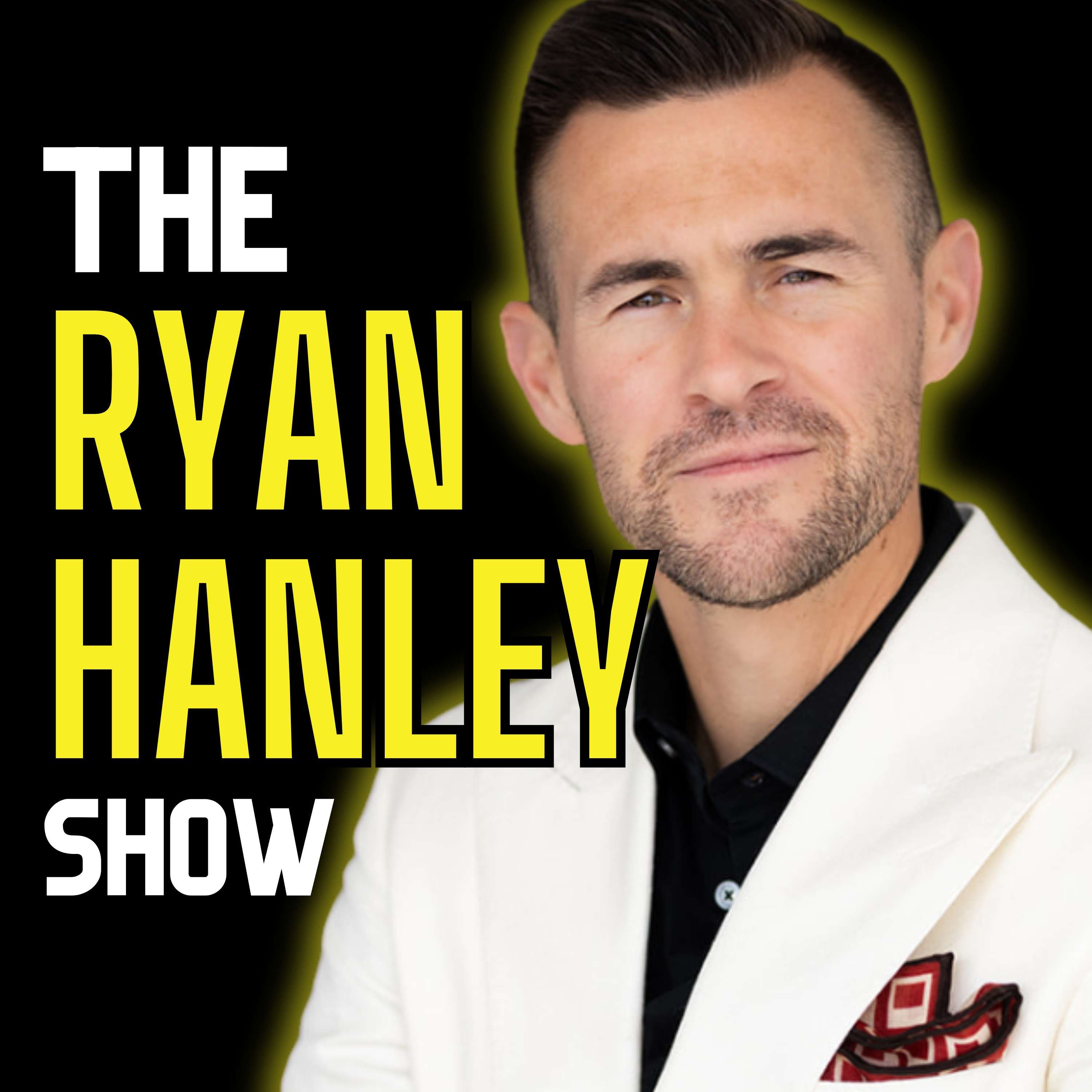
RHS 165 - AI, Data & When to Ask the Right Questions
Listen and Follow Along
Full Transcript
I need directions for paying down debt.
Starting route.
Apply for a SoFi personal loan and consolidate your debt into one fixed payment.
Huh.
Turn right into a positive outlook and get $5,000 to $100,000 as soon as the same day you sign with no fees required.
Got it.
You could get out of high interest credit card debt with a SoFi personal loan.
View your rate at SoFi.com slash debt in 60 seconds with no impact to your credit score.
Loans originated by SoFi Bank in A. Member FDIC.
Terms and conditions at SoFi.com slash debt. NMLS 696891.
In a crude laboratory in the basement of his home. Hello, everyone, and welcome back to the show.
Today, we have an absolutely tremendous episode for you. It is a conversation with Dave and Helen Edwards, authors of Make Better Decisions, a tremendous new book with the subtitle of How to Improve Your Decision-Making in the we talk, this is really a fantastic conversation.
It's one of those conversations that like, it's why I love doing these podcasts. You get to meet new people that you didn't know who are doing awesome things with great ideas.
And we talk a lot about how to make great decisions, how to integrate those decisions in the massive amount of data that we have. What is the value of data? When should we use data? When should we go with intuition and instinct as leaders? This is a fantastic conversation.
I took quite literally two and a half, three full pages of notes during this conversation. I could have talked to these guys all day.
And I have the book. I'm reading the book.
It's wonderful.
It is very much something that is worth picking up.
You can make better decisions on Amazon or anywhere that books are sold.
You can always go to the show notes for the page and find the book link there if you want.
Wherever you consume books, you can find this book.
I highly recommend it.
I really like it. I think you're going to know exactly what I'm talking about after you have a chance to listen to Dave and Helen and their thoughts on how to make better decisions.
It's a tremendous conversation. Before we get there, guys, make sure that you are subscribed to Finding Peak.
Go to findingpeak.com. It is my new sub stack.
Free content coming out every week around peak performance in business, in life, and in insurance, specifically tailored to us, the insurance industry. We do a wide range of topics, everything from personal development to leadership development, development in business, our relationships, and also deep dives into marketing, into lead generation into into digital sales, into what we're doing at Rogue Risk to be a human-optimized digital agency.
Very much the model that I believe is the future of the insurance industry, the future of the independent agency. If you want to learn how we're doing it, go to findingpeak.com, subscribe, get the emails, and if you want the deep dives, you can pay for that, which is like seven bucks a month.
So appreciate you guys for listening to this show. As always, this is a labor of love, and I just love that you guys give me your time, so I appreciate you.
With all that said, it is time to get on to our conversation with Dave and Helen Edwards, co-founders of Saunders Studios and the authors of Make Better Decisions. Awesome.
