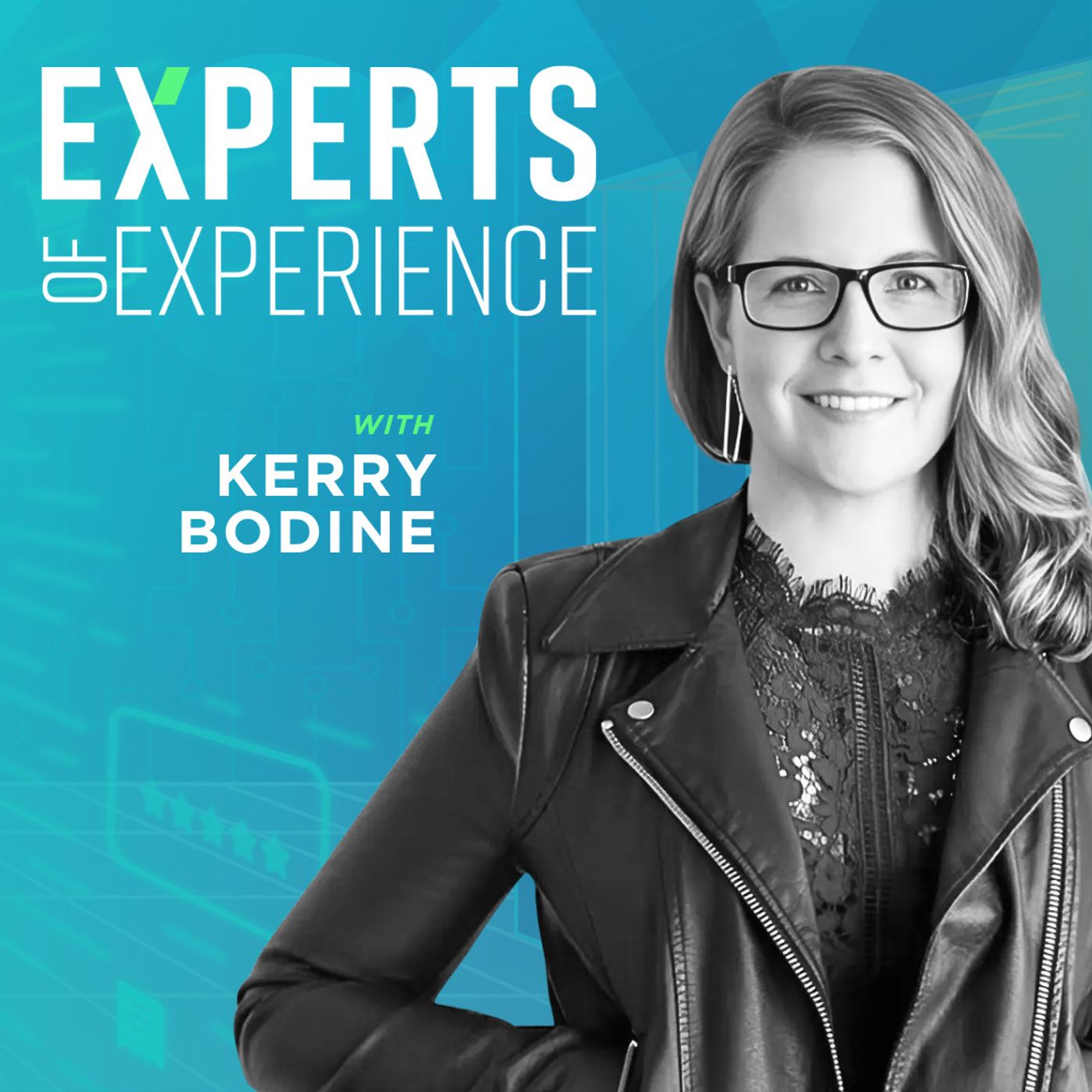
The Big AI Lie & Why You Still Don’t Feel Ready
Listen and Follow Along
Full Transcript
It doesn't matter what you think.
