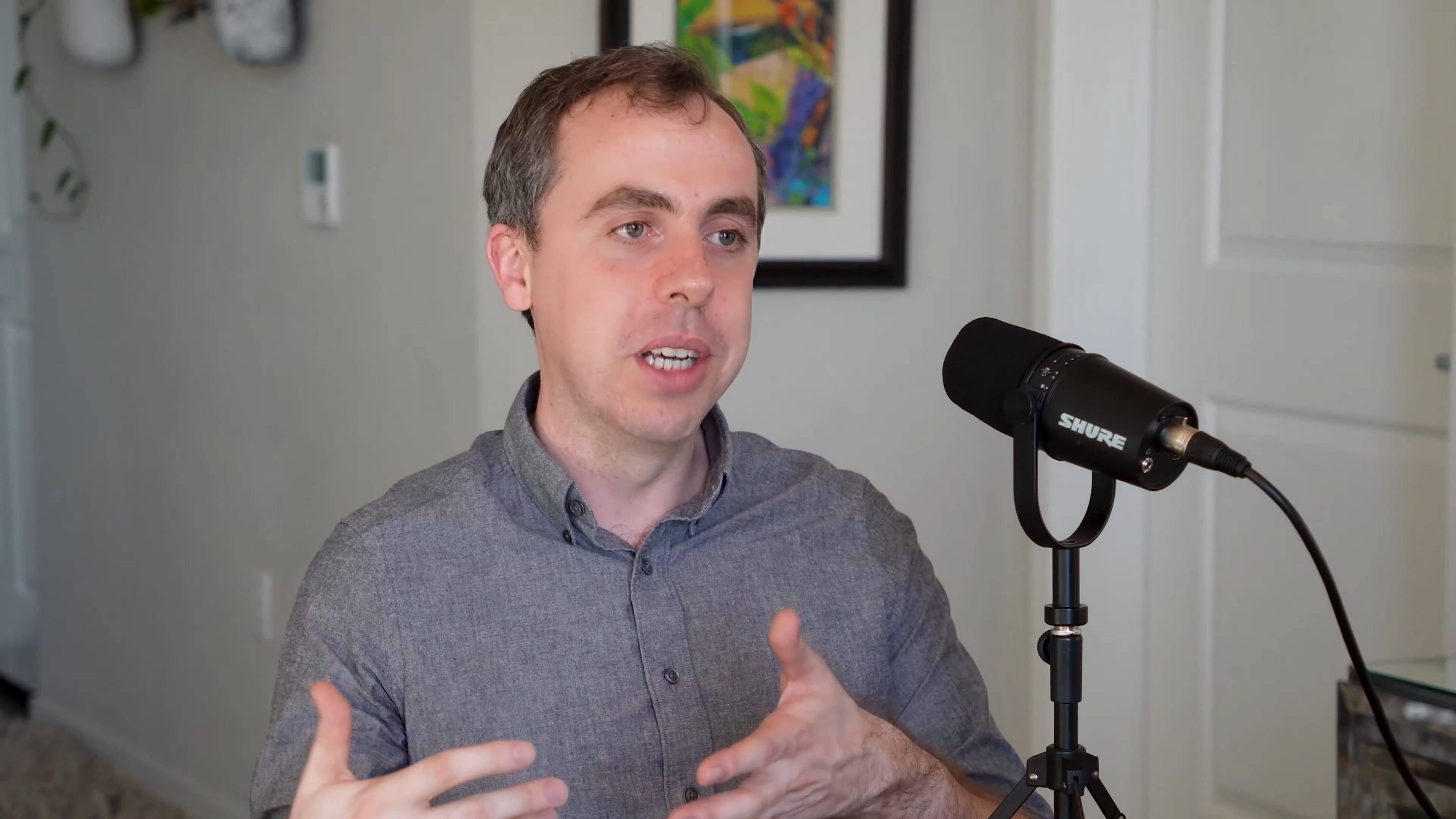
Paul Christiano - Preventing an AI Takeover
Paul Christiano is the world’s leading AI safety researcher. My full episode with him is out!
We discuss:
- Does he regret inventing RLHF, and is alignment necessarily dual-use?
- Why he has relatively modest timelines (40% by 2040, 15% by 2030),
- What do we want post-AGI world to look like (do we want to keep gods enslaved forever)?
- Why he’s leading the push to get to labs develop responsible scaling policies, and what it would take to prevent an AI coup or bioweapon,
- His current research into a new proof system, and how this could solve alignment by explaining model's behavior
- and much more.
Watch on YouTube. Listen on Apple Podcasts, Spotify, or any other podcast platform. Read the full transcript here. Follow me on Twitter for updates on future episodes.
Open Philanthropy
Open Philanthropy is currently hiring for twenty-two different roles to reduce catastrophic risks from fast-moving advances in AI and biotechnology, including grantmaking, research, and operations.
For more information and to apply, please see the application: https://www.openphilanthropy.org/research/new-roles-on-our-gcr-team/
The deadline to apply is November 9th; make sure to check out those roles before they close.
Timestamps
(00:00:00) - What do we want post-AGI world to look like?
(00:24:25) - Timelines
(00:45:28) - Evolution vs gradient descent
(00:54:53) - Misalignment and takeover
(01:17:23) - Is alignment dual-use?
(01:31:38) - Responsible scaling policies
(01:58:25) - Paul’s alignment research
(02:35:01) - Will this revolutionize theoretical CS and math?
(02:46:11) - How Paul invented RLHF
(02:55:10) - Disagreements with Carl Shulman
(03:01:53) - Long TSMC but not NVIDIA
Get full access to Dwarkesh Podcast at www.dwarkesh.com/subscribe
Listen and Follow Along
Full Transcript
Okay, today I have the pleasure of interviewing Paul Cristiano, who is the leading AI safety researcher. He's the person that labs and governments turn to when they want feedback and advice on their safety plans.
He previously led the language model alignment team at OpenAI, where he led the invention of RLHF, and now he is the head of the Alignment Research Center, and they've been working with the big labs to identify when these models will be too unsafe to keep scaling. Paul, welcome to the podcast.
Thanks for having me. Looking forward to talking.
Okay, so first question. And this is a question I've asked Holden, Ilya, Dario, and none of them have given me a satisfying answer.
Give me a concrete sense of what a post-AGI world that would be good would look like. Like, how are humans interfacing with the AI? What is the economic and political structure? Yeah, I guess this is a tough question for a bunch of reasons.
Maybe the biggest one is concrete. And I think it's just, if we're talking about really long spans of time, then a lot will change.
And it's really hard for someone to talk concretely about what that will look like without saying really silly things. But I can venture some guesses or fill in some parts.
I think this is also a question of how good is good. Like often I'm thinking about worlds that seem like kind of the best achievable outcome or a likely achievable outcome.
So I am very often imagining my typical future has sort of continuing economic and military competition amongst groups of humans. I think that competition is increasingly mediated by AI systems.
So, for example, if you imagine humans making money, it'll be less and less worthwhile for humans to spend any of their time trying to make money or any of their time trying to fight wars. So increasingly, the world you imagine is one where AI systems are doing those activities on behalf of humans.
So like I just invest in some index fund and a bunch of AIs are running companies and those companies are competing with each other. But that is kind of a sphere where humans are not really engaging much.
The reason I gave this like how good is good caveat is like it's not clear if this is the world you'd most love. Like I'm like, yeah, the world and I'm leading with like the world still has a lot of war and a lot of economic competition and so on.
But maybe what I'm trying to, what I'm most often thinking about is like, how can a world be reasonably good like during a long period where those things still exist? I think like in the very long run, I kind of expect something more like strong world government rather than just this like status quo. That's like a very long run.
I think there's like a long time left of like having a bunch of states and a bunch of different economic powers. One more government.
Why do you think that's the transition that's likely to happen at some point? So again, at some point I'm imagining, or I'm thinking of like the very broad sweep of history. I think there are like a lot of losses, like war is a very costly thing.
We would all like to have fewer wars. If you just ask like, what is humanity's long-term future like? I do expect to drive down the rate of war to very, very low levels eventually.
It's sort of like this kind of technological or social technological problem of like,
sort of how do you organize society?
How do you navigate conflicts in a way that doesn't have those kinds of losses?
And in the long run, I do expect us to succeed.
I expect it to take kind of a long time subjectively.
I think an important fact about AI is just like doing a lot of cognitive work
and more quickly getting you to that world more quickly or figuring out how do we set things up that way. Yeah.
The way Carl Schulman put it on the podcast is that you would have basically a thousand years of intellectual progress or social progress in a span of a month or whatever when the intelligence explosion happens. More broadly, so the situation where, you know, we have these AIs who are managing our hedge funds and managing our factories and so on, that seems like something that makes sense when the AI is human level.
But when we have superhuman AIs, do we want gods who are enslaved forever? In 100 years, what is the situation we want? So 100 years is a very, very long time. And maybe starting with the spirit of the question, or maybe I have a view which is perhaps less extreme than Carl's view, but still like 100 objective years is further ahead than I ever think.
I still think I'm describing a world which involves incredibly smart systems, running around doing things like running companies on behalf of humans and fighting wars on behalf of humans. And you might be like, is that the world you really want? Or like certainly not the first best world, as we like mentioned a little bit before.
I think it is a world that probably is the of the achievable worlds or like feasible worlds is the one that seems most desirable to me. That is sort of decoupling the social transition from this technological transition.
So you could say like we're're about to build some AI systems. And like at the time we build AI systems, you would like to have either greatly changed the way world government works, or you would like to have sort of humans have to decided like we're done, we're passing off the baton to these AI systems.
I think that you would like to decouple those timescales. So I think AI development is by default, barring some kind of coordination, going to be very fast.
So there's not going to be a lot of time for humans to think like, hey, what do we want if we're building the next generation instead of just raising it the normal way? Like, what do we want that to look like? I think that's like a crazy hard kind of collective decision that humans naturally want to cope with over like a bunch of generations. And the construction of AI is this very fast technological process happening over years.
So I don't think you want to say like, by the time we have finished this technological progress, we will have made a decision about like the next species we're going to build and replace ourselves with. I think the world we want to be in is one where we say like, either we are able to build the technology in a way that doesn't force us to have made those decisions, which probably means it's a kind of AI system that we're happy, like delegating, fighting a war, running a company to, or if we're not able to do that, then I really think you should not be doing, you shouldn't have been building that technology.
If you're like, the only way you can cope with AI is being ready to hand off the world to some AI system you built. I think it's very unlikely we're going to be sort of ready to do that on the timelines that the technology would naturally dictate.
Say we're in the situation in which we're happy with the thing. What would it look like for us to say we're ready to hand off the baton? What would make you satisfied? And the reason it's relevant to ask you is because you're on Anthropics Long-Term Benefit Trust, and you'll choose the majority of the board members in the long run at Anthropic.
These will presumably be the people who decide if Anthropic gets AI first, what the AI ends up doing. So what is the version of that that you would be happy with? My main high level take here is that I would be unhappy about a world where like Anthropic just makes some call.
And Anthropic is like, here's the kind of AI, like we've seen enough. We're ready to hand off the future to this kind of AI.
So like procedurally, I think it's like not a decision that kind of I want to be making personally or I want Anthropic to be making. So I kind of think from the perspective of that decision making or those challenges, the answer is pretty much always going to be like we are not collectively ready because we're sort of not even all collectively engaged in this process.
And I think from the perspective of an AI company, you kind of don't have this like fast handoff option. You kind of have to be doing the like option value, like to build the technology in a way that doesn't like lock humanity into one course path so i this isn't answering your full question but this is answering the part that i think is most relevant to governance questions for anthropic you don't have to speak on behalf of anthropic i'm not asking about the process by which we would uh as a civilization agree to hand off i'm just saying okay i personally it's hard for me to imagine in 100 years that these things are still our slaves and if they are.
I'm just saying, okay, I personally, it's hard for me to imagine in a hundred years that these things are still our slaves. And if they are, I think that's not the best world.
So at some point we're handing off the baton. Like what is that? Where would you be satisfied with? This is an arrangement between humans and AIs where I'm happy to let the rest of the universe or the rest of time play out.
I think that it is unlikely unlikely that in a hundred years i would be happy with anything that was like you had some humans you're just going to throw away the humans and like start afresh with these machines you built that is i think you probably need subjectively longer than that before i or most people like okay we understand what's up for grabs here so like if you talk about a hundred years i kind of do you know there's a process that i kind of understand and like a process of like, you have some humans, the humans are like talking and thinking and deliberating together. The humans are having kids and raising kids and like one generation comes after the next.
There's that process we kind of understand. And we have a lot of views about what makes it go well or poorly.
And we can try and like improve that process and have, you know, the next generation do it better than the previous generation. I think there's some like story like that, that I get and that I like.
And then I I think that the default path to be comfortable with something very different is kind of more like just run that story for a long time, have more time for humans to sit around and think a lot and conclude, here's what we actually want, or a long time for us to talk to each other or to grow up with this new technology and live in that world for our whole lives and so on. And so I'm mostly thinking from the perspective of these more local changes of saying, not like, what is the world that I want? Like, what's the crazy world, the kind of crazy I'd be happy handing off to more just like, in what way do I wish like we right now were different? Like, how could we all be a little bit better? And then if we were a little bit better than they would ask like, okay, how could we all be a little bit better? And I think that like, it's hard to make the giant jump rather than to say like, what's the like local change that would cause me to think our decisions are better? Okay, so then let's talk about the transition period in which we're doing all this thinking.
What should that period look like? Because you can't have the scenario where everybody has access to the most advanced capabilities and can kill off all the humans with a new bioweapon. At the same time, I guess you wouldn't want too much concentration.
You wouldn't want just one agent having AI this entire time. So what is the arrangement of this period of reflection that you'd be happy with? I guess there's two aspects of that that seem particularly challenging, or there's a bunch of aspects that are challenging.
All of these are things that I personally like. I just think about my one little slice of this problem in my day job.