Well, I'm excited to talk to you guys. Thank you.
Same here. Yeah.
I went through and looked at a lot of what you're doing.
And I think that it's incredibly relevant to particularly the audience who listens to this show, which is primarily insurance professionals from up and down the spectrum. So our audience is individuals from, you know, everything from one person, startup agencies in small town, wherever, America, to executives at the highest level and corporations in Hartford and all the different places, Des Moines and Columbus and all the places where insurance companies operate, primarily in the US.
So just so you know who we're talking to today. But normally I like to get right into the show.
So I'd love if you guys maybe start with your origin story. Obviously I'd done some background, but I'd love to hear kind of, you know, every good superhero duo has an origin story.
And maybe we start there and we dig into some of the stuff that I, that I think is incredibly relevant to what's happening. Okay.
Did we just launch in? Yeah. Yeah, rock and roll.
We're talking. Sounds good.
Awesome. Well, thanks for having us.
So I guess we've been working together for more than a decade. I've lost track of the number of years.
We've started multiple companies. Some have worked out.
Some haven't as well well as the others. Sonder Studio has been around since 2019-ish.
And it continues work that we've done for several years. We started off working really closely around thinking about how do humans and machines come together? And what's happening to us as individuals as we are digitized, as our behavior is being monitored, as our communications are being managed, as the algorithms are making decisions for us and pointing things out in the world.
As we've been put into finer and finer grain buckets. Finer and finer grain buckets and being ultra personalized, but in a way that we can't interrogate and understand because we can't see it.
And we sort of started Sondra Studio with the mission of really wanting to open people's minds of the richness of humans while we're in this digital age, you know, that it's not us being supplanted. It is actually, where's the beauty and where's the wonderful parts of being human? And how do we help people understand that? Yeah.
I mean, so many, you know, when we kind of got into this, the, the, the, the zeitgeist, if you like, was, you know, very much an either or it's either machines or humans and the machines are going to rule us all. And the more we looked into it, the more we did the research, the more we talked to people, the less we were convinced of that story.
And so this is very much a, how do we do both? Yeah. And we spent time with organizations that sit there and say, we've spent all of this money on these huge data projects and putting all kinds of AI into organizations to make more predictive analytics.
And it's not really working or people aren't really using it, or they feel like decisions are harder than they would have been before with all of this data. What do we do about this? And that was the genesis
of the book, Make Better Decisions, was helping people really understand the core of who we are as humans and who we are as decision makers, as individuals, who we are as team decision makers, and how we think about making decisions with data and with AI. And that book accompanies workshops that we do with large organizations.
And we also are have started up working in complex problem solving, which is an interesting area of thinking about complexity. And how do you think about solving complex problems in a way that it's quite different from simple or complicated problems? There's lots to unpack there, which is awesome.
That makes my job very easy so i'll give you some context to some of the issues that we're facing specifically in the insurance industry and then i think it's going to be highly relevant to what you guys do and i think we'll have an awesome conversation here so um you know i actually i own uh a independent insurance agency called rogue risk. One of the very first things that I wrote down was the term human optimized.
And what I meant by that was not necessarily all the way to, uh, a, a, I M L situation. However, um, what, what I realized throughout my career, having done this for 17 years and spending a lot of time on the traditional side, is that the all-human version of our business was dying.
There are plenty of boomers that are holding on for dear life to the paper, file cabinet, very human, all-human version of this business. And they've been highly successful in that method.
But we are rapidly changing into an ecosystem that most industries have already moved into, which is this mixed up, mashed up, you know, what is the value of data? What do we use? What data actually allows us to have better outcomes? You know, how do we capture it? Who owns it? You know, I mean, there's all these crazy decisions happening. And kind of the premise of my agency was that there are moments that add value.
And there are moments that don't. And I want the humans only spending time or spending the most time possible in the moments that add value and have systems,
processes, use data, feedbacks. And eventually I think we get to a place where we're using
some form of AI. I've been playing around with open AI a lot lately to handle those
processes that don't add value, the humans don't add value to, right? So you waste a lot of time,
a lot of energy, resources, brain cycles throughout the day, doing stuff as a straight human, that as a full human, not necessarily a, that wasn't a comment on sexual orientation. As a, as a, just a human, you lose a lot of time and value and energy just doing all these things that don't matter.
So, so where do you mash those up? Where, where do you, what value, what that actually is value are, are enormous questions in our industry. I mean, we still, I think we employ, I think, I think something like 80% of the COBOL programmers left in the, in the world are employed in the insurance industry in the United States.
So like, it's this, it's this snap forward of technology. And now we're having these types of conversations and I don't think anybody has an answer and no one is doing it well.
So, um, you know, kind of unpacking what you said and maybe one place that I'd like to start, um, just because it's a, it's a enormous buzz term in most industries, but certainly in ours is data. And frankly, the two questions that I wrote down and related to them was, can we have too much value and how, or can we have too much data and what does that look like? And does data even have value? And this is a conversation I've had multiple times in this podcast.
Is it the data that has value or is it what you bring out of the data? What does all that mean? You know, kind of, let's start with the actual nuts and bolts and try to get to how we use it to make better decisions as we go. Data definitely has value once people understand it, right? Or machines understand it.
And that's a,
let's start with that, that why do we even want AI in the first place? And it's because humans think in one, two, three, four, lots and lots, and machines can think in unlimited dimensions. and this ability for machines to take data
that in some cases is really quite alien or seemingly inhuman, like collected below our conscious, outside of our conscious recognition, eye tracking, mouse clicks and things like that, that the machines can find patterns in that at enormous dimensions. Now, there's really no real sort of practical limit other than compute power and cost.
But the problem with that is that eventually, for most situations, a human has to be able to justify how they use that prediction from a machine. So if the prediction from the machine is decoupled from the human level decision making, which is what you'd expect in most human facing products, then we need to have accountability and responsibility.
And we need some sort of justification, which generally means some kind of causality, some not just a correlation, which is what the machines are good at. And humans come in because they need to be, it's only us that can really put the causation and put the justification and say, yeah, the machine says this, but we're going to do this, or we're going to do this anyway, or we're going to follow what the machine says, and this is why.
And that level of responsibility and accountability that sits only with humans for now, the danger is, of course, that we fail to recognize that. And that's really what the sort of AI ethicists work on, is how do you stitch together hidden bias in data sets and make the right decision on top of that? So I can see Dave's itching to jump in here.