All that matters is what your customers think. In the world of customer experience, Carrie Bodine stands as one of the most influential voices shaping how businesses connect to their customers.
I don't think most organizations are understanding just how big the data lift is going to be to create all of these magical AI systems. We literally have AI employees on our team doing things.
This is leadership in the age of AI. One of the tools that we are teaching is a framework called consequence scanning.
Just because you can doesn't mean you should. They basically said we're not responsible for the answers that our chatbot provides.
That's completely ridiculous. It went to court and now it is on the books that yes, you are responsible.
Hello, everyone, and welcome back to Experts of Experience. I'm your host, Lauren Wood.
What happens when companies stop treating customer experience as a checkbox and start treating it as their competitive advantage? In the world of customer experience, Keri Bodine stands as one of the most influential voices shaping how businesses connect to their customers. She's the co-author of Outside In, a book that has helped many, many businesses reshape how they think about customer experience.
She's the founder of Bodine & Co, where she works with Fortune 500 brands and beyond to design more human and customer-centric experiences. And today, we're going to be diving into the business opportunity of effective CX strategies, the frameworks to design those strategies, and why designing with AI in mind is fundamentally different in how we approach customer experience.
Keri, so great to have you on the show. I am thrilled to be here, Lauren.
Thanks so much. Your name has come up many times as I've done this show.
Many people say you have to talk to Keri. She's such an incredible voice in the space.
And so I'm so thrilled to finally have you on the show. And in going through your work and reading what you have done, there's two key things that I really want to dive into today.
One is how human-centered experience design has always been key to customer experience when we think about how are we building for a customer. And then this piece of AI, because you're teaching a really interesting course on how to design for AI with the customer in mind that we want to dive into.
So we'll start with the basics of customer experience and all the incredible work that you've done in this space. And then we're going to dive into our second favorite topic on the show, which is AI.
So first and foremost, this is kind of a broad and big question, but I would love to hear it from you. How do you define customer experience? Customer experience is your customer's thoughts, emotions, and perceptions about all of their interactions as they do business with you.
So it doesn't matter what you think. All that matters is what your customers think.
That is the gospel. And I think so many people are like, oh, customer experience equals customer service.
No, it goes so much deeper than that. I see your eyes rolling.
And so my second question is, what do businesses typically get wrong as they approach customer experience?
Oh, gosh.
I think one of the things they get wrong is that they really have to go out and listen
to their customers.
So first of all, companies have all kinds of data at their disposal.
And I'm sure we're going to talk about data later in our conversation.
But really going out and having conversations, talking to your customers one-on-one and really
I think... about data later in our conversation, but really going out and having conversations, talking to your customers one-on-one and really hearing what it is they're trying to achieve, what's going on in their lives, what's bringing them to your organization in the first place.
People have very complex things going on in the background and they bring all of that to your website, to your call center, however they are interacting with you. And you've got to really understand that complexity in order to serve your customers in the way that they expect.
I think that something that you're saying that I really want to underscore is that our perceptions do not always equal reality. And often they do not.
We can look at the data and we can infer insights or we can infer assumptions about our customer, which get us part of the way. But until we actually speak to someone, until we can hear their voice, we can understand the context.
we can listen to their body language with our eyes and our ears, you know, until we do that, we can't fully understand where our customer is at. And I think that that is such an important thing.
There's companies that relentlessly speak to the customer and companies that think it's just a nice add-on. Yeah, exactly.
It's a big mistake. Exactly.
And there are organizations that, you know, they have retail outlets, whether that's an actual like retail commerce or a bank branch or whatever it is. And they do get the benefit of, you know, having their customers right there, being able to, like you said, pay attention to body language and potentially have more in-depth discussions about what's really going on with someone when they are submitting an insurance claim or purchasing a dress for a big event, whatever it is.
But those background stories are just as important when we're talking about digital interactions as well. The other thing, Lauren, that you just said about perceptions not equaling reality is that that's true from the customer perspective as well.