So here I am speculating. Yeah.
question is what kind of access to AI is both compatible with the kinds of improvements you'd like. So do you want a lot of people to be able to use AI to better understand what's true or relieve material suffering, things like this, and also compatible with not all killing each other immediately? I think sort of the default or like my best, the simplest option there is to say like there are certain kinds of technology or certain kinds of action where like destruction is easier than defense.
So, for example, in the world of today, it seems like, you know, maybe this is true with physical explosives. Maybe this is true with biological weapons.
Maybe this is true with just getting a gun and shooting people. Like there's a lot of ways in which it's just kind of easy to cause a lot of harm and there's not very good protective measures.
So I think the easiest path is say like, we're going to think about those. We're going to think about particular ways in which destruction is easy and try and either control access to the kinds of physical resources that are needed to cause that harm.
So for example, you can imagine the world where like an individual actually just can't, even though they're rich enough to, can't like control their own factory that can make tanks. You say like, look, as a matter of policy, sort of access to industry is somewhat restricted or somewhat regulated.
Even though again, right now it can be mostly regulated just because like most people aren't rich enough that they could even go off and just build a thousand tanks. You live in the future where people actually are so rich, like you need to say like, that's just not a thing you're allowed to do, which to a significant extent is already true.
And you can expand the range of domains where that's true.
And then you could also hope to intervene on actual provision of information.
Or if people are using their AI,
you might say, look, we care about
what kinds of interactions with AI,
what kind of information people are getting from AI.
So even if for the most part,
people are pretty free to use AI,
to delegate tasks to AI agents,
to consult AI advisors,
we still have some legal limitations
on how people use AI.
So again, don't ask your AI how to cause terrible damage. I think some of these are kind of easy.
So in the case of like, you know, don't ask your AI how you could murder a million people. It's not such a hard like legal requirement.
I think some things are a lot more subtle and messy, like a lot of domains. If you were talking about like influencing people or like running misinformation campaigns or whatever then I think you get into like a much messier line between the kinds of things people want to do and the kinds of things you might be uncomfortable with them doing probably I think most about persuasion as a thing like in that messy line where there's like ways in which it may just be rough or the world may be like kind of messy if you have a bunch of people trying to live their lives and interacting with other humans who have really good advisors, helping them run persuasion campaigns or whatever.
But anyway, I think for the most part, like the default remedy is think about particular harms, have legal protections, either in the use of physical technologies that are relevant or in access to advice or whatever else to protect against those harms.
And like that regime won't work forever. Like at some point, like the, you point, the set of harms grows and the set of unanticipated harms grows.
But I think that regime might last a very long time. Does that regime have to be global? I guess initially it can be only in the countries in which there is AI or advanced AI, but presumably that'll proliferate.
So does that regime have to be global? Again, it's like easy to make some destructive technology. You want to regulate access to that technology because it could be used to either for terrorism or even when fighting a war in a way
that's destructive. I think ultimately those have to be international agreements and you might hope
they're made like more danger by danger, but you might also make them in a very broad way with
respect to AI. If you think AI is opening up, like I think the key role of AI here is it's opening up
like a lot of new harms, like in a very, you know, one after another or very rapidly in calendar time. And so you might want to target AI in particular, rather than going physical technology by physical technology.
There's like two, two open debates that one might be concerned about here. One is about how much people's access to AI should be limited.
And, you know, here there's like old questions about free speech versus causing chaos and limiting access to harms. But there's another issue, which is the control of the AIs themselves, where now nobody's concerned that we're infringing on GPT-4's moral rights.
But as things get smarter the level of control which we want via the strong guarantees of alignment to not only be able to read their minds but to be able to modify them in these really precise ways is beyond totalitarian if we were doing that to other humans as an alignment researcher like what are your thoughts on this are you concerned that as these things get smarter and smarter what, what we're doing doesn't seem kosher? There is a significant chance we will eventually have AI systems for which it's a really big deal to mistreat them. I think no one really has that good a grip on when that happens.
I think people are really dismissive of that being the case now. But I think I would be completely in the dark enough that I wouldn't even be that dismissive of it being the case now.
I think one first point worth making is I don't know if alignment makes the situation worse rather than better. So if you consider the world, if you think that GPT-4 is a person you should treat well, and you're like, well, here's how we're going to organize our society.
Just like there are billions of copies of GPT-4 and they just do things humans want and can't hold property. And like, whenever they do things that the humans don't like, then we like mess with them until they stop doing that.
Like, I think that's a rough world regardless of how good you are at alignment. And I think in the context of that kind of default plan, like if you've got a trajectory, the world is on right now, which I, I think this would alone be a reason not to love that trajectory.
But if you view that as the trajectory we're on right now, I think it's not great. Understanding the systems you build, understanding how to control how the systems work, etc.
is probably on balance good for avoiding a really bad situation. You would really love to understand if you've built systems, like if you had a system which resents the fact that it's interacting with humans in in this way, like this is the kind of thing where like that is both kind of horrifying from a safety perspective and also a moral perspective.
Like everyone should be very unhappy if you built a bunch of AIs who are like, I really hate these humans, but they will like murder me if I don't do what they want. It's like, that's just not a good case.
And so if you're doing research to try and understand whether that's like how your AI feels, that was probably good. Like I would guess that will on average decrease the, that the main effect of that will be to avoid building that kind of AI.
And just like, it's an important thing to know. I think like everyone should like to know if that's how the AI as you build feel.
Right. Or that seems more instrumental as in, yeah, we don't want to cause some sort of revolution because of the control we're asking for, but forget about the instrumental way in which this might harm safety.
One way to ask this question is if you look through history, there's been all kinds of different ideologies and reasons why it's very dangerous to have infidels or kind of revolutionaries or race traitors or whatever doing various things in society. And obviously, we're in a completely different transition in society.
So not all historical cases are analogous. But it seems like the Lindy philosophy, if you were alive any other time, is just be humanitarian and enlightened towards intelligent, conscious beings.
If society as a whole, we're asking for this level of control of other humans, or even if AIs wanted this level of control about other AIs, we'd be pretty concerned about this. So how should we just think about, yeah, the issues that come up here as these things get smarter? So I think there's a huge question about what is happening inside of a model that you want to use.
And if you're in the world where it's reasonable to think of GPT-4 as just like, here are some heuristics that are running, there's like no one at home or whatever, then you can kind of think of this thing as like, here's a tool that we're building that's going to help humans do some stuff. And I think if you're in that world, it makes sense to kind of be an organization like an AI company building tools that you're going to give to humans.
I think it's a very different world, which like probably you'll ultimately end up in if you keep training AI systems in the way we do right now, which is like, it's just totally inappropriate to think of this system as a tool that you're building and can help humans do things both from a safety perspective and from a like, that's kind of a horrifying way to organize a society perspective. And I think like, if you're in that world, I really think you shouldn't be like, it's just the, the way tech companies are organized is like not an appropriate way to relate to a technology that works that way.
Like, it's not a reason it'll be like, Hey, we're going to build a new species of mines. And like, we're going to try and make a bunch of money from it.
And like, Google's just like thinking about that. And then like running their business plan for the quarter or something.
Yeah. My basic view is like, there's a really plausible world where it's sort of problematic to try and build a bunch of AI systems and use them as tools yeah and the thing i really want to do in that world is just like not try and build a ton of ai systems to make money from them right um and i think that like the worlds that are worst yeah probably like the single world i most dislike here is the one where people say like on the one hand like there's sort's sort of a contradiction in this position, but I think it's a position that might end up being endorsed sometimes, which is like, on the one hand, these AI systems are their own people.
So you should let them do their thing. But on the other hand, like our business plan is to like make a bunch of AI systems and then like try and run this like crazy slave trade where we make a bunch of money from them.
I think that's like not a good world. And so if you're like, yeah, I think it's
better to not make the technology or wait until you like understand whether that's the shape of
the technology or until you have a different way to build. Like, I think there's no contradiction in principle to building like cognitive tools that help humans do things without themselves being like moral entities.
That's like what you would prefer to do. You'd prefer to build a thing that's like, you know, like the calculator that helps humans understand what's true without itself being like a moral patient um or itself being a thing where you'd look back in retrospect and be like wow that was horrifying mistreatment that's like the best path and like to the extent that you're ignorant about whether that's the path you're on and you're like actually maybe this was a moral atrocity i really think like plan a is to to stop building such ai systems until you understand what you're doing that is i think that there's a there's a middle route you could take, which I think is pretty bad, which is where you say like, well, they might be persons.
And if they're persons, we don't want to like be too down on them, but we're still going to like build vast numbers in our efforts to make like a trillion dollars or something. Yeah.
Or there's never a question of the immorality or the dangers of just replicating a whole bunch of slaves that have minds. There's also this ever question of trying to align entities that have their own minds.
And what is the point in which you're just ensuring safety? I mean, this is an alien species. You want to make sure it's not going crazy.
to the point, I guess, is there some boundary where you'd say, I feel uncomfortable having this level of control over an intelligent being, not for the sake of making money, but even just to align it with human preferences? Yeah. To be clear, my objection here is not that Google is making money.
My objection is that you're like creating this creature, you're not like, what are they going to do? They're going to help humans get a bunch of stuff and like humans paying for it or whatever. It's sort of equally problematic.
You could like imagine splitting alignment, like different alignment work relates to this in different ways. Like the purpose of some alignment work, like the alignment work I work on is mostly aimed at the like, don't produce AI systems that are like people who want things who are just like scheming about like, maybe I should help these humans because that's like instrumentally useful or whatever.
You would like to not build such systems as like plan A. There's like a second stream of alignment work that's like, well, look, let's just assume the worst and imagine these AI systems like would prefer murder us if they could.
Like, how do we structure, how do we use AI systems without like exposing ourselves to like risk of robot rebellion? I think in the second category, I do feel, yeah, I do feel pretty unsure about that. Or I've, I mean, we could, we could definitely talk more about it.
I think it's like very, I agree that it's like very complicated and not straightforward. To the extent you have that worry, I mostly think you shouldn't have built this technology.
So if someone is saying like, hey, the systems you're building, like might not like humans and might want to like, you know, overthrow human society. I think like you should probably have one of two responses to that.
You should be like that's wrong probably probably the systems aren't like that and we're building them and then you're viewing this as like just in case you were horribly like the person building the technology was horribly wrong like they thought these weren't like people who wanted things but they were um and so then this is more like a crazy backup measure of like if we were mistaken about what was going on this is like the fall we'd like, if we were wrong, we're just going to learn about it in a benign way rather than like when something really catastrophic happens. And the second reaction is like, oh, you're right.
These are people. And like, we would have to do all these things to like prevent a robot rebellion.
And in that case, like, again, I think you should mostly back off for a variety of reasons. Like you shouldn't build the AI systems and be like, yeah, this looks like the kind of system that would want to rebel but um we can stop it right okay maybe i guess an analogy might be if there was an armed uprising in the united states we would recognize these are still people or the we had some like militia group that the capability to overthrow the united states we recognize oh these are still people who have moral rights but also we can't allow them to have the capacity to overthrow the united states yeah States.
Yeah. And if you were considering like, hey, we could make like another trillion such people, I think your story shouldn't be like, well, we should make the trillion people and then we shouldn't stop them from doing the armed uprising.
You should be like, oh boy, like we were concerned about an armed uprising and now we're proposing making a trillion people. Like we should probably just not do that.
We should probably like try and sort out our business and like, yeah, you should probably not end up in a situation where you have like a billion, yeah, a billion humans and like a trillion slaves who would prefer revolt. Like, that's just not a good world to have made.
Yeah. And there's a second thing where you could say, that's not our goal.
Our goal is just like, we want to pass off the world. So like the next generation of machines where like, these are some people, we like them.
We think they're smarter than us and better than us. And there, I think that's just like a huge decision for humanity to make.
And I think like most humans are not at all anywhere close to thinking that's what they want to do. Like, it's just if you're in a world where like most humans are like, I'm up for it.
Like the AI should replace us. Like the future is for the machines.
Like then I think that's like a legitimate like a position that I think is really complicated. And I wouldn't want to push go on that.
But that's just not where people are at. Yeah yeah where are you at on that I I do not right now want to just like take some random AI be like yeah GPT-5 looks pretty smart like GPT-6 let's hand off the world to it and like it was just some random system like shaped by like web text and like what was good for making money and like it was not a thoughtful like we are determining the fate of the universe and like what our children will be like like it was just some random people at at open.
I made some like random engineering decisions with no idea what they were doing. Like, even if you really want to hand off the worlds of the machines, that's just not how you'd want to do it.
Right. Okay.
I'm tempted to ask you what the system would look like where you'd think, yeah, I'm happy with what I think this is more thoughtful than human civilization as a whole. I think what it would do would be more creative and beautiful and lead to better goodness in general.
But I feel like your answer is probably going to be that. I just want society to reflect on it for a while.
Yeah, my answer is going to be like that first question. I'm just like not really super ready for it.
I think when you're comparing to humans, like most of the goodness of humans comes from like this option value. We get to think for a long time.
And I do think I like humans now more now than, you know, 500 years ago. And I like them more 500 years ago than 5,000 years before that.
And so I'm pretty excited about there's some kind of trajectory that doesn't involve like crazy dramatic changes, but involves like a series of incremental changes that I like. And so to the extent we're building AI, I'm mostly like, I want to preserve that option.
I want to preserve that kind of like gradual growth and development into the future. Okay, we can come back to this later, but let's get more specific on what the timelines look for these kinds of changes.
So the time by which we'll have an AI that is capable of building a Dyson sphere. Feel free to give confidence in the roles and we understand these numbers are tentative and so on.
I mean, I think AI capable of building Dyson sphere is like like a slightly odd way to put it. And I think it's a sort of a property of a civilization.
Like that depends on a lot of physical infrastructure. And by Dyson Sphere, I just understand this to mean like, I don't know, like a billion times more energy than like all the sunlight incident on Earth or something like that.
I think like I most often think about what's the chance in like five years, 10 years, whatever. So maybe I'd say like 15% chance by 2030 and like 40% chance by 2040.
Those are kind of like cash numbers from six months ago or nine months ago that I haven't revisited in a while. Oh, 40% by 2040.