I would add that what matters with humans is that data can be utterly overwhelming. That high dimensional space is completely, it's like trying to imagine, you know, it's like trying to apply quantum theory to something.
It's just not intuitive. And people make mistakes on that basis and become overwhelmed.
So I think a lot of the dichotomy that we hear around, well, is data of value? Is it too big? Is it this or that? It's actually really more about how humans tackle overwhelming amounts of data. That's really what our book is about, is ways to not be overwhelmed and to recognize when human cognitive biases work against you in your judgment and decision-making.
I think that there's a, the distinction for me is that data has value or at least hypothetically value, right? There can obviously be data that has zero value, I guess, but the challenge is what in order for it to, for that value to translate to us, we humans have to know what it means.
And we generally communicate things about what one thing means when we're communicating
with each other by telling stories.
It could be a simple story.
Here's the story for why this is the primary customer target.
Here's the story for why this is the right insurance product for you.
Here's the story of the US American dollar, which is essentially a story.
The challenge is that data doesn't tell stories. We have to tell the stories with the data.
And that's a gap that is, I think, misunderstood and easily overlooked. Because people are used to seeing these great dashboards.
You log in and you look at your tableau and it's got all kinds of colors and lines and things. And well, doesn't that tell you everything you need? Well, no, because it's not telling you any sort of story over time.
It's not telling you any cause and effect. It's not applying it any form of context that we understand naturally because we've evolved to be able to communicate with each other using stories.
But data is a really recent addition into this whole concept. And we actually just don't look at data.
Even when it's presented in a two-by-two, in a two-dimensional space, we don't naturally know and understand and agree upon the story that's there. So we have to translate it.
That's a difficult thing for a lot of people. One of my most interesting takeaways in the move from being a foot soldier in a company to being a leader was how differently the same piece of information could be interpreted by a group of people, right? You present a stat on a screen to a group of 10 leaders in an executive forum and the feedback you get from the angles that everyone slices that singular piece of data up is incredible.
We recently did, so we were acquired back in April, um, uh, by a larger holding company. And I'm now on the executive leadership team of that holding company.
And, um, you know, so all the division leaders get together and there's 17 of us total and, uh, whatever. And we're walking through different departments and Hey, you know, this result, and we're seeing this and, you know, we're our variances off here.
And, you know, why do we think that might be happening? And. Like you said, this without a story to the data, the why of that of something all I mean, it is just personal context, filters, biases, experience, you know know all the it gets passed through all these different things and what comes out the other side is like you're you almost start to think like which one of us is the crazy one like we're all staring at the same piece of data yet it's seemingly seeing completely different pictures and I think that's where I sometimes get lost in my own leadership is how much do I trust the data and how much do I trust my gut? And what does that look like? Where is, you know, one of the things I wrote down during your kind of introduction or origin story was, you know, where's the nuance? Where do we, how do we understand nuance in a data rich world? Um, or something that scares me, um, mostly because probably I read too much is I'm a big fan of, um, Nassim Nicholas Taleb.
And right now I'm plowing through his epic, uh, uh, anti-fragile. I don't know if you've read that book, but, um, he talks about, um, black swans and, you know, the, the, the triad or whatever.
And I always think to myself, I, when I look at data and I think patterns and I think pattern recognition and, and all these kinds of things, are we creating fragility in our business? Because pattern recognition essentially starts to carve out black swan events. You're right.
We start to see things as how they happen on the mean or in the average. And we don't realize that there could be this massive thing coming that maybe our gut as humans and experience could possibly see, not always, but a pattern recognized data set that's pushing everything to means and averages and giving you variances tends to carve off.
And, you know, is that a concern or something we have to negotiate? Well, there's a couple of things that you raised there. First, I'll go back to the very first thing you pulled up, which is essentially sort of analysis versus gut feeling.
That's where we start our leadership workshops with exactly that question. And we start it from the perspective of,
well, you know, modern neuroscience is telling us that everything that we, that all decisions are emotional and that here's why. And we kind of unpack that and we unpack what heart versus head means these days, you know, the sort of, and then we look at the sort of fast and slow thinking that, and how to, how to trigger better ways of thinking.
And what, what's really going on when it comes to finding that nuance is, is quite complex. You know, you've got a mix of a bias for machine learning or a bias for automation and taking what a machine says, giving higher weight to that recommendation than you would even in the face of evidence to the contrary.
So the classic example of the people that follow Google Maps into a lake. But these things happen all the time with data because you put you put a good dashboard in front of someone and all of a sudden they forget to ask the good question.
So it's like, how do you step in and how do you intervene and know when you should ask the right questions and what are those questions? And this is a very human process. And sitting around that table, presenting people with one data point that they see differently, we sort of give people a bit of a release from that because that's quite anxiety provoking.
And because the sort of the promise of the analytics movement has bled into how we think about each other. So the promise of the analytics movement is that there's one single optimizable answer that can be found best by a machine, not a human.
And we forget that all difficult decisions by definition are difficult because people have different perspectives. So then why do we have different perspectives? Our cause and effect reasoning causes us to think in quite noisy ways.
This is recent work by Daniel Kahneman and Saboni. And we have this noisy, undesirable variability in our thinking.
That variability can be desirable. It's called creativity, right? We all have a different perspective, but we'll show people perception, illusions, perception, pictures.
We'll ask them, what do they see? And everyone sees something different. It's quite predictable that everyone sees something different.
So we shouldn't be surprised that everyone sees something different in the same data. The question that then becomes, what do you do about that? And what you do about that is firstly, embrace that diversity, that diversity in thinking is what's going to get you through a complex problem.
And there's lots of techniques for optimizing and maximizing the human part of that. Some of it is, well, people do need to have what we call minimum viable math.
You know, you really, especially in something like insurance, you should be sitting there, should know what a mean is, should know what a standard deviation is. You'd be surprised, unfortunately.
I'm not, Yeah, I get it. That's why we teach minimum viable math.
To give everyone the same common language. And so that, especially if you're using machine learning or any kind of predictive analytics, you really need to understand what a false positive is.
You really need to understand what a false negative is. You really need to understand how different cohorts in the data can optimizing for different things in those different cohorts can give you unintended outcomes and overall profitability, for example.
And the final thing I'll just want to touch on is what you were talking about there in terms of the black swan. And this is a new product for us, but moving from decisions to complexity, a lot of our traditional tools, whether they be analysis tools or processes and decision-making structures in organizations are just not fit for purpose
when it comes to this new world of complexity.
And whether it's because we are sorting
by such finer and finer grain cohorts in the data,
whether people on the other end have so much more agency.
