mean, I mean, I mean, I mean, socially, there were a lot of the same problems as at high school, but at least I was able to take courses that were a lot more interesting.
I was able to meet professors, get started doing research. And you apply know, the idea is that after a year at this Clarkson program, then you apply to colleges as a freshman, but, you know, but with a year of college credit.
So I did that. You know, I was, you know, just very disappointed at the time at how things turned out because, you know, I got college that I applied to.
I had a very weird background, but I was lucky that Cornell and Carnegie Mellon were kind enough to accept me. And so I decided to go to Cornell, but then there was one problem that they required a high school diploma before you could enroll there, which I didn't have.
And so we realized that I needed a DED from, you know, well, okay, my old high school, you know, oftentimes one's old high school will just give you a diploma after you've gone to Clarkson. My high school would not do that because they said I was missing phys ed.
I would have to spend that summer doing phys ed. And then New York State said, we can't give him a GED because you have to be 17 to have a GED and he's 15.
And my mom eventually convinced them to make an exception and give me a GED. So then I went to Cornell.
I mean, I've met other people who were homeschooled, who actually did things that were more radical than what I did. I mean, I was accelerated by three years.
You know, that was all. And then after I was in college, I didn't really accelerate anymore because, you know, I felt like I was, you know, in an environment, you know, where I wanted to be.
And, you know, from now on, these are the main limitations were internal. They were no longer external.
Was it intimidating being in classes with people three or four years older than you? The truth is, I would say the majority of them didn't even know that I was younger. If they knew, it was maybe an oddity a little bit, but then they didn't care that much.
It's not like I was a 10-year-old. Right.
I was, you know, I mean, by the time I started at Cornell, I was 16 by then.
And. like I was a 10 year old, right? I was, you know, I mean, by the time I started at Cornell, I was 16 by then.
And, you know, I, so I feel like academically, it was fine. I mean, you know, the main issue was, was social, right? I mean, you know, and my, my parents had, you know, had warned had warned me that okay you know to uh skip grades is going to completely screw up my social life and uh you know it's going to make dating you know incredibly difficult and and so on and so on and I I sort of brushed all of that aside you know in my my you know, I mean, you know, of course that all turned out to be true.
You know, I got, I got my PhD before, basically before I, I learned how to drive really, or, or, or learned how to have any kind of a social life, you know, to speak of, I mean, or, you know, any kind of a dating life, really. But, you know, I did things in a weird order, you could say.
And that did cause, you know, an enormous amount of stress for me. But my main argument was that, you know, I was already socially unhappy in high school, right? I was already miserable, you know, without having skipped grades.
And so I felt like, you know, as long as I'm going to be miserable socially anyway, as it seemed at the time, you know, I would be, you know, at least I could be learning stuff. At least the academics could be better.
Yeah. And as far as the academics go, what were the advantages and disadvantages of specializing so early?
Well, I mean, I don't get to rerun my life multiple times and compare. But I think that, you know, mainly I'm grateful that I had the opportunity to, you know, to sort of learn about stuff that interested me.
And it was not it was not just, you know, that I wanted to take only math and CS and nothing else. Right.
It was not like that. I mean, I I took, you know, plenty of humanities in college.
right and uh to have some freedom to pick what to learn about. I mean, like in high school, humanities means the five paragraph essay.
know, you basically have to regurgitate what the teacher wants from you, right? And, you know, if you try to, you know, do your own thing, write things in your own way, then you will actually fail, right? And, you know, this is, I learned this from experience, you know, this is not theoretical, right? And, you know, so theoretical. Even the humanities, I was a lot happier with in college than I was in high school.
It wasn't just a matter of specialization, but partly it was. I think that by the age of 16 or so, I knew what I was passionate about.
It was the fundamentals of computing, right, and understanding what computers could or couldn't do. And I was ready to be learning about that and working on it.
And, you know, I think that there is, you know, the entire concept of, you know, of sort of teenagerhood, right? That like people are, you know, from the age of 12 to 18, or now maybe even 20 or so, are still basically children, right? I think that that's largely a modern construction, right? If you go back even a few hundred years, you know, by the time someone is a teenager, they're an apprentice. They can work.
They can be learning while they're also working. They can be learning the trade that they choose.
and um so so so i think that that that's actually natural right i don't feel like i'm that
unusual in that respect i think you know i mean you know maybe you know unusual in some respects
but but not in wanting to sort of get started with life when, you know, I was 15 or 16. Yeah, I had Tyler Cowen on the podcast and he started, he wanted to be an economics professor basically in his early teens and he was reading and preparing for that from that time on.
Economics professor is not what, you know, one often thinks of as like a child, you know, or an adolescent realizing that they want, but that is, in the case of Tyler Cohen, I could easily believe that. Is there some special advantage of learning the fundamentals of the field you know you're going to go into early on? So instead of, except for the fact that you just get more years to accumulate knowledge,
is there the special advantage of learning it in your early years?
You know, I'm not sure. It's a very interesting question.
I mean, you know, one could imagine that,
you know, while someone's brain is still developing, right, that it's good to be exposed to
certain things at that age. And, you know, I mean, like, we know examples that are like this, right, like, you know, an obvious one would be learning languages, right, where, you know, there's a window where, you know, children can just soak up languages, you know, like a sponge, and, you know, after that window, okay, you know, you can learn a language, but it will be a difficult, you know, intellectual puzzle, and you'll after that window uh okay what you know you can learn a language but it will be
a difficult you know intellectual puzzle and you'll never speak it as well as a as a as a native five-year-old will speak it right uh so um so it it could be like that but it could also be that, you know, like our brains are not really adapted for learning any of this stuff, right? I mean, like, you know, when we get to, you know, theoretical physics, theoretical computer science, and so on, right? You know, all of it is like, you know, learning a language that we don't natively speak, right? And on that model, it would just be a question of getting a head start of, you know, of how much time you have to spend on it. I would love for someone to research that question, because I don't know the answer.
I have heard, well, so as you know, like many of the important discoveries in quantum mechanics were made by people who at the time were very young, right? And that's an interesting fact. Not only did they learn that stuff early on, but they made those contributions early on.
Yeah, but that's not just quantum mechanics. I mean, that's all over math and physics, right? I mean, Newton was about 22 when he had his miracle year of inventing calculus, discovering the laws of mechanics.
I'm already old by the standards of math and physics, which is weird to think about.
But, you know, there are also many examples of, you know, great contributions that were made by people in their 40s, their 50s, their 60s, right? So, you know, so that's another empirical question that I wonder about, right? Is it that people's brains actually slow down as they as they get older?