And so they may be on hold, let's say, for two minutes to talk to a human. But their perception might be that it was an hour and a half or just forever, just way too long.
And so we have to find a way to marry up what is the objective reality and what is the subjective reality, whether that's someone in the business or a customer. Why is it important that businesses do this? What's the opportunity at stake? Well, the opportunity, like you said earlier, is around competitive advantage.
Companies that truly get this, they win in the marketplace again and again and again. The challenge is that it's a long game.
When you are investing in customer experience, you're not going to see the results tomorrow or next week. You've got to have some degree of patience and just know that this is going to pay out down the road.
Now, how far down the road, that's going to be different for every organization and the degree of investment that they're putting in. But companies that are relentlessly managing every single decision to their quarterly results, they're not going to get this.
They're not going to get the strategic benefits of truly investing in customer experience like you and I are talking about. Yeah.
I mean, it comes down to lifetime value. And I think that's such a difficult metric sometimes to think about because it doesn't line up with our quarterly metrics.
If we are making an investment in the trust that our customer has in us, in the relationship that we have with our customer, in listening to our customer today, the benefits are not necessarily going to come in the next three months. This is investing in the long-term relationship with our customer.
And in the long run, the dividends certainly pay out. Logically, we understand that.
But when we get too focused on hitting our quarterly or even our monthly metrics, we lose the ability to really see how what we do today is going to impact this long-term relationship with our customer for a long time into the future. Right.
And the important thing to realize is that this is not an either or. This is not, oh, we just make these grand plans for somewhere down the road and hope that they'll work out.
Yes, we've got to make quarterly plans and all that. But the important thing to realize here is that quarterly earnings, we weren't born with those.
Those aren't embedded in our DNA. They were invented at a certain point, and I don't remember the exact year, but during the 20th century because we wanted to create more transparency into how organizations were doing.
We didn't want to wait for those annual reports. And so there is some benefit to them.
Totally. But we've really just focused on them.
How do you guide organizations to think about the long-term relationship with their customer and really shift their focus to build for that? Honestly, I think everyone is struggling with that. One of the things that I really focus on is just helping organizations take their customers' perspective, looking at the entire customer journey from the outside in and looking at where the pain points are, where there's frustration, even hidden pain points, like things like waiting for a response from an organization.
It's invisible to an organization, but it's a very real thing to a customer to be waiting hours, days, weeks, way too long to be hearing back with an answer. So taking the customer's perspective.
And then the second piece is looking at what's going on behind the scenes. People, process, technology, policies.
I mean, there's so much more. Compensation.
Compensation drives so much in terms of the behavior of an organization. And helping them realize how all of those factors that are hidden behind the scenes, how they bubble up and really do impact the customer experience, either directly or indirectly.
And once they can start to see some of those things, they realize that decisions that they might have made about a policy years ago are now catching up with them. And they start to realize that the decisions that they're taking today are going to impact the organization and the customer experience in years to come.
But really, this whole topic of taking a long-term approach, it really is my passion project right now because I feel that it's just so necessary in our organizations, in our own individual lives, even, because we are at a point in human history where we have at our disposal, the tools to really make significant changes in the world that we live in. And we've got to start thinking about those long-term consequences.
And along with that are the long-term benefits of really taking a beat right now to say, hey, what's the potential impact of these decisions that we're making? What do we want to create right now that's going to pay off in dividends in the future? What's inspiring you right now as you think about this topic? What's inspiring me right now is actually really personal. I want to create a better world for all of us who are alive right now and all the generations to come.
I also care a lot about the planet itself, regardless of humans on it. And so, yeah, again, we're really at this inflection point.
There's a lot of issues around AI and the natural resources that it takes for us to consume all of these AI-enabled products and services. And I want to help people and organizations make better choices so that we can all create a better world.
Thank you for saying that. And I could not agree more because I think that our kind of going into this topic of short-term thinking and long-term thinking, I think our short-term nature has caused a lot of the issues that we are seeing today.
If we talk about the environment, that's a whole rabbit hole. We're not going to go down right now, but I just have to say one thing.