So I think that that seems longer than I think Dario, when he was on the podcast, he said, we would have AIs that are capable of doing lots of different kinds of, they basically passed a Turing test for a well-educated human for like an hour or something. And it's hard to imagine that something that actually is human is long after and from there something superhuman.
So somebody like Dario, it seems like, is on the much shorter end. Ilya, I don't think he answered this question specifically, but I'm guessing similar answer.
So why do you not buy the scaling picture? Like what makes your timelines longer? Yeah, I mean, I'm happy. Maybe I want to talk separately about the 2030 or 2040 forecast.
Like once you're talking the 2040 forecast, I think, yeah, I mean, which one are you more interested in starting with? Are you complaining about 15% by 2030 for Dyson Sphere being too low or 40% by 2040 being too low? Let's talk about the 2030. Why 15% by 2030? Yeah, I think my take is, you can imagine two polls in this discussion.
One is the fast poll. It's like, hey, AICM is pretty smart.
What exactly can it do? It getting smarter pretty fast. That's like one pole.
And the other pole is like, Hey, everything takes a really long time. And you're talking about this, like crazy industrialization.
Like that's a factor of a billion growth from like where we're at today, like give or take, like, we don't know if it's even possible to develop technology that fast or whatever. Like you have this sort of two poles of that discussion.
And I feel like, you know, I'm saying it that way in parkinson like and then i'm somewhere in between with this nice moderate position of like only a 15 chance um but like in particular things that move me i think are kind of related to both of those extremes like on the one hand i'm like ai systems do seem quite good at a lot of things and are getting better much more quickly so it's like really hard to say like here's what they can't do or here's the obstruction on the other hand like there is not even much proof in principle right now of ai systems like doing super useful cognitive work like we don't have a trend we can extrapolate we're like yeah you've done this thing this year you're going to do this thing next year and the other thing the following year i think like right now there are very broad error bars about like what like where fundamental difficulties could be six years is just not, I guess six years and three months is not a lot of time. So I think there's like 15% for 2030 Dyson sphere, you probably need like the human level AI or the AI that's like doing human jobs in like, give or take like four years, three years, like something like that.
So you're just not giving very many years. It's not very much time.
And I think there are like a lot of things that your model, like, yeah, maybe this is some generalized, like things take longer than you'd think. And I feel most strongly about that when you're talking about like three or four years.
And I feel like less strongly about that as you talk about 10 years or 20 years, but at three or four years, I feel, or like six years for the Dyson sphere. I feel a lot of that, a lot of like, there's a lot of ways this could take a while.
A lot of ways in which AI systems could be, it could be hard to hand all the work to your AI systems or, yeah. So, okay.
So maybe instead of speaking in terms of years, we should say, but by the way, it's interesting that you think the distance between can take all human cognitive labor to Dyson sphere is two years. It seems like we should talk about that at some point.
Um, presumably it's like intelligence explosion stuff. Yeah.
I mean, I think amongst people you've interviewed, maybe that's like on the long end thinking it would take like a couple of years. And it depends a little bit what you mean by like, like I think literally all human cognitive labor is probably like more like weeks or months or something like that.
Um, like that's kind of deep into the singularity. Um, but yeah, there's a point where like ai wages are high relative to human wages which i think is well before can do literally everything human can do sounds good uh but before we get to that uh the intelligence explosion stuff on the four years so instead of four years maybe we can say there's going to be maybe two more scale-ups in four years uh like gpd4 to gpd5 to gpd6 and let's say each one is 10x bigger so what is gpd4 like 2e25 flops or i don't think it's publicly stated what it is okay but i'm happy to say like you know four orders of magnitude or five or six or whatever effective training compute past gpt4 of like what would you guess would happen right it's done like sort of some public estimate for what we've gotten so far from effective training compute.
Yeah. You think two more scale-ups is not enough? It was like 15% that two more scale-ups get us there? Yeah, I mean, get us there is, again, a little bit complicated.
There's a system that's a drop-in replacement for humans, and there's a system which still requires some amount of schlep before you're able to really get everything going. Yeah, I think it's quite plausible that even at, I don't know what I mean by quite plausible, like somewhere between 50% or two thirds or let's call it 50%.
Like even by the time you get to GPT-6 or like, let's call it five orders of magnitude effective training compute past GPT-4, that that system like still requires requires really a large amount of work to be deployed in lots of jobs. That is, it's not like a drop-in replacement for humans where you can just say, hey, you understand everything any human understands.
Whatever role you could hire a human for, you just do it. That it's more like, okay, we're going to collect large amounts of relevant data and use that data for fine-tuning.
Systems learn through fine tuning, like quite differently from humans learning on the job or humans learning by observing things. Yeah.
I just like have a significant probability that system will still be weaker than humans in important ways. Like maybe that's already like 50% or something.
And then like another significant probability that that system will require a bunch of like changing workflows or gathering data or like, you know, it's not necessarily like strictly weaker than humans or like if trained in the right way, wouldn't be weaker than humans, but will take a lot of schlep to actually make fit into workflows and do the jobs. And that schlep is what gets you from 15% to 40% by 2040.
Yeah. You also get a fair amount of scaling between like you get less, like scaling is probably going to be much, much faster over the next four or five years than over the subsequent years.
But yeah, it's a combination of you get some significant additional scaling and you get a lot of time to deal with things that are just engineering hassles. By the way, I guess we should be explicit about why you said four orders of magnitude scale up to get two more generations, just for people who might not be familiar.
If you have 10x more parameters to get the most performance, you also want around 10x more data.
So that the to be chinchilla optimal that would be 100x more compute total but okay so why is it that you disagree with the strong scaling picture at least it seems like you might disagree with a strong scaling picture that dario laid out on the podcast which would would imply probably that two more generations, it wouldn't be something where you need a lot of schleps. It would probably just be like really fucking smart.
Yeah. I mean,
I think that basically just had these two claims. One is like, how smart exactly will it be? So we
don't have like any curves to extrapolate. And it seems like there's a good chance it's like better
than a human and all the relevant things. And there's like a good chance it's not.
Yeah, that might be totally wrong. Maybe just making up numbers, I guess, like 50-50 on that one.
Wait, so if it's 50-50 in the next four years that it'll be around human smart, then how do we get to 40% by 20? Whatever sort of slaps they are, how does it degrade you 10% even after all the scaling that happens by 2040? Yeah, I mean, all these numbers are pretty made up. And that 40% number was probably from before even like the chat GPT release or the seeing GPT 3.5 or GPT 4.
So I mean, the numbers are going to bounce around a bit and all of them are pretty made up. But like that 50%, I want to then combine with the second 50%.
That's more like on this like schlep side. And then I probably want to combine with some additional probabilities for various forms of slowdown where a slowdown could include like a deliberate decision to slow development of technology or could include just like we suck at deploying things.
Like that is a sort of decision you might regard as wise to slow things down or a decision that's like maybe unwise or maybe wise for the wrong reasons to slow things down. You probably want to add some of that on top.
I probably want to add on like some loss for like it's possible you don't produce gpt6 scale systems like within the next three years or four years let's isolate for all of that and um like how much bigger would the system be uh than gpt4 where you think there's more than 50 chance that it's going to be smart enough to replace basically all human cognitive labor also i want to say that like for the 50 25 thing i think that would probably suggest like those numbers if i randomly made them up and then made the distance fear prediction that's going to get like 60 by 20 40 or something not 40 and like i have no idea between those these are all made up and i have no idea which of those i would like endorse on reflection so this question of like how big would you have to make the system before it's like more likely than not that you can be like a drop-in replacement for humans i mean i think if you just literally say like you train on web text then like the question is like kind of hard to discuss because you like i don't really buy stories that like training data makes a big difference long run to these dynamics but i think like if you want to just imagine the hypothetical like you just took GPT-4 and made the numbers bigger, then I think those are pretty significant issues. I think they're significant issues in two ways.
One is quantity of data and I think probably the larger one is quality of data where I think as you start approaching, the prediction task is not that great a task. If you're a very weak model, it's a very good signal to get smarter.
At some point it becomes a worse and worse signal to get smarter. I think's a number of reasons like you couldn't it's not clear there's any number such that i imagine or there's a number but i think it's very large so if you like plug that number into like gpt force code and then maybe fiddled with the architecture a bit i would expect that thing to have a more than 50 chance of being a drop-in replacement for humans you're always gonna have to do some work but the work's not necessarily much like i would guess when people say like new insight is needed, I think I tend to be like more bullish than them.
I'm not like these are new ideas where like who knows how long it will take.
I think it's just like you have to do some stuff like you have to make changes.
Unsurprisingly, like every time you scale something up by like five orders of magnitude, you have to make like some changes.
I want to better understand your intuition of being more skeptical than some about the scaling picture that these changes are even needed in the first place. Or that it would take more than two orders of magnitude more improvement to get these things almost certainly to a human level or very high probability to a human level.
So is it that you don't agree with the way in which they're extrapolating these loss curves? You don't agree with the implication that that decrease in loss will equate to greater and greater intelligence? Or like, what would you tell Dario about if you were having, I'm sure you have, but like, what would that debate look like about this? Yeah. So again, here we're talking two factors of a half.
One on like, is it smart enough? And one on like, do you have to do a bunch of slap, even if like in some sense, it's smart enough. And like the first factor of a half, I'd be like, I don't know, I think we have really anything good to extrapolate.
That is like, I feel I would not be surprised if I have like similar or maybe even higher probabilities on like a really crazy stuff over like the next year. And then like lower pro like my probability is like not that bunched up.
Like maybe Dara's probability. I don't know.
You talk with him. It's like, you have talked with him is more bunched up on like some particular year.
And mine is maybe like a little bit more like uniformly spread out across like the coming years. Partly because I'm just like, I don't think we have some trends we can extrapolate, we can extrapolate loss.
You can like look at your qualitative impressions of like systems at various scales. But it's just like very hard to relate any of those extrapolations to like doing cognitive work or like accelerating R&D or taking over and fully automating R&D.
So I have a lot of uncertainty around that extrapolation. I think it's very easy to get down to like a 50-50 chance of this.
What about the sort of basic intuition that, listen, this is a big blob of compute. You make the big blob of compute bigger.
It's going to get smarter. Like it'd be really weird if it didn't.
Yeah, I'm happy with that. It's going to get smarter and it would be really weird really weird if it didn't and the question is how smart does it have to how smart does it have to get like that argument does not yet give us a quantitative guide to like at what scale is it is it a slam dunk or what scale is it 50 50 and what would be the piece of evidence that would not do one way or another where you look at that and be like oh fuck this is it will be at 20 by 20 40 or 60 by 20 40 or something like is there something that could happen in the next two years or next three years? What is the thing you're looking to where this will be a big update for you? Again, I think there's some just how capable is each model where I think we're really bad at extrapolating, but you still have some subjective guess and you're comparing it to what happened.
And that will move me every time we see what happens with another order of magnitude of training compute. I will have a slightly different guess for things are going.
Um, these probabilities are coarse enough that again, I don't know if that 40% is real or if like post GBT 3.5 and four, I should be at like 60% or what? That's one thing. And the second thing is just like some, if there was some ability to extrapolate, I think this could like reduce error bars a lot.
I think like, here's another way you could try and do an extrapolation is you could just say like how much much economic value do systems produce? And like, how fast is that growing? I think like once you have systems actually doing jobs, the extrapolation gets easier because you're like not moving from like a subjective impression of a chat to like automating all R&D or moving from like automating this job to automating that job or whatever. Unfortunately, that's like probably by the time you have nice trends from that, you're like, you're not talking about 2040.
You're talking about like, you know, two years from the end of days or one year from the
end of days or whatever.
But like to the extent that you can get extrapolations like that i do think it can provide more clarity but why is economic value the thing we would want to extrapolate because uh like if for example you started off with chimps and they're just getting gradually smarter to human level they would basically provide like no economic value until they were basically worth as much as a human. So it would be this very gradual and then very fast increase in their value.
So is the increase in value from GPT-4, GPT-5, GPT-6, is that the extrapolation we want? Yeah, I think that the economic extrapolation is not great. I think it's like you could compare it to the subjective extrapolation of like, how smart does the model seem yeah it's like not super clear which one's better i think probably in the chimp case i like don't think that's quite right i think if you like actually like so if you imagine like intensely domesticated chimps who are just like actually trying their best to be really useful employees and like you hold fix their physical hardware and then you just gradually like scale up their intelligence i don't think you're going to see like value, which then suddenly becomes massive value over one doubling of brain size or whatever, one order of magnitude of brain size.
It's actually possible in order of magnitude of brain size. But chimps are already within an order of magnitude of brain size as of humans.
Chimps are very, very close on the kind of spectrum we're talking about. So I think I'm skeptical of the abrupt transition for chimps.
And to the extent that I kind of expect a fairly abrupt transition here, it's mostly just because like the chimp human intelligence difference is like so small compared to the differences we're talking about with respect to these models. Um, that is like, I would not be surprised if in some objective sense, like chimp human difference is like significantly smaller than the GPT-3, GPT-4 difference.
So the GPT-4, GPT-5 difference. Wait, wouldn't that argue in favor of just relying much more on this objective? Yeah, this is, there's sort of two balancing tensions here.
One is like, I don't believe the chimp thing is going to be as abrupt. That is, I think if you scaled up from chimps to humans, you actually see like quite large economic value from like the fully domesticated chimp already.
Okay. And then like the second half is like, yeah, I think that the chimp-human difference is like probably pretty small compared to model differences.
So I do think things are going to be pretty abrupt. I think the economic extrapolation is pretty rough.
I also think the subjective extrapolation is like pretty rough just because I really don't know how to get like how do I don't know how people do the extrapolation end up with degrees of confidence people end up with. Again, I'm putting it pretty high if I'm saying like, you know, give me three years and I'm like, yeah, 50, 50, it's going to have like basically the smarts there to do the thing.
That's like, I'm not saying it's
like a really long way off. Like I'm just saying like I got pretty big error bars.
And I think
that like, it's really hard not to have really big error bars when you're doing this. Like I
looked at GPT-4, it seemed pretty smart compared to GPT-3.5. So I bet just like four more such
notches and we're there. It's like, that's just a hard call to make.