Or is it simply that they have less motivation or less free time, right? I mean, like, in my case, you know, having two kids, you know, clearly given me enormously less time for research, right? And, you know, like, in those cases where I'm, you know, traveling, where I'm, you know, away from the kids for a week, you know, maybe, like, I can work again, and it suddenly, you know, it feels a lot like it did when I was in my 20s, right, and so, you know, yeah, and then there's also the issue of motivation, right, That like, when I was, before I was established, you know, like my entire conception of myself, you know, my entire like goals for my life were wrapped up and, you know, succeeding in research, you know, and, you know, doing whatever it took. And now, you know, it's more like, you know, I have all kinds of other concerns.
I have my own students to worry about, you know, my postdocs, my kids, of course, my blog, you know, the popular things I write. And, you know, research just feels like one more thing that I do, right, that my identity is not as much wrapped up in.
So, you know, I may have also gotten dumber, right? That's certainly possible, right? But, you know, it's hard to disentangle from those other factors. Yeah, you're the third person, and I promise we'll get to the technical questions eventually, but you're the third person on the podcast I'm about to ask this question because it fascinates me.
Miracle Years, as you mentioned, it's not just that people make very important discoveries at young ages, but they make lots of seemingly uncorrelated important discoveries at young ages. So as you know, Einstein did Brownian motion, special relativity.
What was the third one? There were three more. There was the photoelectric.
Yeah, that's right. And these don't seem, superficially at least, to be related.
And yet it's interesting to happen in the same year. What do you think explains that phenomenon? In the case of Einstein, I mean, you know, you're asking me to explain Einstein's miracle year.
I mean, that's a tall order. I mean, one can say certain things like, you know, one can say that, you know, physics in the early 20th century was ripe for these, you know, for these interrelated revolutions, right, of relativity and quantum mechanics, right? You know, I mean, you know, if it wasn't Einstein, it would have been someone else, you know, not long after with all of those things.
General relativity, which, you know, which took Einstein a decade longer, you know, that was the one thing where if it hadn't been for Einstein, then, you know, for all we know, it might have been decades before anyone else did that. But, you know, the, you know, the stuff that he did in his, in 1905, I mean, you know, they were all things that physics was kind of ripe for, maybe it took, you know, one person just looking at things in a sufficiently different way.
You know, I'm not, I'm not, I'm not sure really, but, but, you know, but, but, but also it might be, you know, the confluence of all of those things is part of why, you know, we think of Einstein as Einstein, right? You know, there were, there were, there were many other great physicists, you know, around the same time, you know, who may have done, you know, one or two things of that caliber, right? great physicists you know around the same time you know who may have done you know one or two things of that caliber right but but you know uh uh but but but but but only only einstein does those three or four of them i'm glad i'm glad you brought up uh the topic of like uh ideas being in the air and ready to plug uh because this is this leads me directly to the next question i was uh rereading the lecture notes from your quantum information science class, and there's the lecture on quantum teleportation. And you were answering, how do people figure this kind of stuff out? And you say, it's worth pointing out that quantum mechanics was discovered in 1926 and that quantum teleportation was only discovered in the 90s.
And of course, quantum computing, I'm a big fan of David Deutsch, and he discovered it, or he thought of it in the 80s. It seems like these ideas were ready to pluck back when quantum mechanics was developed.
Why did it take so long to have these ideas plucked? Yeah, that's an extremely interesting question. I mean, the whole idea of thinking about quantum entanglement, you know, and not as something like metaphysically weird or, you know, spooky or basically how do we explain this away? How do we get rid of this? But in terms of how do we use it, right? How do we use it for, you know,
to improve information processing, right? I think that's a point of view that, as far as I know,
really only started with John Bell in the 1960s, right? With, you know, Bell having this remarkable
insight that, you know, you could do this experiment to test the prediction of entanglement
Thank you. Bell having this remarkable insight that you could do this experiment to test the prediction of entanglement and distinguish it from any possible theory involving local hidden variables.
And then a little bit later, Stephen Wiesner had the idea of quantum money, right?
You're using the uncertainty principle from cryptography. Although he was, again, he was not able to publish that until the 80s.
And there are a few things that I could say here. I mean, one is that when quantum mechanics was discovered in the 20s, it was not at all clear to the people who discovered it that this was sort of a final form that the laws of physics would take.
Right. You know, they thought, you know, I mean, that there was a large contingent that thought that, you know, this is some, you know, scheme that sort of represents our current knowledge.
But, you know, but clearly one, you know, one has to improve on it. Right.
And then that was very much Einstein's point of view. And I think also Schrodinger's.
And then, you know, like Heisenberg, they were very much opposed to looking for something beyond quantum mechanics, but sort of that was sort of, you know, inspiring more research about what you could do with quantum mechanics, right? They just wanted everyone to just sort of shut up and stop, stop asking about these things, right? So you could say, you know, even though Bohr was right, and, and Einstein was wrong on the issue of local hidden variables, you know, there's a, there's a deeper sense in which sense in which, you know, Einstein was the more right one and sort of putting his finger on, you know,
there is something here that we do not yet understand and that we need to understand.
Right. And, you know, indeed, you know, that I'm sorry, you know, indeed, indeed, that thing would
not be understood really until the discovery of the Bell inequality in the 60s. The other
Thank you. You know, indeed, indeed, that that that that thing would not be understood really until until the discovery of the Bell inequality in the 60s.
The other issue is that when quantum mechanics was was discovered into the, you know, the 30s, you know, there was so much to do in terms of, you know, figuring out how chemistry works.
Right. Just applying quantum mechanics to understanding the real world around us, right? And you could say on the other side in computer science, you know, I mean, for God's sakes, the entire notion of a universal computer was just being born, right? The whole, you know, the whole field of classical computing was only just being born, right? So there was sort of so much on the plates of both sides that it might have seemed wildly premature to anyone to combine the two, right? And then of course, World War II intervened, and the people who were doing fundamental science, a lot of them went to work, you know, either at the Manhattan Project or Bletchley Park.
And, you know, and then after that, I mean, I think the theoretical physicists were very focused on just, you know, this zoo of new particles that were being discovered and in formulating quantum field theory, it was very, very much out of fashion for decades to think about the fundamentals of quantum mechanics itself. And in the meantime, people were finally building,, commercializing, figuring out the uses for classical computers.
That was, you know, a very young area itself. And I mean, the entire theory of computational complexity, which, you know, is sort of an intellectual prerequisite to quantum computing, right, that only developed in the 1960s.