20 years ago, you didn't really know
what someone thought of you.
And now you know. Social media will tell you what they think of you.
And these things can be organized. And the self-organization and this decentralization of control and this emergent property that is now humanity on the internet that touches all businesses.
Normal statistics just flat out doesn't work. We have to turn to complexity science, which is coming to the point that there are new heuristics and new shortcuts that we can take out of complexity research.
And a huge amount happened during the during COVID, just in terms of understanding epidemiological models and things like that. But that math is just, I mean, the insurance industry is probably one of the few industries that's poised to adopt some of that complex math to help with decision making.
But until humans really have access to some of this new science, we have to kind of glean lessons out of it. And that's how we deal with the black swan, is releasing yourself from this need to sort of have every, it's really a different way of looking an uncertainty.
It's not trying to say that, well, there's a 0.01% chance of X, because we know that that's just too hard for people to deal with. In fact, actually, that one's not so bad.
I mean, what are the probabilities we understand? 1%, 99%, 50%, 0%, and 100%. Those are the only five probabilities that humans intuitively understand.
I think that came from Richard Thaler. But we try and help people think in a much more dynamic, open, complex, networked way so that you can be sort of released from this tyranny of having to really sort of grapple with uncertainty in a way that's just counter or not intuitive to us and open up the team to thinking much more dynamically, to solving problems as they come at you, to being much more agile about how you use experimentation.
And you just see the data in a different way. It really is a totally different way of thinking.
Yeah. One of the things that you have in your book, which wasn't a huge topic, but it was a topic that I was very interested in.
I just want to bring it up considering my audience probably is on the lighter side with some of these topics, of familiarity with them. But I think, you know, when we're looking at, say, the, and I know where you've talked about predictive analytics, but still those predictions are based on past experience.
And, you know, one of the, one of the, I don't want to say questions, because I had, it hasn't been presented to me, but that, you know, people have framed multiple times in different, you know, when I'm dealing with big dashboards and stuff is, you know, the concept of how do we know, how do we know when to step away from the data and trust say our gut. Right.
And having been in business for 20 plus years now, and I know you guys have been in business for a long time too. I think it's undeniable to say there's moments where you look at everything the way that it looks and you're like, nah, we got to go this way, right? Here's the answers here.
And you're like, why? I'm not a hundred percent sure. I see this and I see this over here and I feel this and, you know, there's this swelling and I just can't explain a hundred percent other than I know this is a direction we at least have to try.
Right. And that's a really hard call.
Those calls are becoming even tougher now that we have so much data behind every decision, right? You, you, you struggle to justify, you know, one of the things I seemingly have seen in some of the organizations that I've been in is that more data leads to more bureaucracy. People are less willing to take chances because that those chances aren't necessarily backed up by the data that's given to them.
So how do you, if you're a leader and like, unfortunately, my style tends to be more wrecking ball than craftsmen, but how do you know or what is a good heuristic for when to take the leap away from the data and when to stick to it? And I know that's not an easy question, but I know it's a question a lot of people in our industry in particular are dealing with. I'm sure many more are as well, but it's a very common question.
Okay. We see this happening.
Feels like we should go this way really, but the data is telling us to go here and how do we manage that? How do we manage that divergence? Yeah. I mean, I think it's a terrific question.
I think it's the core of sort of where we all are right now, because what you highlighted is that the data can make us quite risk averse. Yeah.
We need the next data. If so much data is available, surely the answer is there.
So there's a couple of things, and this is really why we wrote this book, because you can't go head on into any of this. That there's a subtlety.
The real nuance has been able to sort of look at it from lots of different angles. It's pick up your wrecking ball and turn it around a few times.
And the first one is feelings, that there's no question that feelings come first. If you don't like the way that graph looks, you're going to feel it.
And that feeling is going to impact how you evaluate it. Yeah.
It's part of how you, it's part of the, we don't sample from our brains in a way that like a computer does. It's a probability distribution, depending on how we feel, we're going to take a different reaction to data.
So the first thing is how are your feelings actually influencing the way you process information? Another reason, another thing, another nudge that I use all the time is ambiguous data. If the data is unclear, if the answer isn't in the data, then we have a natural tendency to use our intuition and our gut.
So as a leader, you step back and you say, well, why is the answer not in the data? Is this question actually not able to be answered by the data? Or is the data not representative in a way that helps me make a decision? Yeah. So going to that next level of, do I want to use my gut because the data is not clear, or do I want to use my gut because of some other reason? There's a lot of interesting research out of, I can't remember who did it now, but that founder-led organizations are able to take a lot more step away from the data moment.
And that's because the founder has more scope. They're seen as more able to take risky bets.
And it's because their name's on it. There's an accountability thing.
So being able to decouple what the data says is the right decision from what the decision that is made by the actual human. And that's okay.
You know, there's data is past events. It's relying on a stable world.
It's possibly biased. So are people, but there's going to be bias in there.
Data is not imaginative. It has no ability to make transformational creative leaps.
It can only be used in the service of those things. So in the end, it's totally fine that a human makes that decision.
But I think that we have got ourselves in a little bit of a knot because of this promise of the analytics movement that the answer is in the data. It may not be.
Let me go back to what you started with around feelings. And I think that it's an important one, especially as you described yourself as saying that, you know, my leadership style is using a wrecking ball.
So my question is what's the, what's the, what's your emotional sweet spot for making a good decision? Because when we're highly charged, when they're highly stressed, we will lean more on intuition. That's we've evolved to do that.
That's why we run when something is really stressful, when something is scary. Those kinds of emotions, when we're highly charged, will lead us to use our intuition more.
So the question is, what is your emotional sweet spot that allows you to find the places where your intuition is actually reliable? Intuition is great, by the way. It's cheap, cognitively cheap.
It's generally good enough. It is all based on data, meaning the data of our own individual experience.
But it is something that's quite useful. The question is where's the emotional sweet spot that allows you to say, I'm going to slow down and I'm going to consider this a little bit more.
I'm going to think through this.
And I think the next step would be to really evaluate one of the nudges in our book is talking about experience versus data.
So when you look at that data and you go, I don't think so.
To stop and query, what is it?
What is it about your experience that's different from what the data is telling you?
And then thinking about how those two might be, well, you might want to rely on one or
the other experience versus data or combine the two of them.
So for instance, we recently done some work with a big retail operation and the data about what happens in the retail stores can differ from individual experiences working in those stores. That makes sense, right? Large data, individual experience.