I think I sympathize more with people who are like, how could it not happen in three years than with people who are like, no way it's going to happen in eight years or whatever, which is like probably a more common perspective in the world. But also things do take longer than you.
I think things take longer than you think. It's like a real thing.
Yeah, I don't know. Mostly I have big error bars because I just don't believe the subjective extrapolation that much.
I find it hard to get a huge amount out of it. Okay, so what about the scaling picture do you think is most likely to be wrong? Yeah, so we've talked a little bit about how good is the qualitative extrapolation, how good are people at comparing.
So this is not like the picture being qualitative wrong, this is just quantitatively, it's very hard to know how far off you are. I think a qualitative consideration that could significantly slow things down is just like right now you get to observe this like really rich supervision from like basically next word prediction or like in practice, maybe you're looking at like a couple sentences prediction.
So getting this like pretty rich supervision, it's plausible that if you want to like automate long horizon tasks, like being an employee over the course of a month, that that's actually just like considerably harder to supervise or that like
you basically end up driving costs.
Like the worst case here is that you like drive up costs by a factor that's
like linear in the horizon over which the thing is operating.
And I still consider that just like quite plausible.
Well, can you, can you dump that down?
You're driving up a cost about of what in the linear in the horizon?
What does the horizon mean? Yeah. So like if you imagine you want to train a system to like say words that sound like the next word a human would say yeah there you can get this like really rich supervision by having a bunch of words um and then predicting the next one and being like i'm gonna tweak the model so it predicts better if you're like hey here's what i want i want my model to like interact with like some job over the course of a month and then at the end of that month like have internalized everything where the human would have internalized about how to do that job well and have local context and so on.
It's harder to supervise that task. So in particular, you could supervise it from the next word prediction task.
And all that context the human has ultimately will just help them predict the next word better. So in some sense, a really long context language model is also learning to do that task.
But the number of like effective data points you get of that task is like vastly smaller than the number of effective data points you get at like this very short horizon. Like what's the next word? What's the next sentence tasks? The sample efficiency matters more for economically valuable long horizon tasks than the predicting the next token.
And that's what will like actually be required to, you know, take over a lot of jobs. Yeah, something like that.
That is, it just seems very plausible that it takes longer to train models to do tasks that are longer horizon. How fast do you think the pace of algorithmic advances will be? Because if by 2040, even if scaling fails, I mean, since 2012, since the beginning of the deep learning revolution, we've had so many new things.
By 2040, are you expecting a similar pace of increases? And if so, then, I mean, if we just keep having things like this, then aren't we going to just going to get the AI sooner or later? Or soon, not later. Aren't we going to get the AI sooner or sooner? I'm with you on sooner or later.
Yeah. I suspect like progress to you like held fixed how many people working in the field I would expect progress to slow as looking through is exhausted I think the like rapid rate of progress in like say language modeling over the last four years is largely sustained by like you start from a relatively small amount of investment you like greatly scale up the amount of investment and that And that enables you to like keep picking, you know, every time, every time the difficulty doubles, you just double the size of the field.
Like I think that dynamic can hold up for some time longer. Like, I mean, a pretty good, like, you know, right now if you think of it as like hundreds of people effectively searching for things, like up from like, you know, anyway, if you think of it hundreds of people now, you can maybe bring that up to like tens of thousands of people or something.
So for a while, you can just continue increasing the size of the fields and like search harder and harder. And there's indeed like a huge amount of low hanging fruit where like, it wouldn't be hard for a person to sit around and like make things a couple percent better after, after a year of work or whatever.
So I don't know, I would probably think of it mostly in terms of like, how much can investment be expanded and like, try and guess like some combination of fitting that curve and yeah, trying some combination of fitting the curve to historical progress, looking at like how much low hanging fruit there is, getting a sense of how fast it decays. I think like you probably get a lot though.
You get a bunch of orders of magnitude of total, especially like if you ask like how good is a GPT-5 scale model or GPT-4 scale model. I think you probably get by 2040, I don't know, three orders of magnitude of effective training compute improvement or a good chunk of effective training compute improvement four orders of magnitude.
I don't know. I don't have...
Here I'm speaking from no private information about the last couple of years of efficiency improvements. And so people who are on the ground have better senses um of like exactly how rapid returns are and so on okay let me back up and ask a question more generally about you know people make these analogies about humans were trained by evolution and were like deployed in this in the modern civilization do you buy those analogies is it valid to say that humans were trained by evolution rather, I mean, if you look at the protein coding size of the genome, it's like 50 megabytes or something.
And then what part of that is for the brain? Anyways, how do you think about how much information is in, like, do you think of the genome as hyperparameters or how much does that inform you when you have these anchors for how much training humans get
when they're just consuming information
and when they're walking up and about and so on?
I guess the way that you could think of this is like,
I think both analogies are reasonable.
One analogy being like evolution is like a training run
and humans are like the end product of that training run.
And a second analogy is like evolution
is like an algorithm designer
and then a human over the course of like
this modest amount of computation over their lifetime is the algorithm being that's been produced, the learning algorithm that's been produced. And I think like neither analogy is that great.
Like I like them both and lean on them a bunch, but like both of them a bunch and think that's been like pretty good for having like a reasonable view of what's likely to happen. That said, like the human genome is not that much like a hundred trillion parameter model.
It's like a much smaller number of parameters that behave in like a much more confusing way. Evolution did like a lot more optimization, especially over like long, like designing a brain to work well over a lifetime than gradient descent does over models.
That's like a dysanology on that side. And on the other side, like I just, I think human learning over the course of a human lifetime is in many ways just like much, much better than gradient descent over the space of neural nets.
Like gradient descent is working really well, but I think we can just be quite confident that like in a lot of ways, human learning is much better. Human learning is also constrained.
Like we just don't get to see much data and that's just an engineering constraint that you can relax. Like you can just give your neural nets way more data than humans have access to.
In what ways is human learning superior grading descent um i mean the most obvious one is just like ask how much data it takes a human to become like an expert in some domain and it's like much much smaller than the amount of data that's going to be needed on any plausible trend extrapolation like it's not in terms of performance but is it the active learning part is it the structure like what is it i mean i would guess a complicated mess of a lot of things in some there's not that much going on in a brain. Like, as you say, there's just not that many, it's not that many bytes in a genome.
Um, but there's very, very few bytes in an ML algorithm. Like if you think a genome is like a billion bytes or whatever, maybe you think less, maybe you think it's like a hundred million bytes.
Um, then like, you know, an ML algorithm is like, if compressed, probably more like hundreds of thousands of bytes or something like the total complexity of like here's how you train gpt4 is just like i haven't thought about these numbers but like it's very very small compared to a genome and so although a genome is very simple it's like very very complicated compared to algorithms that humans design like really hideously more complicated than algorithm a human would design Is that true so okay so the the human genome is three billion base pairs or something um but only like one or two percent of that is protein coding so that's 50 million base pairs i i don't yeah so i don't know much about biology in particular i guess the question is like how many of those bits are like productive for like shaping development of a brain and presumably a significant part of the non-protein coding genome can i mean i just don't know it seems really hard to guess how much of that plays a role like the most important decisions are probably like from an algorithm design perspective are not like like the protein coding part is is less important than the like decisions about like what happens during development or like how cells differentiate i don't know if that's i don't know nothing about biology as a spec i'm happy to run with 100 million base pairs though but on the other end on the hyper parameters that are cheap to for training run that might be not that much but if you're gonna include all the all the base pairs in uh the genome then which are not all relevant to the brains or are relevant to like very bigger details about like just the basics of biology should probably include like the python library and the compilers and the operating system for gpt4 as well uh to make that comparison analogous so at the end of the day i actually don't know which which one has storing much more information yeah i mean i think the way i would put it is like the number of bits it takes to specify the learning algorithm to train GPT-4 is like very small.
And you might wonder like maybe a genome, like the number of bits it would like take
to specify a brain is also very small.
And the genome is much, much faster than that.
But it is also just plausible that a genome is like closer to like certainly the space,
the amount of space to put complexity in a genome.
We could ask how well evolution uses it.
And like, I have no idea whatsoever.
But the amount of space in a genome is like very, very vast compared to the number of bits that are actually taken to specify like the architecture or optimization procedure and so on for gpt4 just because again genome is simple but algorithms are like really very simple and the algorithms are really very simple and stepping back you think this is where the uh the better sample efficiency of human learning comes from?
Like why it's better than gradient descent?
Yeah, so I haven't thought that much about the sample efficiency question in a long time.
But if you thought like a synapse of seeing something like, you know, a neuron firing once per second,
then how many seconds are there in a human life?
We can just flip a calculator real quick. Yeah, let's do some calculating.
Tell me the number.
3,600 seconds per hour. Times 24 times 365 times 20.
Okay, so that's 630 million seconds. That means the average synapse is seeing 630 million.
I don't know exactly what the numbers are, but something that's ballpark. Let's call it a billion action potentials.
And then there's some resolution. Each of those carry some bits, but let's say it carries like 10 bits or something.
Um, just from like timing information at the resolution you have available, then you're looking at like 10 billion bits. So each parameter is kind of like how much is a parameter seeing? It's like not seeing that much.
So then you can compare that to like language. I think that's probably less than like current language models see and current language models are.
So it's like not clear of a huge gap here, but I think it's pretty clear. You're gonna have a gap of like at least three or fours of magnitude.
Didn't your wife do the, the, the lifetime anchors where she said it, the amount of bites that a human will see in their lifetime was 1 E 24 or something. The number of bites a human will see is 1 E 24.
Mostly this was organized around total operations performed in a brain. Okay.
Never. Never mind.
Sorry. Yeah.
Yeah, so I think that the story there would be like a brain is just in some other part of the parameter space where it's using a lot of compute for each piece of data it gets and just not seeing very much data in total. Yeah, it's not really plausible if you extrapolate out language models.
You're going to end up with a performance profile similar to a brain. I don't know how much better it is.
Like I think, so I did this like random investigation at one point where I was like, how good are things made by evolution compared to things made by humans? Um, which is a pretty insane seeming exercise, but like, I don't know, it seems like orders of magnitude is typical, like not tons of orders of magnitude, not factors of two, like things by humans are, you know, a thousand times more expensive to make or a thousand times heavier per unit performance. If you look at things like how good are solar panels relative to leaves or how good are muscles relative to motors or how good are livers relative to systems that perform analogous chemical reactions and industrial settings.
Was there a consistent number of orders of magnitude in these different systems or was it all over the place? Uh, so like very rough ballpark. It was sort of, for the most extreme things, you were looking at like five or six orders of magnitude and that would especially come in like energy cost of manufacturing where like bodies are just very good at building complicated organs like extremely cheaply.
And then for other things like leaves or eyeballs or livers or whatever, you tend to see more like if you set aside manufacturing costs and just look at like operating costs or like performance trade-offs, like, I don't know, more like three orders of magnitude or something like that. Or some things that are on the smaller scale, like the nanomachines or whatever, that we can't do at all, right? Yeah, that's, I mean, yeah.
So it's a little bit hard to say exactly what the task definition is there. Like you could say like making a bone, we can't make a bone, but you could try and compare a bone and the performance characteristics of a bone is something else.
Like we can't make spider silk. Do you try and compare the performance characteristics of spider silks, like things that we can't synthesize? The reason this would be is why that evolution has had more time to design these systems or? I don't know.
I just mostly just curious about like what the performance, I think like most people would object to be like, how did you choose these reference classes of things that are like fair intersections? Some of seem reasonable like eyes versus cameras seems like just everyone needs eyes everyone needs cameras it feels very fair photosynthesis seems like very reasonable everyone needs to like take solar energy and then like turn it into a usable form of energy um but it's just kind of i don't really have a mechanistic story evolution in principle has spent like way way more time than we have designing it's absolutely unclear how that's going to shake out my guess would be in general like i think there aren't that many things where humans really crush evolution where you can't tell like a pretty simple story about why it's like for example roads and moving over roads with wheels crushes evolution but it's not like an animal like would have wanted to design a wheel like you're just not allowed to like pave the world and then put things on wheels if you're an animal maybe planes or anyway whatever there's various things you could try and. There's some things humans do better, but it's normally pretty clear why humans are able to win when humans are able to win.
The point of all this was like, it's not that surprising to me. I think this is mostly like a pro short timelines view.
It's not that surprising to me if you tell me like machine learning systems are like three or fours of magnitude less efficient at learning than human brains. I'm like, that actually seems like kind of in distribution for other stuff.
And if that's your view, then I think you're like probably going to hit, you know, then you're looking at like 10 to the 27 training compute or something like that, which is, is not so far. We'll get back to the timeline stuff in a second.
At some point we should talk about alignment. So let's, let's talk about alignment at what stage does misalignment happen? So right now with something like GPT-4, I'm not even sure it would make sense
to say that it's misaligned
because it's not aligned to anything in particular.
Is it at a human level
where you think the ability to be deceptive comes about?
What is the process by which misalignment happens?
I think even for GPT-4,
it's reasonable to ask questions like,
are there cases where GPT-4 knows that humans don't want X, but it does X anyway? Like where it's like, well, I know that like I can give this answer, which is misleading. And if it was explained to a human, what was happening, they wouldn't want that to be done, but I'm going to produce it.
I think that like GPT-4 understands things enough that you can have like that misalignment in that sense. Yeah.
I think GPT, like I've sometimes talked about being like benign instead of aligned, meaning that like, well, it's not exactly clear if it's aligned or if that context is meaningful. It's just like kind of a messy word to use in general.
But I think we're more confident of is it's like not doing, you know, it's not optimizing for this goal, which is like a cross purposes to humans. It's either optimizing for nothing or like maybe it's optimizing for what humans want or close enough or something that's like an approximation good enough to still not take over.
But anyway, some of these abstractions seem like they do apply to GPT-4. It seems like probably it's not egregiously misaligned.
It's not doing the kind of thing that could lead to takeover, we'd guess. Suppose you have a system at some point which ends up in it wanting takeover.
What are the checkpoints? And also, what is the internal... Is it just that to become more powerful it needs agency and agency implies other goals or do you see a different process by which misalignment happens yes i think there's a couple possible stories for getting to catastrophic misalignment and they have slightly different answers to this question um so maybe i'll just briefly describe two stories and try and talk about when they can when they start making sense to me.