Right. You theory of P and NP and NP completeness, that was only the 1970s.
So now, if we think about the people who could have combined these fields, I think of John von Neumann as an obvious possibility because he was, you know, of course, one of the great pioneers of both of computer science and of quantum mechanics. In fact, you know, he invented the notion of entropy of quantum states.
And but, you know, he just he had a lot of other things on his plate. And then he died early.
He died in the 50s. Alan Turing was also, of course, a founder of computer science, who was also passionately interested in the foundations of quantum mechanics.
As we know from his letters to his friends, he died when he was 42. Whatever the case, I feel like quantum mechanics and the theory of computing were both in place by the 1930s, but there was a lot of other stuff on people's plates.
The, you know, the, the, the idea of, you know, thinking of entanglement as a resource that only starts in the sixties, computational complexity that only starts in the sixties and seventies. And then, you know, maybe a decade after that, people start thinking about quantum computation.
Interesting. I've heard two other theories and I want to see how, what you think of them.
So the first one is, think, I might be misquoting him, but I think at some point David Deutsch said the reason he was able to think seriously about quantum computing was that he took the manual world's interpretation seriously, and because people before him hadn't taken manual world seriously, they hadn't been able to go quantum computing. in a second sorry go ahead go on go on okay maybe maybe maybe should i respond to that one yeah yeah
okay so so that is definitely true for Deutsch, right? And I know, you know, Deutsch, well, you know, and he actually, you know, became a big believer in the many worlds interpretation when he was here at UT Austin, right, as a student, as you know, and he, he heard Hugh Everett himself give a lecture about it. Bryce DeWitt, who was one of the main early proponents of the many worlds interpretation was also here at UT Austin and had a big role, had a big influence on Deutsch.
And Deutsch was thinking about how would you sort of make, you know, sort of shake people? How would you sort of make them realize that quantum mechanics is universally valid, that it applies at all scales? Well, you know, if you could build a computer, right, that, you know, could do a superposition over different computations, and then you could see the results of interference between them. At that point, conceptually, it is almost like making a superposition over a brain that can think different thoughts.
And then if you could have that, then the whole idea that observers collapse the wave function just by being observers is no longer tenable. So one problem is that Richard Feynman had the idea of quantum computing around the same time as Deutsch did, right? And Feynman, I mean, he was certainly aware of the many worlds interpretation.
He was aware of effort, but that was not his motivation for thinking about quantum computing. He was, you know, as usual, he was much more practically focused.
He was thinking about how do we simulate physics? You know, how do we simulate nature with a computer? And if we use classical computers, we suffer this exponential slowdown, right? And so can we build a new kind of computer that will solve that problem and give us a universal quantum simulator? You know, people were, there was also a whole movement in the 70s and 80s to think about the physics of computation, including like the thermodynamics of computation. Can you make computation inherently reversible, you know, and all kinds of other issues, right, that are not about, you know, exponential speed ups.
But that was also one of the intellectual streams that sort of led directly to quantum computation, right? So I think that what you said is very much true for Deutsch,
but there were others besides Deutsch
who were thinking about these things.
Feynman was only one, there were others as well.
I think Benioff, several others.
And few people in this field are as messianic as Deutsche's about the many worlds interpretation. It's interesting that, come to think of it, that both Deutsche and Turing were trying to solve a separate philosophical problem when they came up with their model of computation, at least in part.
Okay, so the second theory I've heard is that since the 1970s, that academia has been less open to new ideas. And you mentioned Weisner, Everett, and both of them, I understand, were kind of, I don't know, looked down, ostracized or something.
Is that theory confirmed? I mean, it is possible that in some parts of academia, you know, it has become harder to explore new ideas, you know, than it once was. You know, I could believe that about the social sciences.
I could believe that, you know, about parts of medicine. You know, people used to do just completely crazy things, you know, invent some new concoction, inject themselves with it, you know, see what happens, you know, write an article about it.
You know, whereas today, you know, it might take like a billion dollars of investment before you could even get to the point where, you know, anyone would consider
it remotely ethical to do such a thing, right? So on the other hand, in physics and, let's say, speculation about
the
foundations of
computing
and you know, speculation about, you know, the, you know, foundations of computing and cosmology and things like that. I think if anything, things have gone in the opposite direction.
And, you know, and this is partly because we now have this preprint server, this archive, where, you know, everyone can post, you know, all of their new research ideas, you know, with no filter, right? You know, and I think that that is, that is somewhat transformed the scientific landscape. You know, because, I mean, we still have journals,
but journals are now just like a final stamp of approval
that in many fields is, yeah, I mean,
it's important when you're looking for jobs
or things like that,
but journals are no longer gatekeepers
that can sort of prevent people from seeing your paper, right?
And so, if you just look at the quantum physics archive every day, you will see so many far out ideas. It is so much crazy stuff that it's very hard to believe that a modern day Wiesner or Everett would feel any barrier barrier to, you know, it's very hard to believe that a modern day Wiesner or Everett, you know, would feel any barrier to, you know, to getting their idea out there, right? I mean, today, the problem is more like there are so many, you know, bold new ideas that, you know, of course, you know, most of them won't go anywhere, right? Most of them will fail, right? And, you know, and so there's so much to sift through if you're, you know, looking for what is actually going to be revolutionary.
I'm sure your comments section and email fills up with these. But have you, how many of the important ideas, or do many important ideas in the field come from people outside academia? Or is it mostly people with PhDs working within
the system? Well, okay. I mean, those are not exhaustive categories, right? Because, you know,
there have been breakthroughs that have come from people who were not in academia.
Very often, what you find is that these are people who were sort of on the margin of academia. Like they got a PhD and then they left the academic world or they did part of a PhD or things like that.
There was a famous case of Yi Tang Zhang,
who was this mathematician from China, right?
Who proved that there are infinitely many pairs of primes
at most 70 million apart, right?
Which was a major advance in number theory, right?
And this was a guy who would like,
he had gotten a PhD in math in China, I think,
but then moved to the US and then worked making sandwiches at Publix in order to support his family and work various other jobs, but continued working on math. So I don't, you know, I hear all the time from people who are complete autodidact, who taught themselves the S and that they think that they've solved the P versus envy problem or whatever, right? I've, you know, that is, you know, that is usually someone who just doesn't understand the question, who has just made some, you know, and then, you know, I mean, there is a distinction because sometimes people, you know, who sort of teach themselves the field, they just, you know, they really want to learn.