Sometimes one is more important than the other, but sometimes you have to put, you have to mesh them together in order to make a decision. You can't just blindly follow one or the other.
You have to go into it and you learn from the extra context of, well, my experience is different from the data and here's why. Okay.
Now that I know why, what do I want to do with that? Why? Resolving that anomaly is I think a really important step. And it's actually really fun to do.
If your gut feeling is telling you something really different than the data, like you said, you explained the process of sort of digging into it more, but resolving that anomaly can be extremely satisfying. It's that one of those aha moments.
Oh, you know, for example, we use a fun little case study that it's come out of Tim Hartford's work about his experience of the London Underground, where the trains are just packed all the time. But the data collected by Transport London suggests that the trains are empty.
And he's like, wow, this doesn't make any sense. So he dug into the data and he explained about how the measurement's taken, because, you know, really understanding that exactly the moment the measurement's taken and why and who's looking at it.
And his pithy sort of putting, you know, integration of the story is, well, one, Transport for London measures the experience of the trains, whereas he measures the experience of the people. And that's such a lovely insight, right? How do you move from measuring the experience of the trains to measuring the experience of the people? So we sort of nudge, we have these nudges that have you really dig into it from the perspective of, well, where is exactly that data point is taken? Why is it taken? Who's looking at it? And what kind of processing happens before you see it as a chart or a graph or a table? And what you find as you step through that process is you realize, huh, some of this was taken for an entirely different reason.
It's measuring a completely different experience. Yeah.
What's up, guys? Sorry to take you away from the episode. But as you know, we do not run ads on this show.
And in exchange for that, I need your help. If you're loving this episode, if you enjoy this podcast, whether you're watching on YouTube or you're listening on your favorite podcast platform, I would love for you to subscribe, share, comment if you're on YouTube, leave a rating review if you're on Spotify or Apple iTunes, et cetera.
This helps the show grow. It helps me bring more guests in.
We have a tremendous lineup of people coming in, men and women who've done incredible things, sharing their stories around peak performance, leadership, growth, sales, the things that are going to help you grow as a person and grow your business. But they all check out comments, ratings, reviews.
They check out all this information before they come on. So as I reach out to more and more people and want to bring them in and share their stories with you, I need your help.
Share the show. Subscribe if you're not subscribed.
And I'd love for you to leave a comment about the show because I read all the comments. Or if you're on Apple or Spotify, leave a rating review of this show.
I love you for listening to this show. And I hope you enjoy it listening as much as I do creating the show for you.
All right, I'm out of here. Peace.
Let's get back to the episode. I love that concept of whose experience are you measuring? I love that.
So a couple things. One, they actually just discussed the concept of around founders making more decisions off of judgment.
I don't know if you guys listen to the All In podcast, which is like a big entrepreneurial podcast and whatever. But, you know, one of the hosts, Jason Kalkanis, said that there's I'm going to forget the stats.
I'm not going to try to quote it, but there's some statistic on there's a certain percentage of equity at which
once the founder is below that, they compress down into almost like they stop taking chances,
they stop stretching, they stop breaking new boundaries. They just really start day-to-day
operationally running the company. But any innovation slows, all these things kind of slow
because it has to do with the fact that at a certain point of equity, they know they can be fired. And like when they, as soon as the founder hits, whatever that percentage of equity is that they can potentially be fired, like the, the percentage of growth, innovation and everything just compresses way down because now they can't step out onto a ledge and come back from it.
And I find that to be incredibly interesting because I have been fired multiple times and
seemingly because I have not learned whatever that trigger point is that's broken in my brain.
So, you know, and again, to the part you asked the question, like, you know, your leadership
style is to be more of a wrecking ball. And oftentimes I think the reason that
Thank you. um, you know, and, and again, to the part you asked the question, like, you know, your leadership style is to be more of a wrecking ball.
And, and, and oftentimes I think, um, the reason that I prefer that method personally is I like to know the actual answer. I really struggle with, um, uh, armchair, I don't know why to call it quarterbacking because we're not playing football, but youchair decision-making, you know what I mean? Where we kind of, if I try something and I get a result, then I know what the answer is versus if I just kind of sit back and go, well, we think this is what would happen if we tried that thing.
So we're not going to do it. I tend to just be like, okay, let's do it.
Let's go try that thing. And if it works, then, you know, sure as enough, we know what the answer is.
And I don't know that that's for everybody or the right way because it gets you in a lot of trouble. But what I do think you get is very real, tangible data points on what actually happens and what doesn't.
That's why the reason- I definitely think you want to differentiate between throwing stuff at the wall and just trying things versus a good experiment. Testing everything is a good thing.
So if you can write, I mean, the discipline is write down the hypothesis, design the experiment, go do it. That is the way to sort of not be overwhelmed by data.
It's also the way to be cautious, to be sort of realistic in what the outcomes are that you're expecting. One of the nudges that we use an awful lot, and so do people that, you know, we come back and talk to people after a year or so, and it's become sort of one of their favorites, is called Calibrate Confidence.
And it's the idea that you, that most people are overconfident most of the time. And that's not, that's served us well as a species, right? You don't go and try stuff if you don't have some degree of overconfidence.
If you knew everything that you were up against over the last decade, how many different decisions would you have made? Sometimes it's better not to know, you know, it's good. So you've got to balance this a bit, but in general, that it's a good idea to have a good understanding of the state of your own knowledge and that being able to calibrate your accuracy with how well you understand something is actually a pretty good thing.
And so one of the, so, so being able to put a number on, on your knowledge, I'm a hundred percent sure of that, or I'm 90% sure of that, or I'm 75% sure of that. One of the things that that enables is it enables one, you to think, huh, okay, I have to put a number on it.
So you come up with one and you realize as you do that, you sort of generate this curve in your own mind as to where you sit in your state of your own knowledge. But it also allows other people or you to someone else to flip it around and say, 80% confident? Well, why not 100? What's that 20%? What's up with that? And what it does is it forces an explanation and explanations are generative.
You don't just blurt out something. You actually have to sort of sit and think.
And most people, most of the time under explain. So the minute they have to explain them and you can do it to yourself, it really draws out and you generate new knowledge by actually doing it.
You generate a new understanding in yourself and in others. It's a very, very powerful technique.
And it doesn't mean that you become a risk-averse sort of institutionalized ops guy. It means that you are more able to recognize the state of your own knowledge because none of us wanna program in regrets or live a life where we're sort of denying that we regretted a decision, but a regret's okay.
I mean, you know, you want a few false positives, right? You want to be able to do a few things that were kind of wrong. They were just, they were the right call statistically to sort of have enough risk taking in your life.