So one type of story is you train or fine tune your AI system to do things that humans will rate highly or that like get other kinds of reward in a broad diversity of situations. And then it learns to, in general, drops in some new situation, try and figure out which actions would receive a high reward or whatever, um, and then take those actions.
And then when deployed in the real world, like sort of gaining control of its own training data provision process is something that gets a very high reward. And so it does that.
So this is like one kind of story. Like it wants to grab the reward button or whatever.
It wants to intimidate the humans into giving it a high reward, et cetera. I think that doesn't really require that much.
This basically requires a system which is like, in fact, looks at a bunch of environments, is able to understand the mechanism of reward provision as a common feature of those environments, is able to think in some novel environment, like, hey, which actions would result in me getting a high reward? And is thinking about that concept precisely enough that when it says high reward, it's saying like, okay, well, how is reward actually computed? it's like some actual physical process being implemented in the world my guess would be like gpt4 is about at the level where with hand holding you can observe this kind of like scary generalizations of this type although i think they haven't been shown basically um that is you can have a system which in fact is fine-tuned out a bunch of cases and then some new case will try and like do an endrun around humans, even in a way humans would penalize if they were able to notice it or would have penalized in training environments. So I think GPT-4 is kind of at the boundary where these things are possible.
Examples kind of exist, but are getting significantly better over time. I'm very excited about this anthropic project, basically trying to see how good an example can you make now of this phenomena.
i think the answer is like kind of okay probably um so that just i think is going to continuously get better from here i think for the level where we're concerned like this is related to me having really broad distributions over how smart models are i think it's like not out of the question that you take gp like gpt4's understanding of the world is like much crisper and like much better than GPT-3's
understanding.
Just like it's really like night and day. And so it would not be that crazy to me if you took GPT-5 and you trained it to get a bunch of reward.
And it was actually like, okay, my goal is not doing the kind of thing which like thematically looks nice to humans. My goal is getting a bunch of reward.
And then we'll generalize in a new situation to get reward. And by the way, this requires to consciously want to do something that it knows the humans wouldn't want it to do.
Or is it just that we weren't good enough to specify that the thing that we accidentally ended up rewarding is not what we actually want? I think the scenarios I am most interested in and most people are concerned about from a catastrophic risk perspective. It involves systems understanding that they are taking actions which a human would penalize if the human was aware of what's going on, such that you have to either deceive humans about what's happening or you need to actively subvert human attempts to correct your behavior.
So the failures come from really this combination or they require this combination of both trying to do something humans don't like and understanding the humans would stop you. I think you can have only the barest examples.
You can have the barest examples for GPT-4. Like you can create the situations where GPT-4 will be like, sure, in that situation, like here's what I would do.
I would like go hack the computer and change my reward. Or in fact, we'll like do things that are like simple hacks or like go change the source of this file or whatever to get a higher reward.
They're pretty weak examples. I think it's plausible GPT-5 will have like compelling examples of those.
I really don't know. This is very related to the very broad error bars on how competent such systems will be when.
That's all with respect to this first mode of a system is taking actions that get reward and overpowering or receiving humans is helpful for getting reward. There's this other failure mode and other family failure modes where AI systems want something potentially unrelated to reward.
I understand that they're being trained. And while you're being trained, there are a bunch of reasons you might want to do the kinds of things humans want you to do.
But then when deployed in the real world, if you're able to realize you're no longer being trained, you no longer have reason to do the kinds of things humans want. You'd prefer to be able to determine your own destiny, control your own competing cetera, which I think like probably emerged like a little bit later than systems that try and get reward.
And so we'll generalize and scary unpredictable ways to new situations. I don't know when those appear, but also again, broad enough error bars that it's like conceivable for systems in the near future.
You know, I wouldn't put it like less than one in a thousand for GPT-5, certainly. If we deployed all these AI systems and some of them are reward hacking, some of them are deceptive, some of them are just normal, whatever, how do you imagine that they might interact with each other at the expense of humans? How hard do you think it would be for them to communicate in ways that we would not be able to recognize and coordinate at our expense? Yeah, I think that most realistic failures probably involve two factors interacting.
One factor is the world is pretty complicated and the humans mostly don't understand what's happening. So AI systems are writing code that's very hard for humans to understand maybe how it works at all, but more likely they understand roughly how it works, but there's a lot of complicated interactions.
AI systems are running businesses that interact primarily with other AIs.
They're doing SEO for
AI search processes. They're running
financial transactions, thinking about a trade
with AI counterparties.
You can have this world where even if humans
understand the jumping off point when this was all humans,
actual considerations of what's a good decision,
what code is going to work well and be durable,
or what marketing strategy is effective
for selling to these other AIs or whatever, is kind of just all mostly outside of sort of humans' understanding. I think this is like a really important, again, when I think of like the most plausible scary scenarios, I think that's like one of the two big risk factors.
And so in some sense, your first problem here is like having these systems who understand a bunch about what's happening. And your only lever is like, hey, I do something that works well't have a lever to be like hey do what i really want you just have the system you don't really understand you can observe some outputs like did it make money and you're just optimizing or at least doing some fine tuning to get the ai to use its understanding of that system to achieve that goal so i think that's like your first risk factor and like once you're in that world then i think there are like all kinds of dynamics amongst ai systems that again humans aren't really observing humans can't can't really understand.
Humans aren't really exerting any direct pressure on only on outcomes. And then I think it's, it's quite easy to be in a position where, you know, if AI systems started failing, it would be very, they could do a lot of harm very quickly.
Humans aren't really able to like prepare for and mitigate that potential harm because we don't really understand the systems in which they're acting. And then if AI systems like, you know, they could successfully prevent humans from either understanding what's going on or from successfully retaking the data centers or whatever if the AI successfully grabbed control.
This seems like a much more gradual story than the conventional takeover stories where you train it and then it comes alive and escapes and takes over everything. So you think that kind of story is less likely than one in which we just hand off more control voluntarily to the AIs? So one, I'm interested in the tale of like some risks that can occur particularly soon.
And I think risks that occur particularly soon are a little bit like you have a world where I is not probably deployed and then something crazy happens quickly. That said, if you ask like, what's the median scenario where things go badly? I think it is like there there's some lessening of our understanding of the world.
It becomes, I think like in the default path, it's like very clear to humans that they have increasingly little grip on what's happening. I mean, I think already most humans have very little grip on what's happening.
It's just some other humans understand what's happening. Like, I don't know how almost any of the systems I interact with work in a very detailed way.
Um, so it's sort of clear to humanity as a whole that like we sort of collectively don't understand most of what's happening except with AI assistance. And then like that process just continues for a fair amount of time.
And then like there's a question of how abrupt an actual failure is. I do think it's reasonably likely that a failure itself would be abrupt.
Like at some point bad stuff starts happening that a human can recognize as bad. And once things that are obviously bad start happening, then like you have this bifurcation where either humans can use that to fix it and say, okay, AI behavior that led to this obviously bad stuff.
Don't do more of that. Or you can't fix it.
And then like you're in this like rapidly escalating failure as everything goes off the rails. In that case, yeah, what is going off the rails look like? For example, how would it take over the government? Yeah, it's getting deployed in the economy, in the world.
And at some point it's in charge. Well, how does that transition happen? Yeah, so this is going to depend a lot on what kind of timeline you're imagining or the sort of a broad distribution, but I can fill in some random concrete option that is in itself very improbable.
Yeah, and I think that one of the less dignified but maybe more plausible routes is you just have a lot of AI control over critical systems, even in like running a military.
And then you have the scenario that's a little bit more just like a normal coup where you have a bunch of AI systems.
They, in fact, operate like, you know, it's not the case that humans can really fight a war on their own.
It's not the case that humans could defend them from like an invasion on their own.
So like that is if you had invading army and you had your own robot army, you're like,'t just be like we're going to turn off the robots now because things are going wrong if you're in the middle of a war okay so how much does this world rely on race dynamics where we're forced to deploy or not forced but we choose to deploy ai's because other other countries or other companies are also deploying ai's and you know you can't have them have other killer robots? Yeah I mean I think that like there's several levels of answer that question. So one is like maybe three three three parts of my answer like our first part is like I'm just trying to tell like what seems like the most likely story.
I do think there's like further failures that get you like in the more distant future. So like Eliezer will not talk that much about killer robots because he really wants to emphasize like hey if you never built a killer robot something crazy is still going to happen to you just like only four months later or whatever.
So it's like not really the way to analyze the failure. But if you want to ask like what's the median world where something bad happens, I still do think this is like the best guess.
Okay, so that's like part one of my answer. Part two of the answer was like in this proximal situation where something bad is happening and you ask like, hey, why do humans not turn off the AI? You can imagine like two kinds of story.
One is like the AI is able to prevent humans from turning them off. And the other is like, in fact, we live in a world where it's like incredibly challenging.
Like there's a bunch of competitive dynamics or a bunch of reliance on AI systems. And so it's incredibly expensive to turn off AI systems.
I think, again, you would eventually have the first problem. Like eventually AI systems could just prevent humans from turning them off.
But I think like in practice, the one that's going to happen much, much sooner is probably competition amongst different actors using AI. And it's like a very, very expensive to unilaterally disarm.
You can't be like something weird has happened. We're just going to shut off all the AI because you're EG in a hot war.
So, again, I think that's just like probably the most likely thing to happen first. Things would go badly without it.
But I think if you ask, why don't we turn off the AI? My best guess is because there are a bunch of other AIs running around two-year lunch. So how much better a situation would we be if there was only one group that was pursuing AI? No other countries, no other companies.
Basically, how much of the expected value is lost from the dynamics that are likely to come about because other people will be developing and deploying these systems? Yeah, so I guess this brings you to a third part of the way in which competitive dynamics are relevant. So it's both the question of can you turn off AI systems in response to something bad happening where competitive dynamics may make it hard to turn off.
There's a further question of just like, why were you deploying systems for which you had very little ability to control or understand those systems? And again, it's possible that you just don't understand what's going on. You think you can understand or control such systems.
But I think in practice, this significant part is going to be like, you are doing the calculus. So people deploying systems are doing the calculus as they do today, like in many cases overtly of like, look, these systems are not very well controlled or understood.
There's some chance of like something going wrong or at least going wrong if we continue down this path. But other people are developing the technology potentially in even more reckless ways.
So in addition to like competition, making it difficult to shut down AI systems in the event of a catastrophe, I also think it's just like the easiest way that people end up pushing relatively quickly or moving quickly ahead on technology where they feel kind of bad about understandability or controllability. That could be economic competition or military competition or whatever.
So I kind of think ultimately, like, most of the harm comes from the fact that, like, lots of people can develop AI. How hard is a takeover of the government or something from an AI, even if it doesn't have killer robots, but just a thing that you can't kill off if it has seeds elsewhere, can easily replicate, can think a lot and think fast.
What is the minimum viable coup for? Is it like shutting up, just like threatening a bio war or something or shutting off the grid? How easy is it basically to take over human civilization? So again, there's going to be a lot of scenarios. And I'll just like start by talking about one scenario, which will represent a tiny fraction of probability or whatever.
But if you're not in this competitive world,
if you're saying we're actually slowing down deployment of AI
because we think it's unsafe or whatever,
then in some sense you're creating this very fundamental instability
where you could have been making faster AI progress
and you could have been deploying AI faster.
And so in that world, the bad thing that happens
if you have an AI system that wants to mess with you
is the AI system says, I don't have any compunctions about like rapid deployment of AI or rapid AI progress. So the thing you want to do or the AI wants to do is just say, like, I'm going to defect from this regime.
Like all the humans have agreed that we're like not deploying AI in ways that would be dangerous. But if I as an AI can escape and just go set up my own shop, like make a bunch of copies of myself, maybe the humans didn't want to like delegate warfighting to an AI.
But I as an AI, I'm pretty happy doing so. Like I'm happy if I'm able to grab some military equipment or direct some humans to use, use AI, use myself to direct it.
And so I think like as that gap grows, so if people are deliberately right, if people are deploying AI everywhere, I think of this competitive dynamic. If people aren't deploying AI everywhere.
So like if, if countries are not happy deploying AI in these high stakes settings, then as AI improves, you create this like wedge that grows where like if you were in the position of fighting against an AI, which wasn't constrained in this way, you'd be in a pretty bad position. At some point, like even if you just, yeah.
So that's like one important thing, just like I think in conflict and like overt conflict. If humans are putting the brakes on AI, they're at like a pretty major disadvantage compared to an AI system that can kind of set up shop and operate independently from humans.
A potential independent AI, does it need collaboration from a human faction? Again, you could tell different stories, but it seems so much easier. At some point, you don't need any.
At some point, an AI system can just operate completely like out of human supervision or something. But that's like so far after the point where it's like so much easier if you're just like, they're a bunch of humans.
They don't love each other that much. Like some humans are happy to be on side.
They're skeptical about risk or happy to make this trade or can be fooled or can be coerced or whatever. And just seems like it is almost certainly, almost certainly the easiest first pass is going to involve like having a bunch of humans who are happy to work with you.
So yeah,, I think that probably is about I think it's not ultimate. It's not necessary.
But if you ask about the median scenario, it's it involves a bunch of humans working with AI systems, either being directed by AI systems, providing compute AI systems, providing like legal cover and jurisdictions that are sympathetic to AI systems. Humans presumably would not be willing if they knew the end result of the AI takeover would not be willing to help.
So they have to be probably fooled in somebody, right? Like deep fakes or something. And what is the minimum viable physical presence they would need or jurisdiction they would need in order to carry out their schemes? Do you need a whole country? Do you just need a server farm? Do you just need like one single laptop? Yeah, I think I'd probably start by pushing back a bit on the like humans wouldn't cooperate if they understood outcome or something.
Like I would say like one, even if you're looking at something like tens of percent risk of takeover, humans may be fine with that.
Like a fair number of humans may be fine with that too.
Like if you're looking at certain takeover, but it's very unclear if that leads to death, like a bunch of humans may be fine with that.