They just want an expert to talk to, you know, who will tell them, like, here's where you're on the right track. Here's where, you know, you made a mistake.
And they will thank you for that, right? Other times you get people who really dig in their heels and, you know, you know, the establishment is is censoring me. And, you know, I had when I taught at MIT, I had, you know, someone like writing to the president of MIT to try to get me fired.
You know, I had another one sending me death threats, you know, because, you know, very,, very specific, you know, I had to actually contact the police about them, you know, because I would not publish their, you know, proof of P equals NP or their refutation of quantum computing on my blog, right? So you get, you get, you get, you get the whole spectrum. But, you know, the, the, the other thing you find is there are, and a lot of the readership of my blog comes from people who studied technical subjects in college, studied CS or math or physics, and then went out into industry, right? But maintain this connection, you know, maintain their sort of curiosity about fundamental questions.
And some of those people, you know, actually want to continue to do research. And I'm actually co-authoring a paper with one of them right now.
You know, I posted this survey article about the busy beaver function on my blog recently. And there was a, I guess, a hobbyist who solved some of the open problems.
Really? A survey. And yeah, and had a lot of new ideas.
And he and I are going to write a paper about it now. Oh, cool.
Interesting. But by the way, is that Chuang the same one that co-authored the Nielsen textbook on quantum information? No, no, no, no.
There's a Yi Tang Zhang and then there's the mathematician and then Isaac Chuang is the physicist who co-authored the Nielsen Chuang textbook. Okay, got it.
So let me ask you about Busy Beaver. I was, is the
proposition three was that you can't... I have no memory for the numbering of propositions.
Okay. Can you actually just explain what the Busy Beaver function is before I ask you this question? Yes.
Okay, sure. So the Busy Beaver function is a really, really remarkable sequence of hyper rapidly growing integers.
And the way that we define it, it was invented in 1962 by a mathematician named Tibor Rado. And basically what we do is what we would like to say, let me start with what we'd like to say.
We'd like to say, you know, if I want to name the biggest number that I could possibly think to define, then why not just say, you know, the biggest number that can be named using a thousand words or fewer. Okay.
Or something like that, right, but then I have to be careful, because there's an inherent paradox there, right, which is, I also could have said, like, one plus the biggest number that can be named with a thousand words or fewer, but then that, you know, I just named that with fewer than a thousand words, And so from this, the conclusion that logicians, philosophers drew more than a hundred years ago is that the concept of naming a number in English is not as clear as we think it is. It leads to paradox if you're not careful about it.
But what if we could name a number in a way that was completely unambiguous? Well, since Alan Turing in the 1930s, we've had such a way. That way is computer programs or Turing machines.
And so we could say, think of the largest integer that can be generated by a computer program that is at most, let's say, a thousand bits in length. Okay, and, you know, now you have to be careful.
What do you mean by that, right? Because, of course, a computer program could run forever, right, it could start just, you know, I could, we could easily write a program that says do print, not print nine loop, right, and we just print an infinite sequence of nines. So what we do is we restrict attention to those computer programs that eventually halt, right.
We say among, let's say for for a given end, we consider all of the possible computer programs that eventually halt. We say among, let's say for a given n, we consider all of the possible computer programs that are n bits long.
Let's say there are two to the n power of them, or at most two to the n power. Many strings will just lead to things that are not even programs, you know, they won't even compile, so we'll throw those away, right? Now, among all of the valid NBIT programs, some of them run forever.
We just run them on a blank input. So we throw those away as well, right? We consider only the ones that eventually stop.
And now among all of the ones that stop, we take the one that runs for the longest number of steps, the largest number of steps until it stops. And that number of steps, that is what we call the nth busy beaver number.
So the way that Rado defined this was using Turing machine, which is just one particular programming language, the one invented by Alan Turing in the 1930s, he says busy beaver of n is the largest finite number of steps that any n-state Turing machine can run for. And so the amazing thing is one can prove that this function grows faster than any computable function.
So no matter what sequence of integers, you can... Basically, if you are in a contest to name the largest number, and if you say busy beaver of a thousand, then you will utterly destroy any opponent who doesn't know about the busy beaver function or about anything similar to it.
So one can say further remarkable things about this function, like that only a finite number of values of the function can actually be proven from the axioms of set theory, right, for reasons of Gödel's incompleteness theorem, basically, after a certain finite point, you know, the values of this function, you know, we can, you know, presumably it has definite values, right? Because it's a, this clearly defined function and yet we could, we could no longer prove what they are. Okay.
So, so right now only, you know, if you, if you look, if you take the busy beaver function as Rado defined it in the sixties, only four values of the function are known. Okay.
That busy beaver of one is one busy be beaver of two is six, busy beaver of three is 21, and busy beaver of four is 107. Busy beaver of five, it is only known that it's at least 47 million, okay? You know, and we don't know how much bigger it might be.
Busy beaver of six, it's at least, I think, about 10 to the 36,000, okay, might be much bigger. Busy Beaver of seven, it's at least 10 to the 10 to the 10 to the 10 to the 18 million, you know, and might be enormously larger still, okay, just to give people an idea of how this function grows.
Yeah, yeah, and by the way, I recommend everybody who's listening to check out the Busy Beaver Frontier paper because it was written in such a way that I also want to ask you how you learned to write so well. It was written in such a way that non-expert like me could understand it.
And just to clarify from my own understanding, it's not that busy, there isn't a function that for any N is greater than Busy Beaver of. It's just that it won't, busy beaver will eventually be with more states than if you have to.
That's exactly what I meant when I said grows faster than, right? I mean, each particular value of busy beaver is just some positive integer, right? It's a very concrete thing, right? You know, like it's six or it's 21, right? But, you know, if you look at the rate of growth of these integers, right, it will dominate, eventually dominate any computable function. Right.
And in practice, not just eventually, but very, very quickly. Yeah.
And you show very elegantly in the paper that that means you can independently prove the halting problem and Gödel's incompleteness theorem. Yeah.
Yeah. I'm looking at, I mean, I mean, I mean, like the, the, the unsolvability of the halting problem, Gödel's theorem, like these things are so intertwined, like there are many, many different ways, you know, ways to prove them all.
Right. But the, the Busy Beaver function gives you one way that, yeah, you can prove that, you know, that you can use it to prove independently that there is an uncomputable function.
You can use it to prove Gertl's incompleteness theory. Right, okay.
So my question, the proposition three was the one that said for any axiomatic theory, you can't approve all of BusyViewer with it. Right.