But starting out with this, at least a knowledge of your own sort of state of knowledge, I think is really powerful. And yeah, you might back off a few things that you otherwise would have plowed into, but you might not.
You might actually have a better perspective on why you're doing something, even though it is risky. So I'm only 60% sure, but this is a real high stakes cool.
If we win this one, we've cracked this nut. So it's, you're able to differentiate between sort of wild ass non-thought out risks and a really calculated, we're doing this because if we win it, we've won everything.
Yeah. Is it, is it, and I'm going to, I'm going to butcher this, whatever I'm trying to explain to you.
I always get metaphors and analogies. I was a math major, so this words are not always my specialty.
But is it fair to say that like, if you're trying to make a decision, data gives you kind of the vector, the direction that you should be looking. And then your intuition, gut experience, the accumulation of what you've had as a professional gives you the ability to pinpoint in and where you actually go.
Like inside that range, it's going to give you a range of a direction. If you have 360 degrees, it's going to say, here this data shows us, this is kind of where we want to be pointed.
And then because I've been doing this for 10 years and I've had these seven experiences, here's the three places inside that range that we want to run tests. It's, it's more, that's the scalpel.
Your intuition is the scalpel kind of, is that, or is it the opposite or is that just a crazy example? Yeah. Well, no, it's a, it's a really good example.
The neuroscientists would say it's exactly the opposite. Antonio Damasio, who says feelings come first, says that this is how it works.
Feelings and intuition will point you to the appropriate space in the decision space to look. And then data actually allows you to really sort of hone in on that, exactly what that analysis outcome is.
Then you would add again that your humanity, your sense of accountability, your risk aversion, your you enables you to actually grapple with the uncertainty and make the decision.
So it's kind of like a data sandwich is what you're describing. Yes, that data sandwich.
I like that. But it's also, I mean, I'm reflecting back on what you were saying about the data about founders and their percentages of ownership and their risks and so forth.
Because I think about, you know, you express one, I haven't seen the study, so I can't have any reflection other than just hearing what you said and then go, huh, really? Like, because my intuition is telling me, I don't know, I'm questioning that conclusion from that data. And it's because of my lived experience, right? Of which companies have been, I think, the most innovative at different eras in time.
GE under Jack Welch and Apple, you know, after Steve came back and Disney under Bob Iger. Those are all, you know, remarkable success stories that where the leader didn't have meaningful ownership percentage.
Does that mean that my experience overrules the data? No, but it does mean that if I was presented with that and I was thinking about actually using that for some purpose, I'd want to dig deeper into it and question it a little bit more to be able to understand that delta. And one of the nudges we do have in our book is around who are the humans in the data? So understanding what the data representation is.
So who are the humans in the data? What are the, where, where is it? And then there's also the question of how are you actually drawing what story out of it? So there could be an alternate example. So we talk about list, which you'd have to believe to believe the opposite.
Is it about the founder's percentage or could it be about the size of the company? I don't know. Is there other alternative answers and other alternative causes to the result?
Yeah, I think this is really good to your point.
And I forget which one of you made it around like what is good data, right?
Because I, and again, now that you say that, I didn't put this piece of information in
not on purpose.
I just didn't add it.
Is that they were talking specifically about early stage companies.
So you're going from a founder who owns 100% to when they lose that percentage, it is often because one, they just got paid. So they went from usually broke to not broke.
And now they, they went from no one can fire me because I'm, you know, one or three people or whatever to now I can be fired and I have something by lose by venture capitalists, like the people who you quote again, like they're showing up and actually firing the CEO. Yes.
Yeah, exactly. So it's like, so, you know, you, you take that and it's a really interesting, it's a really interesting conversation because you say at this, you know, you take that same individual, you know, they're, they're, you know, maybe a co-founder or the, the only founder, they own the majority of the, of the company, they're growing it.
They can't be fired. They can also go broke tomorrow, but they're, you know, and they're growing.
They bring in a big investor. They take a smaller cut.
Now they're, you know, they have some money. They have something to lose.
They have a board that can kick them out right now. All of a sudden they start to play it a little safer because you don't want to make that decision against the grain of the data where the, the board of directors and your VCs can come in and go, the data told you to do this.
You did this. It didn't work.
You're out, right? Where the flip side of everyone that you just named, while 100% true, incredibly innovative, were also late stage, enormous companies that also those guys had big, huge contracts. And there's a lost cost fallacy, I would believe, in the people who gave them those contracts.
And then if I'm playing Bob Iger, $10 million a year, plus a $50 million bonus to run Disney or whatever, that I'm going to kind of give him some leeway in making some decisions and that we're paying this person. So, you know, and I mean, again, I'm just spitballing off the top of my head, but like it is, it's incredibly interesting how that one data point of these were early stage companies versus all companies completely changes the reference of, of what that story can mean.
So that, that actually worked out. I didn't mean it to, that actually worked out pretty well.
I think it worked out quite well. The serendipity of a good conversation.
So, um, um, all right. So I wanted to go back to, uh, cause I, this is a concept I think is, is tremendous.
And, um, um, I just want to flush it out a little more. Uh, the, that concept with the, the, the train, the, um, subway system, we were talking about whose experience are we measuring, right? That to me feels like that, that feels incredibly powerful to me because it feels like just as we just had a slight miscommunication drastically changed our experience with a comment.
Now, again, he just threw this comment out on a podcast. Who knows how real the study is, right? It seemingly felt real good conversation for, for what we're talking about.
But my point is, how do we know
we're measuring the right experience for our business?
How do we know that?
What's that filter system or heuristic, I guess?
I'm thinking.
It's a good question.
So I mean, I think the,
how do you know whether you're measuring the right experience? I think I'm pausing because there's so many different sort of contextual answers to the question.
Yeah. Right.
If you think about in the insurance industry, obviously, you've got the perspective of the insurer and the insured, potentially also the reinsurer. Right.
Because you've got lots of different layers in the industry. And thinking about, let's say you're trying to assess whether a new insurance product is successful.
I'm following your lead and just kind of spitballing here. Yeah, go ahead.
I mean, understanding the first question is, I would say whether you're measuring the right data and the right perspective is to be a little bit more in depth in terms of what the question is. So define success more deeply.
Think about what you mean by that. So there's sort of this question of making sure you're starting with that.
I think there's also when you actually get to the conclusion and you say, yes, this has been a very successful product going through the classic five whys to make sure you're really digging through to the right answer. You know, have you actually gotten to an answer that you actually think is truly there? Because success could be high revenue, you know, for the company.