It's another podcast, honestly, because I could talk about it forever, but it is what has caused us to get into this place where we're saying, I want this now. And so I'm just going to go and get it instead of thinking about the consequences and the repercussions of it.
And as we think about
our customer journey and the decisions that we make today and how it's going to impact our customer and our business in the long term, it's really to everyone's benefit to put our heads up and look further into the distance and really think through what can we do today that's going to impact tomorrow for better or for worse. And so I would love to talk about how you're helping companies and educating people on this topic as we look at AI.
Because it is radically shifting how we are going to operate in the very near future today. And we are just scratching the surface.
So how are you thinking about that? And how are you guiding people to the future? Say goodbye to chatbots and say hello to the first AI agent. AgentForce Service Agent makes self-service an actual joy for your customers with its conversational language anytime on any channel.
To learn more, visit salesforce.com slash agent force. So I'm teaching this course right now on AI, and it's all about how to evaluate and select the right AI projects to work on.
Essentially, projects that are going to be high value and low risk. And one of the tools that we are teaching is a framework called consequence scanning.
Consequence scanning was developed by this UK think tank called Dot Everyone. They have now closed up shop and their work has been taken over by the Ada Lovelace Institute.
I love this
because Ada Lovelace was our first woman computer scientist. But they have taken it over.
And actually, it was Salesforce who really popularized this tool in recent years. So right back to the heart of your podcast.
Here's how consequence scanning works. You take a two by two grid on the y axis, you put positive and negative.
And then on the x axis, you put unknown and known. And so for any decision you're going to make, and this could be about, you know, should we implement an AI chat bot? Should we, you know, move to Seattle? You know, really, it can be used for just about anything.
And when dot everyone created it, this was really probably not intended to be used with AI, but it works perfectly for AI. What are the positive intended consequences of this, whatever it is that we are thinking about developing and launching into the world? These are going to be, of course, the reasons why you're thinking about this as a feature or a product or a service in the first place.
But then you have to think about, okay, what are the negative known consequences? And you might think about, well, why would we have negative known consequences? Why would we do anything that we know is going to have negative consequences? Well, think about Uber and Lyft when they launched. One of the known negative consequences was that it was going to completely disrupt the taxi industry and all of the people who depended on that for their livelihood.
It was not great for the people involved in that, but it was certainly a known negative consequence that they were absolutely aware of. Then you think about, okay, what could be some possible negative unintended consequences? And this is really where you've got to go deep and think about, okay, what if people misuse this? What if hackers come in and want to take advantage of whatever service that we're creating.
What are the potential harms that could impact people, animals, the earth, global markets? I mean, there's all kinds of potential harms ranging from very small to really very broad reaching that would impact every human on Earth. What are some potential positive unintended consequences? The example that we like to use when we're teaching this in our class is the Ring Doorbell.
If you've seen some of their ads, they have this new ad out about all the moments of joy that the Ring Doorbell creates in terms of, you know, people dancing on their doorstop or, you know, pets running by, capturing people, talking to their friends and telling them they got into a college or, you know, whatever it is. But it's really an opportunity for you to amplify those potential positive unintended consequences in ways that are going to benefit the organization and potentially benefit others as well.
And then for the negative unintended consequences, you've got to find ways to mitigate those. And there are lots of different tactics for mitigation.
One of them being, this is such a big issue, it's going to cause so much harm. We need to abandon this idea altogether.
So the positive consequences, you want to amplify the negative ones you want to mitigate and possibly even say, yeah, we're scrapping this. I think the interesting thing about this, so thank you for breaking this out because I think that I'm like, I wrote it down.
I'm like, I'm going to use this for all my decisions now. It's like, I mean, I love a good two by two.
It just helps you to visualize what is happening here and really get all your thoughts down on paper. I mean, this is why I'm a total facilitation nerd, because if we are guided through having a conversation, especially a difficult one, like what we are talking about here, we can actually get to a decision in a much more meaningful way and faster way.