So my question was, can you keep, even though there's no systematic way to extend our set theory, is there plausibly a way that you can keep extending it such that you can prove higher and higher values of BusyViewaver? That's a wonderful question. I would say we don't really know yet, right? Because right now we can't even pin down Busy Beaver of five, okay? Now, my guess would be that the resources of existing set theory are perfectly enough to do that.
But we don't even know that. So four years ago, a student of mine named Adam Yedidia and I decided to look into a question that for some reason no one had looked at before, which was, you know, like, what is the smallest n for which we can actually prove that the value of busy beaver of n is independent of set theory, right? Like, it was clear that there is some n for which this is true, but are we talking about 10 million or are we talking about 10, right?
So in practice, what this problem boils down to is almost like software engineering.
Like you have to construct a Turing machine
that checks all of the theorems of set theory
and that halts only if it finds a contradiction, right? And you have to build such a Turing machine with as few states as possible. You can build such a machine with only n states, then you've proven that set theory cannot determine the value of busy beaver event.
Because if it did, then it would thereby determine its own consistency, which is exactly what Gödel's theorem does not allow, right? So what we managed to do is we managed to find such a machine with 8,000 states, okay, about 8,000 states. You know, that was after a lot of optimization, right? And, you know, a lot of coding and engineering and ideas.
Right. Since then, again, a hobbyist to come back to your earlier question, someone outside academia by the name of Stefan Orier has managed to improve our balance and got it to under 800 states.
Yeah. Okay.
And that, that is the current record. Now, if you could get that down to like, you know, you know, I, you know, it is a wonderful question.
Could you get it down to like 10 states or something like that? Right. And, you know, that, that would tell us that we have to already go beyond the current axioms of set theory, you know, even to just get the next few values of the busy beaver function, right? But maybe not.
Maybe you can even get a hundred of them with existing set theory, right? We don't know. Now, what you can do is, you know, at whatever point, ZF-Zermalofrankel set theory, which is the accepted basis for most of math, at whatever point it runs out of steam, we don't know exactly where that point is, but one can then extend it by what are called large cardinal axioms, which basically assert that there exists an infinity that is bigger than any infinity that can be defined in ZF set theory, right, or, you know, you can, you can say, you know, you know, infinity is of various particular kinds, right, and, you know, and presumably one could then settle more values of the busy beaver function that way, right? But the issue is, you know, no matter what set theory you think of, right, as soon as you can build a Turing machine that enumerates all of the theorems of that set theory, then however many states there are in that Turing machine, that then sets a bound on how many busy beaver numbers that theory can ever determine.
So in some sense, the set theories that can determine more and more busy beaver numbers will have to become more and more complicated. We know that.
And as you said, there will never be a systematic way to search for them, because if there were, then that would make the busy beaver function computable. And, you know, and what it means for there to be no systematic way to search is that, you know, you could we could keep like proposing more and more set theories.
If you look at modern mathematical logics, theorists do this.
They do propose more and more set theories. And if you look at modern, you know, mathematical logic, set theorists do this, right? They do propose more and more large cardinal axioms, but sometimes they actually discover that their axioms are inconsistent, right? Or, you know, they sort of provisionally, you know, adopt an axiom or use it, but the community is not really convinced that it won't lead to an inconsistency, right? So that, you know, there's no surefire way to think of these axioms and be confident that, you know, that you actually still have a consistent system.
Okay, let me offer a very, very silly thought experiment. Yeah.
Okay, so this is inspired by that joke that if you shoot enough sunlight at the Earth, it'll shoot a Tesla back, right? Okay, so the idea is if the Turing principle is true, you can simulate the Earth and the solar system and everything on a very incomprehensibly big computer, right, with all the humans on it. Given the appropriate initial data.
Yeah, yeah. And so simulating all of that is a computable function.
And if you can check in every 100 years and see what is the biggest busy beaver number that humans have proven this century, what is the biggest busy number prove 100 centuries, is that not a computable function where that tells you busy
beaver of N indefinitely higher? Well, it would give you a way to compute arbitrary values of the busy beaver function if humans were indeed to continue, you know, you could say under the assumptions that number one, you know, the, you know, you know, everything in our physical world is computable. I mean, you know, we, we, we, we let that, you and I just let that assumption pass almost without comment, right? Although, you know, of course, that's an enormous question in itself, right? With some brilliant people like, like Penrose on the, on the other side of that,.
But all right. But, you know, and then just so assuming that, you know, everything we're doing is computable and also assuming that we could somehow, you know, continue finding more and more values of the busy beaver function indefinitely, right? If that were true, then we would have a contradiction.
Yeah. Yeah.
Yeah. So then either the Turing principle is false or we can't indefinitely keep extending.
Yeah. Yeah.
You could say a very conservative layout would be to say, well, our quest to compute more and more busy beaver numbers will come to an end. Right.
Okay. That's very interesting.
In fact, there has not been another Busy Beaver number determined since the early 1980s. Yeah.
That's interesting. Which is when Busy Beaver 4 was pinned down.
Okay. Okay.
So I'm looking forward to the paper you published with the hobbyists. Yeah.
Bruce Smith is his name. Bruce Smith.
Okay, very interesting. Okay, so let me ask you now, I remember in class last year, you said, you know, that basically, we have a very, a few very important quantum algorithms like Grover's and Shor's that are discovered in the 90s.
And now a lot of stuff now is just an extension of those algorithms. What do you think is the potential of finding, is there a good reason to think that there are other quantum algorithms to be discovered that are as fundamental and important as Grover's insurers were? I would, I would love it if there were, right.
I I'd be, I'd be thrilled to, you know, discover such an algorithm, have one of my students discover it. Right.
You know, this is, this is, you know, well, you know, I mean, you know, I mean, that's the kind of discovery that we enter this field for, right? I mean, you know, now, you know, if we're being intellectually honest, you know, we have to admit that, you know, it's been 25 years since Grover's algorithm was discovered, right?
And, you know, maybe no other quantum algorithm as fundamental as Shor's or Grover's has been discovered, you know, in the last 25 years. What we have discovered, as you learned, because you took my class, was an enormous number of generalizations and new applications and variations of Shor's and Grover's algorithms, including what are called quantum walk algorithms, including phase estimation- algorithms.
And some totally different quantum algorithms were also discovered, although the problems they solve are maybe more abstruse. It's harder to explain what problem they're solving.