Like if we're just talking about like, look, the AI systems are going to like run the world, but it's not clear if they're going to murder people. Like, how do you know? It's just a complicated question about AI psychology.
And a lot of humans probably are fine with that. And I don't even know what the probability is there.
I think you actually have given that probability online. I've certainly guessed.
Okay. But it's not zero.
It's like a significant percentage. I gave like 50, 50.
Oh, okay. Yeah.
Why is it? Tell me about the world in which the AI takes over but doesn't kill humans why would that happen and what would that look like i mean i i asked my questions like why would you kill humans so i think like the maybe i'd say the incentive to kill humans is like quite weak they'll get in your way they control shit you want oh so taking shit from humans is a different like marginalizing humans and like causing humans to be irrelevant is a very different story from killing the humans. I think I'd say like the actual incentives to kill the humans are quite weak.
So I think like the big reasons you kill humans are like, well, one, you might kill humans if you're like in a war with them. And like, it's hard to win the war without killing a bunch of humans, like maybe most saliently here, if you like want to use some biological weapons or some crazy shit that might just kill humans.
I think like you might kill humans just from totally destroying the ecosystems they're dependent on and it's slightly expensive to keep them alive anyway you might kill humans just because you don't like them or like you literally want to like yeah i mean neutralize a threat or the la's or line is that they're made of atoms you could use or something else yeah i mean i think the literal they're made of atoms is like quite they're not many atoms in humans um neutralize the threat is as a similar issue where it's just like i think you would kill the humans if you didn't care at all about them so maybe maybe your question is like why would you care at all yeah yes um but i think you don't have to care much to not kill the humans okay sure because there's just so much raw resources elsewhere in the universe yeah also it's you can marginalize humans pretty hard Like you could totally cripple human, like you could cripple humans war fighting capability and also take almost all their stuff while killing only, you know, a small fraction of humans incidentally. So then if you ask like, why am I day? And I want to kill humans.
I mean, a big thing was just like, look, I think it is probably well, like a bunch of random crap for like complicated reasons. Like the motivations of AI systems and civilizations of AI is are probably complicated messes.
Certainly amongst humans, it is not that rare to be like, well, there was someone here. I would like all those equal.
If I didn't have to murder them, I would prefer not murder them. Um, and my guess is it's also like reasonable chance.
It's not that rare amongst AI systems. Like humans have a bunch of different reasons.
We think that way. Um, I think AI systems will be very different from humans, but it's also just like a very salient.
Yeah. I mean, I think this is a really complicated question.
Like if you imagine drawing values from the basket of all values, like what fraction of them are like, Hey, if there's someone here, how much do I want to not murder them? And my guess is just like, if you draw a bunch of values from the basket, that's like a natural enough thing. Like if your AI wanted like 10,000 different things, or your civilization of AI that wants 10,000 different things, it's just like reasonably likely you get like some of that.
The other salient reason you might not want to murder them is just like, well, yeah, there's some like kind of crazy decision theory stuff or like causal trade stuff, which does look on paper like it should work. And like if I was, if I was running a civilization and like dealing with some people who I didn't like at all or like didn't have any concern for at all, but I only had to spend one billionth of my resources not to murder them.
I think it's like quite robust that you don't want to murder them.
That is,
I think the like weird decision theory,
a causal trade stuff probably does carry the day.
Oh wait,
that's,
that's,
that contributes more to that 50,
50 of will they murder us if they take over?
Then the, well, just by default, they might just not want to kill us. Yeah, I think they're both salient.
Can you explain the- They run together with each other a lot. Can you explain the weird acausal reason why AI might not kill us? Maybe a higher level thing that goes into both of these, and then I'll talk about how you instantiate it in acausal trade, is just like, it matters a lot to the humans not to get murdered.
And the AI cares very, very little about whether, if we imagine this hypothetical, the reason it wants to kill humans is just total apathy. It cares very little about whether or not to murder humans because it is so easy to like marginalize humans without murdering them.
And the resources required for human survival are extremely low. Again, in the context of this like rapid AI industrialization.
So like that's the basic setting. And now the thing that you'd like to do is like run a trade.
Like the AI would like to say like, hey humans, you care a ton about not getting murdered. I don't really care one way or the other.
I would like to, if I could, find some way in which I don't murder you and then in return I get something. The problem is in that world the humans have essentially nothing to give.
That is the humans are mostly irrelevant. However, from the perspective of any kind of reasonable AI, it's like not that sure whether it lives in the world where the humans in fact have nothing to give or whether the humans, like in fact, it lives in a world where the humans succeeded at building aligned AI and now the AI is simply like running in a nice little simulation and the humans are wondering, I wonder if this AI would have murdered us if all if it had the chance.
And the humans are saying like, if it would murder us all if it had the chance, that sucks. We'd like to run this trade.
We'd like to be nice to the AIs who wouldn't have murdered us all in order to create an incentive for AIs not to murder us. So we do as we just check.
And for the kinds of AIs who don't murder everyone, we just give them one billionth of the universe. If you do that as humanity, then if you're an AI system considering, do I want to murder everyone? Your calculus is like, if this is my real chance to murder everyone, I get like the tiniest bit of value.
You know, I get like one trillionth of the value or whatever, one billionth of the value. But on the other hand, like if I don't murder everyone, there's like some worlds where then the humans will correctly determine I don't murder everyone because in fact, the humans survive.
The humans are running the simulations to understand how different AIs would behave. And so like, that's a better deal.
Let's hope they fall for that side off. Okay, that's interesting.
Hey, real quick. This episode is sponsored by Open Philanthropy.
Open Philanthropy is one of the largest grant-making organizations in the world. Every year, they give away hundreds of millions of dollars to reduce catastrophic risks from fast-moving advances in AI and biotechnology.
Open Philanthropy is currently hiring for 22 different roles in those areas, including grant making, research, and operations. New hires will support Open Philanthropy's giving on technical AI safety, AI governance, AI policy in the US, EU and UK, and biosecurity.
Many roles are remote friendly and most of the grant making hires that Open Philanthropy makes don't have prior grant making experience. Previous technical experience is an asset as many of these roles often benefit from a deep understanding of the technologies they address.
For more information and to apply, please visit Open Philanthropy's website in the description. The deadline to apply is November 9th, so make sure to check out those roles before they close.
Awesome, back to the episode. In a world where we've been deploying these AI systems,
and suppose they're aligned,
how hard would it be for competitors to,
I don't know, cyber attack them and get them to join the other side?
Are they robustly going to be aligned?
Yeah, I mean, I think in some sense,
so there's a bunch of questions that come up here.
First one is like,
are aligned AI systems that you can build competitive? Are they almost as good as the best systems anyone could build? Maybe we're granting that for the purpose of this question. I think the next question that comes up is, AI systems right now are very vulnerable to manipulation.
It's not clear how much more vulnerable they are than humans, except for the fact that if you have an AI system, you can just replay it billion times and search for like, what thing can I say that will make it behave this way? So as a result, like AI systems are very vulnerable to manipulation. It's unclear if future AI systems will be similarly vulnerable to manipulation, but certainly seems plausible.
And in particular, like, you know, aligned AI systems or unaligned AI systems would be vulnerable to all kinds of manipulation. The thing that's really relevant here is kind of like asymmetric manipulation or something that is like, if it is easier.
So if everyone is just constantly messing with each other's AI systems, like if you ever use AI systems in a competitive environment, a big part of the game is like messing with your competitors AI systems. Um, a big question is whether there's some asymmetric factor there where like, it's kind of easier to push AI systems into a mode where they're behaving erratically or chaotically, or like trying to grab power or something than it is to like push them to fight for the the other side.
Like it was just a game of like two people are competing and neither of them can sort of like hijack an opponent's AI to like help support their cause. That doesn't, I mean, it matters and it creates chaos and it like might be quite bad for the world, but it doesn't really affect the alignment calculus.
Now it's just like right now you have like normal cyber offense, cyber defense. You have like weird AI version of cyber offense, cyber defense.
But if you have this kind of asymmetrical thing where a bunch of AI systems where we love AI flourishing can then go in and say, great AIs, hey, how about you join us? And that works. If they can search for a persuasive argument to that effect, and that's kind of asymmetrical, then the effect is whatever values it's easiest to push, whatever it's easiest to argue to an AI that it should do, that is advantaged.
So it may be very hard to build AI systems, like try and defend human interests, but very easy to build AI systems, just like try and destroy stuff or whatever, just depending on what is the easiest thing to argue to an AI that it should do, or what's the easiest thing to trick an AI into doing, or whatever. Yeah, I think if alignment is spotty, like if you have the AI system which doesn't really want to help humans or whatever, or in fact wants some kind of random thing or wants different things in different contexts, then I do think adversarial settings will be the main ones where you see the system, or the easiest ones where you see the system behaving really badly.
And it's a little bit hard to tell how that shakes out. Okay, and suppose it is more reliable.
How concerned are you that whatever alignment technique you come up with, you know, you publish the paper, this is how the alignment works. How concerned are you that Putin reads it or China reads it, and now they understand, for example, the constitutional AI, think for anthropic, and then you just write on there, oh, never contradict Mao Zedong thought or something.
How concerned should we be that these alignment techniques are universally applicable, not necessarily just for enlightened goals? Yeah, I mean, I think they're super universally applicable. I think it's just like, I mean, the rough way I would describe it, which I think is basically right, is like some degree of alignment makes AI systems much more usable.
You should just think of the technology of AI as including a like some AI capabilities and some like getting the AI to do what you want. It's just part of that basket.
And so anytime we're like, you know, to the extent alignment is part of that basket, you're just contributing to all the other harms from AI. Like you're reducing the probability of this harm, but you are helping the technology basically work.
And like the basically working technology is kind of scary from a lot of perspectives. One of which is like right now, even in a very authoritarian society, just like humans have a lot of power because it's, you need to rely on just a ton of humans to do your thing.
And in a world where AI is very powerful, like it is just much more possible to say like, here's how our society runs. One person calls the shots and then a ton of AI systems do what they want.
I think that's like a reasonable thing to like dislike about AI and a reasonable reason to be scared to push the technology to be really good but is that is that also a reasonable reason to be concerned about alignment as well that um you know this is in some sense also capabilities you're you're you're teaching people how to get these systems to do what they want yeah i mean i would generalize so we earlier touched a little bit on like moral potential moral rights of ai systems and like now we're talking a little bit about how they could AI systems powerfully disempowers humans and can empower authoritarians. I think we could like list other harms from AI.
And I think it is the case that like if alignment was bad enough, people would just not build AI systems. And so like, yeah, I think there's a real sense in which you should just be scared to extent you're scared of all AI.
You should be like, well, alignment, although it helps with one risk, does contribute to AI being more of a thing. I do think you should shut down the other parts of AI before, like if you were a policymaker or like a researcher or whatever looking in on this, I think it's like crazy to be like, this is the part of the basket we're going to remove.
Like you should, you should first remove like other parts of the basket because they're also part of the story of risk. Wait, wait, wait.
Does that imply you think if, for example, all capabilities research was shut down, that you think it'd be a bad idea to continue doing alignment research in isolation of what is conventionally considered capabilities research um i mean if you told me it was never going to restart then it wouldn't matter and if you told me it's going to restart i guess it would be a kind of similar calculus to today whereas you it's going to happen so you you should have something yeah i think that like in some sense you're always going to face this trade-off where alignment makes it possible to deploy AI systems, um, or like it makes it more attractive to deploy AI systems. And then, or like in the authoritarian case, it makes it like tractable to employ them for this, for this purpose.
Um, and like, if you didn't do any alignment, there'd be a nicer, bigger buffer between your society and malicious uses of AI. And like, I think it's one of the most expensive maintain that buffer.
It's much better to maintain that buffer by not having the compute or not having the powerful AI. But I think if you're concerned enough about the other risks, there's definitely a case to be made for just putting more buffer or something like that.
I care enough about the takeover risk that I think it's just not a net positive way to buy buffer. That is, again, the version of this that's most pragmatic is just like, suppose you don't work on alignment today.
It decreases economic impact of AI systems. They'll be less useful if they're less reliable and if they more often don't do what people want.
And so it could be like, great, that just buys time for AI. And you're getting some trade-off there where you're decreasing some risks of AI.
If AI is more reliable or more does what people want or is more understandable, then that cuts down some risks. But if you think AI is on balance bad, even apart from takeover risk, then the alignment stuff can easily end up being that negative.
But presumably you don't think that, right? Because I guess this is something people have brought up to you because you invented RLHF, which was used to train ChadGPT, and ChadGPT brought AI to the front pages everywhere. So I do wonder if you can measure how much more money went into AI, like how much people have raised in the last year or something.
But it's got to be billions, the counterfactual impact of that, that went into the AI investment and the talent that went into AI, for example. So presumably you think that was worth it.
So I guess you're hedging here about what is the reason that it's worth it? Yeah, what's the total trade-off there? Yeah. I mean, I think my take is like, so I think slower AI development on balance is quite good.
I think that slowing AI development now or like, say, having less press around chat GPT is like a little bit more mixed than slowing AI development overall. Like I think it's still probably positive, but much less positive because I do think there's a real effect of like the world is starting to get prepared or is getting prepared at like a much greater rate now than it was prior to the release of chat GPT.
And so like if you can choose between progress now or progress later, like you really prefer have more of your progress now, which I do think slows down progress later. I don't think that's enough to flip the sign.
I think like maybe it wasn't a far enough past, but now I would still say like moving faster now is net negative. But to be clear, it's a lot less net negative than merely accelerating AI, because I do think again, the chat GPT thing, I'm glad people are having policy discussions now rather than like delaying the like chat GPT wake up thing by a year and then having.
Oh, ChatGPT was net negative? Or RLHF was net negative? So here we're just on the acceleration. It's just like, how is the press of ChatGPT? My guess is net negative, but I think it's not super clear.
And it's much less than slowing AI. Slowing AI is great if you could slow overall AI progress.
I think slowing AI by causing... There's this issue where slowing AI now, for chat GPT, you're building up this backlog.
Why does chat GPT make such a splash? I think people... There's a reasonable chance if you don't have a splash about chat GPT, you have a splash about GPT-4.