Success could be low risk for the reinsurer. Success could be customer satisfaction for the, you know, for the, for the insured.
There's a lot of different layers of what success might mean. And that's usually where I think people get caught is that they're not sure exactly what they're asking of, of data.
And so therefore they're not sure which, which experience to rely on. Well, data is generally, it's harder to collect the thing you really want to know about than it is to collect the easy thing.
So I think the first answer is it depends on what your goal is, right? So that's the kind of overarching meta answer. But if you go down a layer from that, there's a couple of things that can happen.
One is that pretty quickly you're in a complex situation like insurance and customer service. You probably pretty quickly find there's some sort of paradox.
There's some sort of dilemma. You can't have the perfect customer service at the same time as keeping costs down, or you can't have the, I mean, in insurance, there's always this background of, we want to have great customer service and settle claims and make everyone happy.
But at the same time, everybody knows that they're on the call with some sort of rationing process, some sort of gotcha kind of process. So I think that being able to very quickly get down to the point that you know why measuring something is hard.
Why is this a hard problem to solve? What's the dilemma that we're constantly going back and forth on? What are the poles of the dilemma? So I think that's an important one. And another one is a, which is more sort of on the complexity side of our house.
But in the decision one, there's a really important concept that again came from Danny Kahneman, which is that we tend to substitute an easy answer for the right answer. So the simplest example is the right question is how happy am I with my life? The easy question is how do I feel right now? and that happens all the time when it comes to data, all the time when it comes to measurement.
So using this nudge of right versus easy, what is the right measure? Like write that down. What do we really want to know? And then what's the easy measure? And actually putting them in front of you.
And because in this world of data gathering by machine, of unconscious stuff, or of using a product like Cogito to capture emotional responses and what have you, and put nudges into, say, a call center with agents in a call center. There are so many things that are easy to measure, not necessarily right measures.
But it doesn't mean you don't do them. It just means that you really need to be much more consciously aware of, on one level, what are the proxies, but then on the other, just what's the right answer? What's the right thing we're trying to get versus what's the easy thing? Yeah, there's two really incredibly relevant problems that you guys addressed in there.
One is, so technically, insurance agents work for carriers. So all the marketing that you'll see out of insurance agents is that we work for you.
That is technically not accurate. Now, there is a term for that.
It's called a broker. But in the United States, 90 plus percent of the property casualty insurance agents are not brokers.
They're agents, which means that technically they work for the carrier. While in order to get paid by the carrier, you have to convince your client to come buy a product from that carrier.
So when you think about that, and that you, you know, have two very large stakeholders who are, you know, in some cases at odds with each other, who's, do you care if they do you want the carrier to be happy? Because if the carrier is happy, you get faster response time, oftentimes higher and more inclusive compensation. You get access to additional products.
You get access to special programs, special pricing, right? If the carrier is happy. But if the carrier is happy, that doesn't necessarily mean that the clients are as happy.
Even if they purchased for you, it doesn't mean they're as happy as they could be. And if you measure straight client happiness and you're only about the client and all that matters is the client relationship, well, oftentimes, and this is very, very common, your relationship with the carriers starts to actually become at odds.
And now who you've actually signed a contract with and technically are responsible to and is at, you're at odds with to the client, which sounds good and feels good. And everyone likes to thump their chest and say, my clients love me.
But if your carriers hate you, then your business is making less money. You oftentimes can't offer as good a product set.
You may not get first pass into different beta programs or specialty programs or specialty lines programs that can ultimately provide either greater access or just better products to your customers. And it is a very, and that's not even to mention, do you care what your, what your employees, how they feel, how they're doing, you know, their metrics, like, you know, or the vendors that you work with or, you know, any referral partners that come in.
So like you have, and we're not alone in this, the, the, the, the kind of principal agent problem in the insurance industry is fairly unique, not wholly unique, but fairly unique. But it is this like, I'm thinking through just the millions of conversations, or probably thousands is technically accurate, of conversations that I've had around this particular problem.
Where do you focus your attention and which relationship is more important to value? And I think that goes all the way down to the baser of where you said, to begin your answer, which was, how do you define success? Like is success maximizing revenue in every way, shape or form, then probably you need your carriers to be happy and focus on the things that make them happy. Do you care more about the relationship you have with your clients, the longevity of those relationships, the ancillary benefits that comes out of having deep, rich, well-built, solid foundation with your clients, which can also be profitable as well, but probably not maximizing profit.
And I think that's going to be different for every agency or every individual business and who those leaders are and who the people are inside them. And that's, that is, there's no, I get, particularly in our industry again, and I'm sure this is the case with others is just, I've spent two, almost two decades of my life.
And this one is as soon as someone starts telling me like, this is the way you should do it. I am like every bell in my, in my being starts to go off and say like, ah, I've been part
of too many different conversations for that to be true.
So it does seem like this is work that very much needs to be done on an individual basis.
And this is maybe where my question is.
My next question is coming from being that I want to be cognizant of your time and respectful to our audience's time. It does vary.
It seems very much like we should be doing this work on an individual basis versus, and not that we can't look at best practice studies and stuff like that. They're probably good benchmarks, but versus relying solely on the benchmarks or the frameworks passed down by a consultant, we need to be maybe working with a consultant to figure out what this is individually.
This is individual work that we need to do because it oftentimes can be very unique to us. Is that a fair assessment? I think that's fair.
And I'd actually make it even more individual in the sense that, well, basically everything's moving to sort of more personalized, but I'd take it, I'd hazard a guess that, you know, when you started out 20 years ago, these relationships were sort of much more one-to-one and not a lot of machines involved. Now, what if 50% of the value of that relationship is now done by machine? And that inside of that, there's an artificial intelligence that's making predictions that sometimes decisions are coupled still with that prediction because they're policies that are put in, they're rules that are set across at scale across the whole client base or whatever.
But if there's agency in that, if there's variability, if there's agency in the way that you're making your judgments and your decisions, this is a much more complex system. Suddenly we're down into self-organizing, we're down into emergent properties, we're down into adaptation, things that you make decisions on within your own discretion and judgment that are fundamentally different than you would have made 20 years ago.
You've got totally different access to information. Rules are different.
There's either more rules or less rules, more decisions, less decisions. They're sort of on a spectrum.
So I think that that's actually the real reason that this is so individual and so unique and why we went to nudges. Because in the end, this is about personal practice.
This is about getting to know what it is that you value and being able to understand how you specifically understand context, how your imagination works, how your creativity works. You're clearly a bit of a status quo buster yourself.