And, you know, so, you know, you could wonder, you know, is it lack of imagination on our part? Or is it, you know, I mean, another possibility is, you know, if you look at the history of classical computer science, you know,
what you find is that there are a few basic techniques that were discovered very early on in the history of classical CS. One of them is dynamic programming, like, you know, dividing, you know, breaking down your problem, like recursively into sub problems, right? I mean, you know in general recursion right so you know divide and conquer uh greedy algorithms uh you know uh uh convex programming you know linear programming uh you know um um gaussian elimination right uh and you know and and and most you know, I mean, the field of classical algorithms is enormous.
And yet, you know, most of the classical algorithms that we know are somehow built up out of these motifs that are just that were discovered very early on, right? And we don't normally think of that as a failure of classical algorithms, right? We just think of it as, you know, there are these fundamental features of the algorithmic universe that, you know, that people noticed as soon as they started looking, right? And then, you know, and then, you know, you can go much further, but you go much further by building on the basic things that you have, right? And so maybe we should think of Shor's algorithm and Grover's algorithm, not as just specific algorithms, but as sort of some of the basic design motifs of the world of quantum algorithms. And then, you know, it's not surprising that they were discovered very early on, just like dynamic programming was discovered, you know, right at the beginning of the history of classical algorithms.
So, you know, so, you know, I mean, that's one point of view. Now, another point of view is, you know, if, like, when people ask for more quantum algorithms, or, you know, they hold us to account for our failure to discover
more quantum algorithms. You know, I like to answer that question with another question,
which is, well, what are the problems that you would like these algorithms for, right? And amazingly, that question almost always stops, you know, they ask it, right? Because like they didn't didn't even, you know, well, because no matter what problem they name, you know, there's an excellent chance that people in quantum algorithms have thought about it, you know, and we know, you know, something about how much speed up you can get from a Grover-type algorithm, you know, but we have good reasons to think that you're not going to be able to get better than that. Or, you know, I mean, or, you know, we, or, or we say, well, maybe there's like, if, in the case of the graph isomorphism problem, yeah, sure, maybe there's a polynomial time quantum algorithm, but probably just because there's a polynomial time classical algorithm, right? And that, you know, and it's just, it's that that hasn't been discovered yet, Right.
Although, you know, there's been major progress toward it. So no matter what problem they name, I mean, probably someone has studied it in the context of quantum algorithms.
And I could then tell them for that problem exactly what is the current situation and what are people stuck on? And so it might be that if we want to discover fundamentally new quantum algorithms, that the way to do it will be to realize fundamentally new problems, right? Problems that people hadn't even thought about designing an algorithm for previously, right way that I like to put it is in any given area of math or science, there is this phenomenon of low-hanging fruit that gets picked very, very early on. And then those of us who come into the field a little bit later have to leap higher, you know, if we want to find any fruit, right? But the, you know, I think the ultimate solution to the problem of low-hanging fruit being picked is to find a new orchard, you know? And so, you know, figure out what are, you know, potential problems that quantum computers could solve that no one has been thinking about before.
You know, maybe people actually starting to get quantum computers, as they finally are today, that they can experiment with, will help stimulate the discovery of those new or new applications. Just like with Grover's algorithm, is there some reason to expect that from first principles, there are other algorithms that can be solved by quantum algorithms? Yeah.
So it is, you know, anything where it's sort of as basic as Grover's algorithm that you just look at, you know, the way that amplitudes are changing over time and think that an algorithm is going to exist. Right.
That probably would have been snapped up by now just because quantum algorithms have become so much more sophisticated compared to what they were 25 years ago. But there are some problems where there is some evidence that a quantum algorithm might exist, even though we don't yet know it, if it does exist.
A good example is computing the edit distance between two strings, which means the minimum number of insertions and deletions and changes that I have to make to change one string to another string. This is a fundamental problem for a DNA sequence alignment, for example.
The best known algorithm for it takes quadratic time based on dynamic programming, in fact. And, you know, there is some evidence that there might be a quantum algorithm that would take n to the three halves time or something like that.
But if so, it has not yet been discovered. So there are cases like that.
Um, um, no. Gotcha.
Okay.
The next question I wanted to ask you was, why do many of the important discoveries, not just in this field, but in many other fields come from closely communicating groups of collaborators or people within such groups? You said on Sean Carroll's podcast that Shore and Grover were collaborators about labs. I'm not sure that they actually were collaborators.
I mean, they worked in the same building, I believe. I think the discovery of Shore's algorithm created a lot of excitement, well, all over the place, but certainly at Bell Labs, which is where Shore was.
right and I think that think that Grover, who worked also at Bell Labs, but in a completely different department, I think he was affected by that excitement. And, you know, I'm not even sure if they knew each other prior to Grover's discovery of Grover's house.
But could ask them that. But more broadly, it is certainly true that in the history of ideas, major innovations seem to come in clusters all the time.
Bell Labs was a huge example of that, right? I mean, you know, the Shores and Grover's algorithms were really, really at the tail end, right, of, you know, the heyday of Bell Labs, right, which was mostly the, you know, the 40s, 50s, 60s, 70s, right? But I mean, you had the invention of the transistor, of the communication satellite, and so many other things, right? From this one place. You know, another example would be, you know, I mean, you know, we could take Athens and the ancient world.
Right. We could take Florence.
We could we could look at Cambridge University.
Right. Right.
Let's say, you know, the turn of the 20th century.
Right. That had just so many mathematicians, economists, philosophers, physicists who revolutionized the world.
Now, you know, this might be because, you know, something about the environment, right, that, you know, that, you know, ideas bounce off of each other, right? People see something, they see someone achieve something spectacular, and they're either, you know, inspired by that, or they view it as a challenge, you know, they want to compete against that, and come up with their own thing, right? You know, a Silicon Valley, of course, you know, would be another big example, although more for technology than for science, right? So that would be one explanation. And a different explanation would be that, you know, these certain places at certain points in time just, you know, attract all of the people who, you know, maybe anyway would have had these great ideas, but, you know, that kind of person wants to go to these, you know, these centers, you know, wherever they are.
And so, so, so, so, so, so these centers will just collect the kind of people who are likely to discover these things. Right.
Correlation doesn't equal causation in this case. Okay.
All right. Let me ask you now, I've interviewed a lot of economists on this podcast.
I think this question will be interesting to the listeners. In your paper on why philosophers should care about complexity, you talk about how the difficulty in finding Nash equilibrium might be relevant to discussions on economics.
Can you explain this? Yeah. Okay.
So there was a big advance in theoretical computer science 14 years ago when theoretical evidence was finally discovered for why computing a Nash equilibrium is a hard problem, basically. And this confirmed a suspicion that people had had for a long time.