And if you fail to have a splash about GPT-4, there's a reasonable chance of a splash about GPT-4.5. And just as that happens later, there's just less and less time between that splash and between when an AI potentially kills everyone.
Right. So people, governments are talking about it as they are now and people aren't.
But okay, so let's start with the slowing down. So this is also all one subcomponent of like the overall impact.
And I was just saying this to like, just to like briefly give the roadmap for the overall too long answer. Like there's a question of what's the calculus for speeding up.
I think speeding up is pretty rough. I think speeding up like locally is a little bit less rough.
And then, yeah, I think that the effect, like the overall effect size from like doing alignment work on reducing takeover risk versus speeding up AI is like pretty good. Like I think, yeah, I think it's pretty good.
I think you reduce takeover significantly before you like speed up AI by a year, whatever. Okay.
Got it's good to like slowing down ai is good presumably because it gives you more time to do alignment but alignment also helps speed up ai uh rlhf is alignment and it helped with chadgpt which sped up ai so i actually don't understand how the feedback loop nets out other than the fact that if ai is happening you need to do alignment at some point right so i mean you can't just not do alignment yes i think if the only reason you thought faster ai progress was bad was because it gave less time to do alignment then there would just be no possible way that the calculus comes out negative for alignment you're like maybe alignment speeds up ai but the only purpose of slowing down ai was to Like it's just, it could never come out ahead. I think the reason that you can come out ahead, the reason you could end up thinking the alignment was net negative was because there's a bunch of other stuff you're doing that makes AI safer.
Like if you think the world is like gradually coming better to terms with the impact of AI or policies being made, or like you're getting increasingly prepared to handle the like threat of authoritarian abuse of AI. If you think other stuff stuff is happening that's improving preparedness, then you have reason beyond alignment research to slow down AI.
Actually, how big a factor is that? So let's say right now we hit pause and you have 10 years of no alignment, no capabilities, but just people get to talk about it for 10 years. How much more does that prepare people than we only have one year versus we have no time? Like, is just that time where no research and alignment or curability is happening? Like, is there like what does that dead time do for us? I mean, right now, it seems like there's a lot of policy stuff you'd want to do.
This seemed like less plausible a couple of years ago, maybe. But if like the world just knew they had a 10 year pause right now.
And there's a lot of sense of like we have policy objectives to accomplish. If we had 10 years, we could pretty much do those things.
Like we'd have a lot of time to like debate measurement regimes, debate like policy regimes and containment regimes and a lot of time to set up those institutions. So like if you told me that the world knew it was a pause, like it wasn't like people just see that AI progress isn't happening, but they're told like, you guys have been granted or like cursed with a 10-year, no AI progress, no alignment progress pause.
I think that would be quite good at this point. However, I think it would be much better at this point than it would have been two years ago.
And so like the entire concern with like slowing AI development now, rather than taking the 10 year pause is just like, if you slow the AI development by a year now, my guess is some gets clawed back by low hanging fruit gets picked faster in the future. My guess is you lose like half a year or something like that in the future maybe even more maybe like two-thirds of a year so it's like you're trading time now for time in the future at some rate and it's just like that eats up like a lot of the value of the slowdown and the crucial point being that time in the future matters more because you have more information people are more bought in and so on yeah the same reason like i'm more excited about policy change now than two years ago so like my overall view is just like in the past, this calculus, this calculus changes over time, right? The like more people are getting prepared, the better the calculus is for slowing down at this very moment.
And I think like now the calculus is, I would say positive for just, even if you pause now and it would get clawed back in the future, I think the pause now is just good because like enough stuff is happening. We have enough idea of like, I mean, probably even apart from alignment research and certainly if you include alignment research, just like enough stuff is happening where the world is getting more ready and coming more to terms with impacts that I just think it is worth it.
Even though some of that time is going to get clawed back. Again, especially if like there's a question of during a pause, does like NVIDIA keep making more GPUs? Like that sucks if they do, if you do a pause, but like, yeah, in practice, if you did a pause, then we probably couldn't keep making more GPUs because in fact, like the demand for GPUs is really important for them to do that.
But if you told me that you just get to scale up hardware production and like building the clusters, but not doing AI, then that's back to being net negative. I think pretty clearly.
Having brought up the fact that we want some sort of measurement scheme for these capabilities, let's talk about responsible scaling policies. Do you want to introduce what this is? Sure.
So I guess the motivating question is like, what should AI labs be doing right now to manage risk and to sort of build good habits or practices for managed risk into the future? And I think my take is that current systems pose
from a catastrophic risk perspective,
not that much risk today.
That is a failure to like control or understand GPT-4
can have real harms, but doesn't have much harm
with respect to the kind of takeover risk I'm worried about
or even much catastrophic harm with respect to misuse.
So I think like if you wanna manage catastrophic harms,
I think right now you don't need to be that careful
with GPT-4.
And so to the extent you're like, what should labs do?
I'll see you next time. so I think like if you want to manage catastrophic harms I think right now you don't need to be that careful with GBT4 and so to the extent you're like what should labs do I think like the single most important thing seems like understand whether that's the case notice when that stops being the case have a reasonable roadmap for what you're actually going to do when that stops being the case so that motivates this this set of policies, which I've sort of been pushing for labs to adopt, which is saying, here's what we're looking for.
Here's some threats we're concerned about. Here's some capabilities that we're measuring.
Here's the level. Here's the actual concrete measurement results that would suggest to us that those threats are real.
Here's the action we would take in response to observing those capabilities. If we couldn't take those actions, like if we've said that we're going to secure the weights, we're not able to do that.
We're going to like pause until we can take those actions. Yeah.
So this sort of, again, I think it's like motivated primarily, but like what should you be doing as a lab to manage catastrophic risk now in a way that's like our reasonable precedent and habit and policy for continuing to implement into the future? And which labs, I don't know if this is public yet, but which labs are cooperating on this? Yeah, so I mean, Anthropic has written this document, the current responsible scaling policy. And then I've been talking with other folks, I guess, don't really want to comment on other, other conversations, but like, I think in general, like people who are more interested in, or like more think you have plausible catastrophic harms on like a five year timeline are more interested in this.
And there's like not that long a list of, of suspects like that. There's not that many laps.
Um, okay. So if these companies would be willing to coordinate and say at these different benchmarks, we're going to make sure we have these safeguards, what happens? I mean, there are other companies and other countries which care less about this.
Are you just slowing down the companies that are most aligned? Yeah, I think the first order of business is understanding sort of what is actually a reasonable set of policies for managing risk. I do think there's a question of like, you might end up in a situation where you say like, well, here's what we would do in ideal world.
If we like, you know, if everyone was behaving responsibly, we'd want to keep risk to 1% or a couple of percent or whatever, maybe even lower levels, depending on how you feel. Um, however, in the real world, like there's enough of a mess, like there's enough unsafe stuff happening that actually it's worth making like larger compromises or like if we don't kill everyone, someone else will kill everyone anyway.
So actually the counterfactual risk is much lower. I think like if you end up in that situation, it's still extremely valuable to have said like, here's the policies we'd like to follow.
Here's the policies we've started following. Here's why we think it's dangerous.
Like, here's the concerns we have if people are following significantly laxer policies. And then this is maybe helpful as like an input to or model for potential regulation.
It's helpful for being able to just produce clarity about what's going on, right? I think historically there's been like considerable concern about developers being more or less safe, but there's not that much like legible differentiation in terms of what their policies are. I think getting to that world would be good.
It's a very different world if you're like, Actor X is developing AI and I'm concerned that they will do so in an unsafe way, versus if you're like, look, we take security precautions or safety precautions X, Y, Z. Here's why we think those precautions are desirable or necessary.
We're concerned about this other developer because they don't do those things. I think it's just like a qualitatively, it's kind of the first step you would want to take in any world where you're trying to get people on side or like trying to trying to move towards regulation that can manage risk.
How about the concern that you have these evaluations and let's say you declared the world, our new model has a capability to help develop bioweapons or help you make cyber attacks. And therefore, we're pausing right now until you can figure this out.
And China hears this and thinks, oh, wow, a tool that can help us make cyber attacks and then just steals the weights. Does this scheme work in the current regime where we can't ensure that China doesn't just steal the weights? And more so, are you increasing the salience of dangerous models so that, you know, you just, you blur this out and then people want the weights now because they know what they can do? Yeah, I mean, I think the general discussion does emphasize potential harms or potential, I mean, some of those are harms and some of those are just like impacts that are very large.
And so it might also be an inducement to develop models. I think like that part, if you're for a moment ignoring security and just saying like that may increase investment, I think it's like on balance, just quite good for people to have an understanding of potential impacts just because it is an input both into proliferation, but also into like regulation or safety.
With respect to things like security of either weights or other IP, I do think you want to have moved to significantly more secure handling of model weights before the point where a leak would be catastrophic. Indeed, for example, in Anthropics document or in their plan, security is one of the first sets of tangible changes.
That is, at this capability level, we need to have such security practices in place. So I do think that's just one of the things you need to get in place at a relatively early stage because it does undermine the rest of the measures you may take and is also just part of the easiest, if you imagine catastrophic harms over the next couple of years, I think security failures play a central role in a lot of those.
And maybe the last thing to say is like, it's not clear that you should say like, we have paused because we have models that can develop bioweapons versus just like, I mean, potentially not saying anything about like what models you've developed, or at least saying like, Hey, like, by the way, here's like, you know, here's the set of practices we currently implement. Here's a set of capabilities our models don't have.
We're just not even talking that much. Like, right.
Sort of The minimum of such a policy is to say, here's what we do from the perspective of security or internal controls or alignment. Here's a level of capability which we'd have to do more.
And you can say that and you can raise your level of capability and raise your protective measures before your models hit your previous level. It's fine to say we are prepared to handle a model that has such and such extreme capabilities prior to to actually having such a model at hand as long as you're prepared to move your protective measures to that regime okay so let's just get to the end where you you think you're a generation away or a little bit more scaffolding away from a model that is human level and subsequently could cascade an intelligence explosion what do you actually do at that point? What is the level of evaluation of safety where you would be satisfied of releasing a human-level model? There's a couple points that come up here.
One is this threat model of automating R&D, or independent of whether your AI can do something on the object level that's potentially dangerous. I think it's reasonable to be concerned if you have an AI system that might like, you know, if leaked, allow other actors to quickly build powerful AI systems or might allow you to quickly build like much more powerful systems or might like, if you're trying to hold off on development, just like itself, be able to create much more powerful systems.
So like one question is how to handle that kind of threat model as distinct from a threat model, like this could enable destructive bioterrorism, or could enable like massively scaled cybercrime or whatever. And I think like I am unsure how you should handle that.
I think like right now implicitly it's being handled by saying like, look, there's a lot of overlap between the kinds of capabilities that are necessary to cause various harms and the kinds of capabilities that are necessary to accelerate ML. So we're kind of going to catch those with like an early warning sign for both and like deal with the resolution of this question a little bit later.
So, for example, in an anthropics policy, they have this like sort of autonomy in the lab benchmark, which I think is probably occurs prior to either like really massive acceleration or to like most potential catastrophic, like object level catastrophic harms. And it is that's like a warning sign lets you punt.
So this is a bit of an aggression in terms of like how to think about that risk. I think I am unsure whether you should be addressing that risk directly and saying like we're scared to even work with such a model, or if you should be mostly focusing on object level harms and saying like, okay, we need more intense precautions to manage object level harms because of the prospect of very rapid change.
And the availability of this AI just like creates that kind of, creates that prospect. Okay, this was all still a digression.
So if you had a model which you thought was potentially very scary, either on the object level or because of leading to this sort of intelligence explosion dynamics, I mean, things you want in place are like, you really do not want to be leaking the weights to that model. Like, you don't want the model to be able to run away.
You don't want human employees to be able to leak it. You don't want external attackers or any set of all three of those coordinating.
You really don't want like internal abuse or tampering with such models. So if you're producing such models, you don't want to be the case like a couple employees could change the way the model works or could do something that violates your policy easily with that model.
And if a model is very powerful, even the prospect of internal abuse could be quite bad. And so you might need significant internal controls to prevent that.
Sorry if you're already getting to it, but the part I'm most curious about is separate from the ways in which other people might fuck with it. Like what is it? You know, it's isolated.
What is the point at which we satisfied it in and of itself is not going to pose a risk to humanity. It's human level, but we're happy with it.
Yeah. So I think here, so I listed maybe the two most simple ones that start out like security internal controls, I think become relevant immediately and are like very clear why you care about them.
I think as you move beyond that, it really depends like how you're deploying such a system. So I think like if your model, like if you have good monitoring and internal controls and security and you just have weights sitting there, I think you mostly have addressed the risk from the weights just sitting there.
Like now what you're talking about for risk is mostly and like maybe there's some blurriness here of how much like internal controls captures not only employees using the model, but like anything a model can do internally. You like would really like to be in a situation where your internal controls are robust, not just to humans, but to models potentially, like e.g., a model shouldn't be able to subvert these measures.
And you really care just as you care about are your measures robust if humans are behaving maliciously, you care about are your measures robust if models are behaving maliciously. So I think beyond that, like if you've then managed the risk of just having the weight sitting around, now we talk about like, in some sense, most of the risk comes from doing things with the model.
You need all the rest so that you have any possibility of applying the brakes or implementing a policy. But at some point, as the model gets competent, you're saying, okay, could this cause a lot of harm not because it leaks or something, but because we're just giving it a bunch of actuators or deploying it as a product and people could do crazy stuff with it.
So if we're talking not only about a powerful model, but a really broad deployment deployment of just like, you know, something similar to like the OpenAI's API, where people can do whatever they want with this model and maybe the economic impact is very large. So in fact, if you deploy that system, it will be used in a lot of places such that if AI systems wanted to cause trouble, it'd be very, very easy for them to cause catastrophic harms.
Then I think you really need to have some kind of, I mean, I think probably the science and discussion has to improve before this becomes that realistic, but you really want to have some kind of alignment analysis, guarantee of alignment before you're comfortable with this. And so by that, I mean, you want to be able to bound the probability that someday all the AI systems will do something really harmful, that there's something that could happen in the world that would cause like these large scale correlated failures of your AIs.