But I think the interesting thing about this is when we talk about the unintended negative consequences and those like big risks, those big looming risks that are, I mean, the way that AI is transforming our world today, even a year ago, we could not have seen some of the things that are happening for better and for worse, right? And there are some very large risks at play with just AI period. And we know we're still going down this path.
Globally,
together, we're all on this train. And there's just going to be some bad guys that jump on the train with us.
And it's part of it. And of course, we are responsible in mitigating those risks.
But how can organizations... The question I'm having is, how can organizations really make a decision of go or no go?
Like, how do we know if the risk is so big that we should abandon ship? And I want to just like play this out. And I know there's no black and white answer.
This is the nature of AI is it's gray area. And we have to use our instincts, our human instincts to decide if something is worthwhile or not.
And I'd love your thoughts on that because I think about this all the time. Like, how do we make the decision? And there's no right answer.
It's just how do we make that?
This is leadership in the age of AI. These are the really hairy questions that we all need to start being more comfortable grappling with.
To your point, this is not going away. Like, we got to figure this out pretty quickly, how we're going to make decisions, the frameworks and tools that we're going to use, our different thresholds for, you know, where we're going to where we're going to stand our ground, where we're going to take accountability for things.
One of the most ridiculous examples in my mind is the Air Canada example, where they basically said, we're not responsible for the answers that our chatbot provides. Well, that's completely ridiculous, but it went to court and now it is on the books that yes, you are responsible for the answers
that your AI provides to customers.
And so, you know, this is,
I'm sure there's gonna be a lot more lawsuits.
We're gonna have to figure things out together,
but the more that we can, again, take a pause
and think about this before we launch,
Well,
Thank you. we're going to have to figure things out together.
But the more that we can, again, take a pause and think about this before we launch, before we spend thousands, hundreds of thousands, millions of dollars, not to mention just time investing in something, let's take just a short period of time where we get people together who have different lenses from different parts of our business, and we talk about this. Why not do that? I love that you just said that the tool, the consequence scanning tool can be used really quickly.
We could talk about something and do it in five minutes. You could take a couple of weeks to do it, but that's nothing in terms of how long it's going to cost to develop a big AI product, service, feature, whatever it is.
And so take the time to do that, get out in front of some of those decisions beforehand. And then the other part of your question is, we all need to get better at just understanding the types of potential harms.
So there's psychological harms, there's financial harms, environmental harms. I mean, there's a whole list of the different types of harms.
The severity of the harms, this is a minor harm or really severe harm. And then there's a duration of the harm as well from acute to intergenerational.
If you build an AI that denies a certain portion of the population home loans, that has intergenerational impacts, which gets us back to our long-term thinking. And so there's just many aspects of how we need to really start thinking about the decisions that we're making.
And we need to start thinking about it now. Yeah.
I think you're also highlighting there's both an urgency in thinking about it, and there is a need to tread lightly just in terms of like a lot of people want to just dive into the AI world headfirst. I see a lot of organizations saying, okay, great.
We can turn AI on today and it's going to answer all of our tickets tomorrow. And like, yeah, it can.
It totally can. But should it? Just because you can doesn't mean you should.
And we need to take a minute to think about what could happen here. What could go wrong and how can we train the AI to not do that? How can we test it? How can we build trust in this new employee that we essentially just brought on the team to do all these things? And I actually think it's helpful to think about AI in terms of a human that is very multidimensional, especially as we get into agentic AI.
And we literally have AI employees on our team doing things because this is what's happening. We have AI employees on our teams doing things, and we need to train that employee and provide that employee with guardrails of you can do this and you cannot do this.
And like we have all learned through our leadership journeys, I am sure there are things that you didn't expect that someone would do, but they did anyways. And AI is even more unpredictable than humans.
So yeah. And one
of the things that I'm seeing in my classes and in my consulting is that a lot of leaders don't
even really understand that there's, when you talk about training, there's the data and there's the model. And, you know, there are different ways that you can train your AI employee by changing, you know, different parts of that equation.