If we look at a von Neumann equilibrium, which is like an equilibrium of a two-player zero-sum game, then this can be found easily, you know, using linear programming, right? But a Nash equilibrium is somehow a more complicated beast, right? And it's, you know, the way that Nash proved that they exist in the 50s was using the, what's called the Kakutani fixed point fixed point theorem. It's some fixed point theorem from topology.
And if you try to actually unwind the existence proof into an actual algorithm to calculate the equilibrium, then what you get is an algorithm that ends up taking exponential time. It eventually hits the equilibrium, but it may have to follow an exponentially long trail before it reaches it.
If you're interested in this, by far the best things that have been written about it, I think, are by Christos Papadimitriou. And Papadimitriou was one of the discoverers in 2006, along with Goldberg and Das Kalakis, of this hardness theorem, which, you know, it doesn't prove that it's hard to find a Nash equilibrium.
In fact, it doesn't even prove that it's NP-hard. This problem kind of doesn't have the right structure to be an NP-complete problem just
because of Nash's theorem that tells us that a Nash equilibrium always exists, right? Like in order to be NP-complete in any way that we currently understand, there has to be a decision problem. You know, is there a solution or is there not a solution, right? But for finding a Nash equilibrium, there always is a solution.
There's only the problem of how to find it. But what was shown is that basically finding a Nash equilibrium is at least as hard as any other problem for which a solution is guaranteed to exist because of the same kinds of principles.
Okay. So it is complete for that complexity class of problems for which a solution is guaranteed to exist for this sort of reason.
So what does this mean for economics? Well, it's not clear, right, if it has a sort of direct implication, but it
sort of fits into this general narrative of just because an equilibrium exists, that's not the end of the story. I mean, if the market can't actually find the equilibrium, we know, if calculating this equilibrium would take exponential time, then we shouldn't expect the market to be able to find it either, right, and so, you know, now economists are well aware, right, that there are these issues, right, that, you know, people are not perfectly rational, you know, even if they want to be perfectly rational, which they don't always, you know, being perfectly rational might involve computations that they're just not able to do, right? And, you know, and they've, you know, with varying degrees of success, you know, they've tried to account for such phenomena.
But, you know, I would say, you know, the, you know, Nash equilibria are so central to economic theory, right, that, you know, the hardness of finding Nash equilibria, I think, you know, is a, you know, maybe a non-trivial result, you know, underscoring that general point. that that's interesting.
Do you think Hayek's knowledge problem, or the way he phrased it, might be related to complexity as well? So in terms of like central planning, in order to satisfy some constraints said by bureaucrats might be like an NP-complete problem where it's like, you know, I want to separate two different things. One is lack of knowledge about what is going on in the economy.
And the other one is lack of ability to do computations on the knowledge that you have. right so so you know the the the hardness of Nash equilibria is talking about the latter issue
yeah I see what I'm saying you know they're you know, they're related in a way, right? They're both, you know, they're both different kinds of deviations from perfect omniscience, right? But there are different kinds of deviations from omniscience. And, you know, in theoretical computer science, you know, very, very often we have to distinguish them.
So, you know, I mean, often, like, people will ask me if some problem is, you know, is or isn't NP complete when, you know, what they really mean is, like, how hard is it to collect the information, right, which is, you know, it's kind of like apples and oranges. It's a category mistake, right? Once, you know, it's like to even talk about whether a problem is in P or is an NP or whatever, we assume that an input is given to you, right? So all of the information that you need, you know, you have it in front of you.
And then, you know, we are exclusively concerned with the difficulty of calculating something about that information, right? Now, there is also, you know, the difficulty that, you know, people who are in, you know, economic actors, you know, don't have the information that they need, or certainly central planners, you know, don't have the information that they need, right? And there's actually, there actually is a whole subfield of economics, you know, that the economics of information, right? How much do you pay to learn something about, you know, what is going on? Or how do you hold a, how do you design an auction in a way that you elicit the information that you want from the participants in the auction and things like that. I think that economists maybe have an easier time dealing with those things, or that stuff has been better integrated into economics than the computational considerations.
Okay, that's incredibly interesting. There's a few more.
Yeah, I'm going to bring us back to David Deutsch and creativity. Okay.
In the Ask Me Anything chapter of quantum computing since Democritus, you have a student ask you what complexity class is creativity in, and you say, part of what you say is, we've got a billion years of natural selection giving us a very good toolbox of heuristics of solving certain kinds of search problems like problems in np um but that makes it kind of sound like we have more heuristics to solve these problems than chimpanzees do chimpanzees have more than ants uh i don't know if this is how you meant it but do you see like the algorithm for creativity as a thing you have or you don't have?
Or is it like you just have better heuristics for searching through different places?
I don't know that there is such a thing as the algorithm for creativity, right?
In fact, you know, the phrase is almost oxymoronic, right?
That if there were such an algorithm, well, then whatever it output would no longer be creative, would it? Because it would just be the output of that algorithm, right?
So, you know, I think that, you know, it seems like there is such a thing as, you know, general
purpose reasoning ability or general purpose ability to invent creative solutions to problems,
Thank you. a thing as general purpose reasoning ability or general purpose ability to invent creative solutions to problems, which let's say Einstein had more of than some random person off the street, but the random person off the street has more of than a chimpanzee, and a chimpanzee has more of than an ant.
But it is somehow very, very hard to articulate what we mean by that, you know, in a way that would actually support these, you know, comparisons across, you know, vastly different evolutionary histories and goals in life and all these things. Do you, from the beginning of infinity, do you buy David Deutsch's term a universal explainer, that people are universal explainers, AIs will be universal explainers, but non-human animals aren't, and that's like the only demarcation that matters? Yeah, I think Deutsch is like, he's like incredibly optimistic and also incredibly categorical in his thinking, right? You know,
I don't know anyone else who was sort of as optimistic or, you know, and hardly anyone else who was as black and white, right? I mean, I, you know, it does seem likely that there is some kind of threshold that you cross in going from a chimpanzee to a human, right?