So that's worked really well for you, right? That wrecking
balls worked well, worked really well for me until I turned 40. And then it just didn't work.
And I don't know what it was about that. Some sort of transition.
A little bit of, you know, you can be a kind of young upstart. And a lot of us who are our contrarians in our younger years, that doesn't work as you get older.
People expect the gray hairs. They expect that wisdom.
They're not really as forgiving of those behaviors. And plus, there's a lot of survivor bias.
You're here. It's worked, you're not looking at the people who haven't survived when they were wrecking
balls.
I can, I won't, but I can tell you some names of people who just didn't survive that process. Yeah.
And they're no longer that those kinds of decision makers. So I think it really is this world of personalization exists because the, because we can do it.
i think that it. I think that it's quite wise to think about this as an individual decision bubbling up to your organization's decision, whatever size your organization is.
And you think about other industries that have gone through perhaps somewhat similar major transitions, just looking at the financial services business and I'm thinking management. 20 years ago, it was all about stockbrokers making individual stock calls for their clients.
You'd be wanting to work with one of the big banks because they had the flow. They had the trading desk right there.
Their optimization was around how much am I getting in terms of my trading commissions versus how much money am I making for my clients? It was that kind of a sort of tension. You could go to smaller places, but they wouldn't have the same access to the market timing that you could get at the big banks.
Now, fast forward 20 years, and it's a totally different world. A lot of the portfolios that you're picking are optimized around ETS that are all set up in terms of in large scale research situations.
If you go to the little brokers, they can pick anything you want in the market. Actually, when you go to the big banks now, they're all regulated out of central research organizations of what they're allowed to give to their clients because the regulations have changed.
Still, same sorts of tensions. Am I making money for the bank? Am I making money for my client? But the whole profile of who you are and what you do and what you can offer has changed.
I have friends who've lived through that entire timeline, being the high net worth folks at big banks, and their jobs are completely different from what they were 20, 25 years ago. Now, do they stay there? Well, that's their own individual personal decision and that's fine.
And I would echo, I think what Helen said is our premise in our book and our premise around decision-making is that there isn't one optimizable answer. There isn't one heuristic to follow.
There isn't one process to follow. There isn't a six-step process to make a good decision.
We believe this is truly a practice, which is why we have 50 nudges that help you get better. That's why we call it make better decisions.
It's more like meditation, you know, in terms of practice and thinking about what works for you as an individual inside the sphere of people that you're making decisions with, then it is about some sort of step-by-step process that you can put on boxes and have a framework and do. That can be really unsatisfying for people when we say it.
We truly believe it. We're not having some sort of easy get out of jail free card by saying there isn't a six-step process and we haven't invented it.
We actually just truly believe decision-making is way too complex to be able to have a set process. You have to think about what nudge do I want to use right now in this situation with this topic, with this group of people to make my decisions just a little bit better than they would have been otherwise.
Yeah, I love that. I love it.
Guys, I have thoroughly enjoyed this conversation. The book is Make Better Decisions, How to Improve Your Decision-Making in the Digital Age on Amazon.
I'm assuming the rest of the places. Bookshop.org.
Yep. Local bookshops, wherever you need it.
Where, if people want to connect with you guys in the digital space, where link, you know, what's your spot? Webs LinkedIn, where, where do you want people to go to connect with you? Yeah. Our website is getsonder.com and you can reach us there.
Hello at getsonder.com is an easy email address. You can find us both on LinkedIn.
We also have a, we have a podcast ourselves called artificiality, which is a combination podcast newsletter that we host on Substack. So you can find us there.
That's great. And I'm on TikTok.
Yes, we do. We do.
We do. We do have particular participate in some of the other social medias.
How do you like the TikTok and Instagram and Facebook? TikTok. I set a time limit.
Like it's just any more than five minutes. I'm wasting too much time, but it's just too damn addictive.
I think that the interesting thing about that, about TikTok and Instagram for us is that, you know, we wrote this book coming out of working in a corporate setting and working at workshops, but it's so quickly becomes really applicable for people in the personal lives. So we got a wonderful comment on a TikTok video where someone said, you just explained why my marriage has been in the
shitter for the last five years. Thank you.
And so that was quite an eye-opening moment,
especially, and it was quite encouraging, especially since we're obviously a married
couple. We work together.
We've done this for a long time. It's kind of nice to feel like maybe
actually this could be, you know, this people find applicability in their personal lives too. That's good.
That's tremendous. I mean, I think, I mean, all the concepts we're talking about while applied, obviously to business, you know, I'm sure there's a derivative that applies very much to how you, and, and I really liked the fact that you position it as a practice.
I think that, um, you know, in my own life, I very much try to approach things as a practice versus when I was younger, I think I oftentimes was shooting for the goal, right? I just was, it was, you know, did I get to this thing or did I not get to this thing? And today, I think, hopefully, maybe it's turning 40, which I did recently. you know, I seemingly viewing all changes in our lives as practices, unless it's something
very acute, tends to be a more sustainable and predictable and proactive way of getting stuff done. So I love it.
This has been absolutely wonderful conversation. I wish you guys nothing but success on the book and everything that you're doing.
Obviously, highly recommend this and hope everyone will check it out who's listening.
Guys, I appreciate your time and I hope you have a wonderful day.
It's been fun. Thanks so much.
Cheers. close twice as many deals by this time next week.
Sound impossible? It's not. With the one call close system, you'll stop chasing leads and start closing deals in one call.
This is the exact method we use to close 1,200 clients in under three years during the pandemic. No fluff, no endless follow-ups, just results fast.
Based in behavioral psychology and battle-tested, the one-call-close system eliminates excuses and gets the prospect saying yes more than you ever thought possible. If you're ready to stop losing opportunities and start winning, visit masteroftheclose.com.
That's masteroftheclose.com. Do it today.
I need directions for paying down debt.
Starting route.
Apply for a SoFi personal loan and consolidate your debt into one fixed payment.
Turn right into a positive outlook and get $5,000 to $100,000 as soon as the same day you sign with no fees required.
Got it.
You could get out of high interest credit card debt with a SoFi personal loan. View your rate at SoFi.com slash debt in 60 seconds with no impact to.
Got it. You could get out of high-interest credit card debt with a Silphi personal loan.
View your rate at silphi.com slash debt in 60 seconds with no impact to your credit score.
Loans originated by Silphi Bank and A.
Member FDIC.
Terms and conditions at silphi.com slash debt.
NMLS 696891.