And data, oh my gosh. I mean, again, this could be an entire episode, but I'm doing research right now on the AI readiness of organizations in terms of their data.
And, you know, if you, if you talk to anyone, there's data issues in any one system, and then you need to start connecting those systems and making sure that the data is consistent across all. Oh my gosh, data is going to be one of the biggest areas that organizations need to focus on.
And I don't think most organizations are understanding just how big the data lift is going to be in order for them to create all of these magical AI systems that they are envisioning. Okay, let's dive into this because I totally agree with you.
Organizations are not understanding the level of the lift. So where do we start? What advice would you give to an organization who's like, we want to use AI? Where do we need to start? First of all, you've just got to understand what it means for data to be AI ready.
You've got to understand all the different characteristics that that entails. You've got to look at your data management processes, governance, how you're dealing with privacy.
I mean, there's all types of issues. How often are you updating your data that you're feeding into your AI? How often do you need to? I mean, there's AI systems that can run on data from a couple of years ago.
And then there's AI systems that require real-time data. Which type of system are you looking to build? And then you've got to start doing an inventory of all of the different data that you've got in your organization.
You know, where is data housed? Not only within systems, but within business units, within different silos, functional units within an organization. And really what we need to have is much more collaboration between the business side of the organization and the IT side.
And we've got to have people and we have people in these roles, business analysts. But their role, I think, is just going to get so much more important.
We're going to need people who can translate from database language into, you know trying to achieve on the website or their mobile app. So we've just got to start collaborating and connecting those silos.
This has been a message that we've been trying to get across for the past couple of decades with customer experience. And the organizations that heeded
that call a while ago are going to be in a better place. But for organizations that still really are
working in silos, you got to start working on that right now.
And when you say work on it, is it like different tooling, different roles of people managing the
data? Let's go a layer deeper. How can people start working on it? It's both.
So again, I think that role of akin to what is the business analyst today that typically sits in IT and can kind of be that glue, that translator. I think that role is going to be important.
It may even morph into a very specific AI-focused business analyst role. So I wouldn't be surprised to see that happen in this year and beyond.
And yeah, and there's also a technology piece that you can put into place that is going to help connect all of those different parts of your data ecosystem. them.
I'm going to speak to something that I actually don't know that much about, but something that I just think is just a message that I, the drum I am drumming these days is I really encourage organizations to go and speak to their current providers. I think of my clients who are on Tableau or Salesforce Data Cloud or any of these, Looker, whatever it is that they're using.
Go to the organization, go to the provider that you currently have and ask them, how can I be more AI ready? I bet you that there is information there and support or new tools or access to what you need to at least start this. Because I always want to break things down to like, what's the next step? And I know I have some clients who are completely overwhelmed by the concept of reimagining how they approach data.
It's like we have been stuffing stuff in a closet for a long time. And now we have to go and open that door and clean it up.
That is, I don't want to do it. But we have to start.
It is essential that you start. And so one tip I would give to people is just look at what new technology has come out from your current provider as a starting point.
And then see if that's fitting. Also have conversations on your team about what data do we need to feed our AI? What AI do we want to start bringing in? And what does that AI need in order to operate? Let's just start breaking down this really big, ugly problem into something that is more actionable because avoiding it is not going to help you.
We have to start taking little bites at the very least out of this apple. Absolutely.
And so yes, going to your current providers is a great step. I would also say if you are in the process, if you're doing an RFP for any type of technology, it's got to be a question that you're asking of any new provider that you're bringing into the ecosystem.
How are you going to connect with all of the other systems and platforms that we have? And then the other thing, this is something that we talk about a lot in my class as well, is that a human-centered design team now has to include a data scientist. You've got to have someone from the data side coming in and providing that lens as to, you know, what you have today, what you don't have, how difficult different pieces are going to be to hook up.
Because your typical, let's say, product manager, UX designer, human-centered designer, marketing manager, that's just not the world that they think in. That's not to say that they're not capable of learning that, of course.
But it's just something that is new that they've got to start learning. And we've been through this before, right? We didn't have the web at one point.