Where like, yes, you know, a chimpanzee is smarter than a cow, right? But like, you know, if you stare at both of them, you know, it doesn't seem like, you know, the chimpanzee is noticeably closer than the cow is to, you know, being able to land on the moon, right, or, or, or, or proof from Oz Last Theorem, right, or any of these things, right, and, you know, with, with humans, you know, you, you had, like, in, in, in succession, you had, you know, a few, you know, extremely important milestones that, you know, that had not been crossed before in the animal kingdom, right? You have universality of language, right? You know, I mean, the ability to have a recursive language that can sort of, you know, express thoughts of, you know, unbounded complexity. You had, you know, the invention of writing, you know, the ability to transmit those thoughts across generations, you know, the, you know, a number system that could refer to arbitrarily large numbers, you know, and then, you know, computers, you know, which are, which are universal machines, right, the ability to build these kinds of machines, and, you know, and all of this went along with, you know, being able to explain the world around us in, you know, in, you know, in explicit theories, to an extent that no animal species, no other animal species was ever able to do.
Having said that, I don't actually know if people are universal explainers. That is, you know, I have, you know, I have no idea if we can explain everything, or even if we can explain everything that is explainable.
You know, I, of course, I hope that we will continue being able to explain a lot more than we can explain right now. But I mean, Deutsch uses words in unusual ways.
Like when he talks about why he is so optimistic, part of his optimism is when he uses the word people, he also includes extraterrestrials. right? So he does like, oh, yeah, you know, it's possible that humans on earth will just all kill themselves out, you know, there will be a nuclear war or an environmental catastrophe, but that's not a big deal, because people in the broader sense of, you know, life elsewhere in the universe will go and do all the amazing amazing things anyway that we would have done.
I mean,
that may be called comfort to most of us here on earth, right? And so when he says something like people are universal explainers, you know, you always have to press him on, you know, not only what does he mean by a universal explainer, but even what does he mean by people? right uh his claim on the universal explainer part is that just as many worlds is the most parsimonious way to describe quantum mechanics so you don't have to like postulate an arbitrary, you know, collapse. Since we have no reason to expect there's things, since we have no proof that there are things we cannot explain, the most farcimonious explanation is that we can explain everything.
Okay, I don't know. I mean, you know, there are certain questions like the hard problem of consciousness, let's say, or the question of why is there a universe at all? where, you know, it's not just that we don't have an explanation.
It's that the mind sort of spins in circles when we try to contemplate what could possibly consist of an explanation, right? What, what, what, what, what could an explanation possibly look like even in principle, right? No, that, that, that, that, that could just be a lack of imagination, right? But, you know, it could be that there are, you know, I mean, like we all know, you know, the two-year-old who just, you know, asks why and you tell them and they ask why. And you tell them and, you know, they ask why.
And, you know, after, you know, a half dozen whys, you know, you're all the way back at the Big Bang, right? you know you're you're back at uh uh you know, a half dozen why's, you know, you're all the way back at the Big Bang, right? You know, you're back at, you know, the beginning of the universe, and, you know, they continue asking why, right? And it could be that, you know, there are questions with the property that every...
So, okay. I mean, first of all, even Deutsch thinks that we will not...
That there is no one time where we'll have an explanation of everything. right because because Deutsch you know says, that each, each, each question that we answer will lead to further questions, right? You know, each, each time you explain something, you know, there's, there's then the question of, you know, whatever the explanation is based on, you know, why is that, right? So just like that two-year-old, right, we can always dig deeper and deeper.
Okay, but now, you know, just now, just to loop back to earlier in this conversation, if we think about the busy beaver function, we know that it's not just that you need more and more resources to compute more and more values of the busy beaver function. And so you'll never know all of them.
It's that there are fixed values, like busy beaver of 800, right, where the existing axioms of set theory, you know, provably will not suffice to let you determine that, right. And so likewise, for all I know, there could be fixed questions where maybe the hard problem of consciousness, maybe why is there a universe where what we currently consider to be an explanation just will not suffice to ever explain these things.
But I don't know. I unlike Deutsch, you know, I don't want to assert that I know the answer from first principles.
I, you know, I want to continue looking for explanations of these things. You know, it can be, when you're searching for explanations, you know, it can be psychologically helpful to, you know, assume that the explanation exists.
But let's not make that into more than it is, right? Let's not take a useful heuristic and elevate it into a basic principle of reality. Right.
But on this point, Deutsch wrote a book in The Fabric of Reality, where he talks about how Godel's incomplete initiative actually verifies the importance of creativity. So that if we need to come up with new axioms to prove a busy viewer of 800, that seems to be a point of creativity.
And as far as like, I think he thinks the hard problem of consciousness can be solved. But even if it can't be solved, like the reason it's so hard is not because it's not an artifact of our mind.
It just seems like we can't imagine a possible mind. And that might itself be an artifact of our mind.
We can't imagine a possible way that you could solve it regardless of what kind of mind you had. The final question is, what advice would you give to a 20-year-old who is interested in technical subjects? Maybe he's not doing a PhD program like you were at the time, but just interested in technical subjects.
Just learn all that you can. I mean, you know, there has never been a time when sort of more resources were available to anyone who wants to learn things.
So, you know, take courses, talk to your professors, you know, go on the internet and, you know, read books, you know, delve deeply into a subject. And, you know, you might be surprised at sort of how low the barriers sometimes are, right? Like, if you, you know, let's say that it was quantum computing that you were interested in, right? It doesn't have to be.
It could be anything else. But, you know, if you, you know, the entire literature of quantum computing pretty much is available for free on, you know, on archive.
go and look every night at the new quantum computing papers that come out and just flag the ones that are interesting to you and read them, each paper will raise new questions that the authors don't know the answer to or yet. and you they'll be explicitly listed in an open problem section.
Other times, there'll be ones that you could think of. And you can study those problems.
If you have ideas about them, you can, talk to the authors of the paper. And, you know, you, you know, it might, you know, it might take, you know, years or decades to become, you know, an expert, like in a whole field.
You know, and you might be very far from that, but it really doesn't take that long to become the world expert on one particular tiny little problem, right? And, you know, so try to, you know, become the world expert on something, you know, even something very, very narrow, right? And, you know, once you've done that, then you can, you know, write an article about it, or, you know, do a do a project about it. And then, you know, that will lead to more things, right, it will lead to, you know, maybe collaborations in the future.
You know, and it will lead to, you know, you can then try to become an expert on something a little bit wider, something a little bit wider, and so on.
Yeah, that's very excellent advice. I love that.
Okay, well, Professor, thank you so much for your time. try to become an expert on something a little bit wider and something a little bit wider and so on.
Yeah, that's very excellent advice. I love that.
Okay, well, Professor, thank you so much for your time. Hey, if you enjoyed this podcast, please consider sharing it with your friends and posting
it on social media. Word of mouth is incredibly valuable for a new and a small podcast like this
one. So thanks for watching.