And then mid-1990s, we started to have to take our existing roles and our existing processes and learn how to integrate them with this new technology. And then the same thing happened with mobile.
And now, you know, and it's going to happen with something else in the future. So I would say, you know, embracing all of those different perspectives from different parts of your organization.
I've always said, oh, you need to bring people from finance and operations and IT into your human-centered design process. But now that data voice is just absolutely critical if you're thinking about anything related to AI.
I'm glad you brought up human-centered design because I know that's a lot of what your course is about and how the approach to human-centered design is changing with AI. And so one piece of that, as you just shared, is having data be a part of it.
How else do we need to be approaching human-centered design differently in the age of AI? Yeah. So a lot of the material from the course is based on research that's coming out of Carnegie Mellon University, where I actually went to school and I'm co-teaching with a colleague of mine who we were in our master's programs at the same time.
And he's now back as a professor there. So he is bringing all of this research out to the professional community.
And so one of the things that Carnegie Mellon has found is that they tend to come up with solutions that require a lot of technical accuracy and therefore require a lot of effort to develop and therefore have a lot of risk involved if they don't operate in the way that we've envisioned when we're putting up sticky notes on a wall. So what the researchers at CMU have found is that rather than just going into a brainstorming process, you know, kind of with a blank slate, it's much more effective to understand not the technology behind AI, but the functional capabilities that AI has.
And I really love this because, you know, the functional capability that we've all been gaga over for the past couple of years has been generate, generating text, generating images, generating code, whatever it is. And now, of course, with agentic AI, we're focused on this capability of ACT.
But those are just two of eight very broad capabilities that we go into in the class. And these examples of these eight great capabilities, as we like to call them, they're all around us.
They're sitting on your phone. You use them every single day, but you don't think of them as AI because once a technology moves from magical to, you know, I just use it every single day, we don't think of it as that technology anymore.
We don't think about type ahead as being AI, but it is. And we use it every single day, all of us.
So that's one of the things that we really focus on in the class is getting folks who are not technical. We do give them some background on, hey, here are the different types of AI models and here are examples of each and here's the type of data that each use and the data requirements.
We give them that basis. But then we're like, you know what? You can forget about all of that and really just focus on what the AI does.
And then use that as a platform for your brainstorming. Oh, that's so exciting.
And you're just getting my wheels turning. There's so much for us to learn when it comes to looking towards our AI future.
There is so much opportunity. There is so much risk.
There's so many things to consider. And the way that we think through approaching it is not, we cannot just think through it in the same way that we've thought through other technology implementations that we've done.
It really requires a mindset shift. And I'm so excited that you are bringing that research to the table to teach people how to really go through that
thought process.
So I'm very excited to learn more about that and take your course. I'm in the next cohort for sure.
Oh, excellent. Excellent.
Yeah. You know, I have been teaching human, practicing, teaching, leading human-centered design for decades.
And it really hasn't been until AI came on the scene that our traditional methods, we really didn't have to change them all that much, a little bit here and there. But the non-deterministic nature of AI, it just means we have to start in a different place.
We actually don't start with human needs, which to me, like I felt my brain exploding when I saw the research on this. So yeah, it's been, it's been incredible.
It's been a huge learning process for me, really humbling too, to be like, oh, I got to let some of this stuff go. These, these things I held so tightly to I got to let some of that go.
It's really been just a huge learning experience. Really fun.
An unlearning and a learning experience. I think we're all going to go through that very, very rapidly here.
Well, Keri, thank you so much for coming on the show. How can people find out more about you? They can go to keribodin.com slash AI.
And I'll have all kinds of information there. That's a page I update all the time with all of my latest thinking and classes that I'm putting out.
So just a great place to go. Amazing.
Well, thank you again. And we'll definitely be in touch.
Thank you so much, Lauren. And if I could leave with just one message, it's just to think about those long-term consequences
of the actions and decisions you are making every day.
That is very important advice.
I really appreciate it.
Thank you so much.