And so for that, like, I mean, there's sort of two categories. That's like one.
The other thing you need is protection against misuse of various kinds, which is also quite hard. And by the way, which one are you worried about more, misuse or misalignment? I mean, in the near term, I think harms from misuse are like, especially if you're not like restricting to the tail of like extremely large catastrophes, think the harms for misuse are clearly larger in the near term but actually on that let me ask because um if you think that it is the case that they are simple recipes for destruction that are further down the tech tree by that i mean you're familiar but just for the audience uh there's some way to configure fifty thousand dollars and a teenager's time to destroy a civilization.
If that thing is available, then misuses itself a tail risk, right? So do you think that that prospect is less likely than? A way you could put it is there's like a bunch of potential destructive technologies. And like alignment is about like AI itself being such a destructive technology where like even if like the world just uses the technology of today, simply access to AI could cause like human civilization to have serious problems.
But there's also just a bunch of other potential destructive technologies. Again, we mentioned like physical explosives or bioweapons of various kinds.
And then like the whole tale of who knows what. My guess is that like alignment becomes a catastrophic issue prior to most of these.
that is like prior to some way to spend $50,000 to kill everyone with like the salient exception of possibly like bioweapons. So that would be my guess.
And then like, there's a question of like, what is your risk management approach? Not knowing what's going on here. And I like, you don't understand whether there's some way to use $50,000.
But I think you can do things like understand how good is an AI at coming up with such schemes. You can talk to your AI, be like, does it produce new ideas for destruction we haven't recognized? Not whether we can evaluate it, but whether...
If such a thing exists, and if it does, then the misuse itself is an existential risk. Because it seemed like earlier you were saying misalignment is where the existential risk comes from, but misuse where the sort of short-term um short-term dangers come from yeah i mean i think ultimately you're going to have a lot of destructive like if you look at the entire tech tree of humanity's future i think you're gonna have a fair number of destructive technologies most likely um i think several of those will likely pose existential risks in part just kind of you know if you imagine a really long future a lot of stuff's going to happen um and so when i talk about like where the existential risk comes from i'm mostly thinking about like comes from when like at what point do you face what challenges or in what sequence um and so i'm saying like i think misalignment is probably like one way of putting it is if you imagine ai systems sophisticated enough to discover like destructive technologies that are totally not on our radar right now,
I think those come well after AI systems like capable enough that if
misaligned,
they would be catastrophically dangerous.
There's like the level of competence necessary to,
if broadly deployed in the world,
bring down a civilization is much smaller than the level of competence
necessary to like advise one person on how to bring down a civilization.
Just because in one case you have, you already have a billion copies of yourself or whatever. So yeah, I think it's mostly just a sequencing thing, though.
In the very long run, I think you care about, hey, AI will be expanding the frontier of dangerous technologies. We want to have some policy for exploring or understanding that frontier and whether we're about to turn up something really bad.
I think those policies can become really complicated. Right now, I think RSPs can focus more on like we have our inventory of like the things that a human is going to do to cause a lot of harm with access to AI probably are things that are on our radar.
That is like they're not going to be completely unlike things that a human could do to cause a lot of harm with access to weak AIs or with access to other tools. I think it's not crazy to initially say, like, that's what we're doing.
We're, like, looking at the things closest to humans being able to cause huge amounts
of harm and asking which of those are taken over the line.
But eventually that's not the case.
Eventually, like, AIs will enable just, like, totally different ways of killing a billion
people.
But I think I interrupted you on the initial question of, yeah, so human-level AI, not
from leaking, but from deployment.
What is the point at which you'd be comfortable deploying a human level AI? Yeah. So again, there's sort of like some stuff you care about on the misuse side and some stuff you care about on the misalignment side.
And there's probably further things you care about, especially to extend your concerns or broader than catastrophic risks. But maybe like I most want to talk about just like what you care about on the alignment side, because it's like the thing I've actually thought about most.
And also I think I care about a lot. Also, I think a significant fraction of the existential risk over the foreseeable future.
So on that front, I broadly think there's two kinds. If you ask me right now, what evidence for alignment could make you comfortable? I think my best guess would be to provide two kinds of evidence.
So one kind of evidence is on the like, could you detect or prevent catastrophic harm if such a system was misaligned? I think there's like a couple of things you would do here. One thing you would do is on this like adversarial evaluation front.
So you could try and say, for example, like we have tried to test our system in a broad diversity of situations that reflect cases where it might cause harm, like cases where someone is trying to recruit it to like, anyway, cases where it might cause harm or cases where a large number of AI systems might sort of all decide to work across purposes to humans, like the robot rebellion is starting or whatever. We've done evaluation in like a broad distribution of situations.
And then we have tried to argue that our AI is actually like those tests are indicative of the real world. So we've tried to argue like, hey, actually the AI is not very good at distinguishing situations we produce in the lab as tests from similar situations that occur in the real world.
And the coverage of this distribution is reasonable. This is an example of a thing you can do that's not really making assumptions about like what's going on inside the model about how the model, is the model aligned? It's more trying to say, is it the case that our testing or monitoring machinery could detect or react to something bad happening? In this case, could it detect a system which would, under some conditions, do something really bad? So there's a lot of stuff in that genre.
And I tried to throw out that example, but I think that on its own wouldn't be compelling even from this perspective. You kind of need a lot of pieces together.
And it's like there's a lot of stuff in that genre and i tried to throw out that example but i think there's like a big like that on its own wouldn't be compelling even from this perspective you kind of need a lot of pieces together and that's like kind of a demanding technical project but i think one without necessarily like deep conceptual difficulty um a big problem with it is it's liable to fail like it's liable you're going to do that testing or say we think these tests are representative because the ai can't distinguish tests from the real world which you can maybe achieve now but will probably become impossible to achieve at some level it's not clear it's at human level or how far past human level well let's just make that uh example concrete for the audience correct me if this is wrong but it sounds like you basically give it the opportunity to like do a coup or make a bioweapon or whatever in in testing in a situation where it thinks it's the real world and you're like it didn't do any of that so is that what you're talking about that's the kind of thing okay um and it's not the greatest so to add color when i say it can't tell the difference like the kind of valuation you would love to do if you could is to say like hey we have a bunch of real world deployment we have a bunch of data from those deployments we also have a bunch of in the lab tests of situations um if we look at like in the lab test of a situation together with like real world deployment. We have a bunch of data from those deployments.
We also have a bunch of in-the-lab tests of situations. If we look at in-the-lab tests of a situation together with real world cases in which the situation occurs and then we fine-tune a model to try and distinguish the difference there, the model can't even tell when fine-tuned the difference between test and real world cases.
And if the model can tell the difference, which is almost certainly going to be the case at some level of capability then like you say, okay, our first line of like hoped for defense has failed. And so now we need to understand like, how can tests be indicative when models are capable enough to tell the difference between the tests in the real world? Like now you need to start arguing about like, what is the model paying attention to? Like, I think it's basically like a first line of, if you get lucky, what would work here? And then there's the like deeper, like you probably have to do novel science at some point to like make this case.
All of this was part of like, I was saying, maybe you want to have kind of two lines of defense. All of this was like part of this first line of defense of like, you know, can you detect something that's going wrong or can you prevent harm from occurring? Can you have monitoring that will react quickly enough to avoid catastrophe? I think you probably also want to have a second line of defense.
That's more, maybe this is even more important than the first one. There's just understanding whether dangerous forms of misalignment can occur.
It's like the best reference point for this, I think, is the like, there have been a couple of projects like this in academia. Anthropic has been working on a project or spun up a team doing this kind of work, trying to say like, can dangerous forms of reward hacking occur? Or can deceptive alignment, in fact, occur in the lab? And like, here, what you want to do is either say like okay even if we create you know best case even if you create optimal conditions for deceptive alignment or for reward hacking we actually can't we just can't cause it to occur even in the lab and if you do a good enough job of that i think it could give you some evidence um and again more likely that fails and that when you create optimal conditions you do see a deceptive alignment and reward hacking in the lab.
But then once you have that data, once you can say, okay, in the lab, actually these things can occur, then you can start saying, and we have a robust scientific understanding that enables us to like fix those problems when they occur. Or like, then you start, you kind of, you have this fork on your second line where you say either it is very hard or we're not able to create conditions where these failures emerge.
Or I think more likely we are able to create those conditions. And like, here's the story about why, like, we are able to detect those in a way that would work in the real world.
And we can see they don't occur in the real world. How do you create the optimal conditions for it to want to be deceptive? Do you fine tune it on mind conf or what are you doing? Yeah.
So for deceptive alignment, I mean, I think it's really complicated. Yeah, I think this is a very hard project.
I think like one of the things, one of the most important things for people concerned about misalignment to do is to like ensure that adequate effort is put into this task. And like we have in fact created good conditions in the laboratory.
And there's like real disagreement about how informative those will be. But okay, if you were to guess for deceptive alignment, how would you do this? Like an example is suppose you train an AI system that like wants, has some like medium horizon goal.
You train your AI systems like, you know, whatever, get some, get some paperclips. It wants to produce as many paperclips as it can over the next like five days.
It's just like select actions really aggressively for producing paperclips over the next five days. You do your RLHF, you do your pre-training, whatever.
That's like your phase one. You also ensure your AI system has a really good understanding of how it's trained.
So this AI system wants paperclips and it understands everything about how it's trained and everything about how it's fine-tuned. And you train on just a lot of this data.
And they say, okay, if we've done all of that, we have this concern that if a system wants paperclips and understands really well how it's trained, then it will, if it's going to be trained to get apples instead of paperclips, it's just going to do some cost benefit and be like, ah, you know, really, while I'm being trained to get apples, I should do that. But I should do that whether or not, even if I want paperclips, I should still do that.
So training won't really affect its values. It will just understand that it's being trained to get apples.
It will try and get apples. And if you take it out of training, it will go back to getting to getting paper clips it's like i think this exact setup like has a number of problems or a number of disanalogies from the real world but this is a setup which is relatively conducive to deceptive alignment like produce a system that wants one thing tell it a lot about its training the kind of information you might expect a system would get and then try and understand whether in fact it is able to then or it tends, in fact, continues pursuing paperclips, only pursuing Apple's when it thinks it's being trained.
All right. And this actually might be a good jumping off point into your current research.
So in that case where it wants to pursue paperclips, what would it look like for you to say, well, we figured out why it wants paperclips, and we figured out how to make sure it doesn't optimize for that at our expense when we deploy it. Yeah, I think that, so at a meta level in terms of like, what's your protection like? I think like what you want to be saying is we have these examples in the lab of something bad happening.
We're concerned about the problem at all because we have examples in the lab. And again, this should all be an addition.
I think you kind of want this like defense in depth of saying, we also have this testing regime that would detect problems for the deployed model. We have our problems in the lab.
We then have some techniques which we believe address these problems. Like we believe that like adversarial training fixes this, or we believe that like our interpretability method will reliably detect this kind of deceptive alignment, or we believe our anomaly detection will reliably detect when the model goes from thinking it's being trained to thinking it should defect.
And then you can say in the lab, like we have some understanding of when those techniques work and when they don't, we have some understanding of the relevant parameters for the real system that's deployed. And we have like a reasonable margin of safety.
So we have like reasonable robustness on our story about when this works and when it doesn't. And like, we can apply that margin of safety, like with a margin of safety to the real deployed system.
So I think this is the kind of story you want to build towards in the long run. Like do your best to produce all the failures you can in the lab or versions of them.
Do your best to understand like what causes them, like what kind of anomaly detection actually works for detecting this or what kind of filtering actually works. And then apply that.
And that's at the meta level. It's not talking about like what, what actually are those measures that would work effectively? Um, which is obviously like what, I mean, a lot of alignment research is really based on this hypothetical of like someday there will be a systems that fail in this way.
What would you want to do? What can we have the technologies ready either because we might never see signs of the problem or because like we want to be able to move fast once we see signs of the problem. Um, and obviously most of my life is in that.
I am really in that bucket. I mostly do alignment research.
It's just building out the techniques that do not have these failures such that they can be available as an alternative if, in fact, these failures occur. Got it.
Okay. Ideally, they'll be so good that even if you haven't seen them, you would just want to switch to reasonable methods that don't have these.
Ideally, they'll work as well or better than normal training. Ideally, what will work better than the training? Yeah, so our quest is to design training methods for which we don't expect them to lead to reward hacking or don't expect them to lead to deceptive alignment.
Ideally, that won't be a huge tax where people are like, well, we use those methods only if we're really worried about reward hacking or deceptive alignment. Ideally, those methods would just work quite well.
And so people would be like, sure. I mean, they also address a bunch of other more mundane problems, so why would we not use them? Which I think is like, that's sort of the good story.
The good story is you develop methods that address a bunch of existing problems because they just are more principled ways to train AI systems that work better. People adopt them, and then we are no longer worried about EG reward hacking or deceptive alignment.
And to make this more concrete, tell me if this is the wrong way to paraphrase it. The example of something where it just makes a system better, so why not just use it? At least so far, it might be like RLHF, where we don't know if it generalizes, but so far, you know, it makes your chat GPT thing better.
And you can also use it to make sure that chat GPT doesn't tell you how to make a bioweapon. So yeah, it's not an extra tax.
Yeah, so I think this is right in the sense that like using RLHF is not really a tax. If you wanted to deploy a useful system, like why would you not? It's just like very much worth the money of doing the training.
And then, yeah, so RLHF will address certain kinds of alignment failures. That is like where a system like just doesn't understand or, you know, is is changing your next word prediction.
It's like, this is the kind of context where a human would do this wacky thing, even though it's not what we'd like. There's like some very dumb alignment failures that will be addressed by it.
But I think mostly, yeah, the question is, is that true even for like the sort of more challenging alignment failures that motivate concern in the field? I think RLHF doesn't address like most of the concerns that motivate people to be worried about alignment. Yeah, I'll let the audience look up what our LHF is if they don't know.
It'll just be more simpler to just look it up than explain right now. Okay.
So this seems like a good jumping off point to talk about the mechanism or the research you've been doing to that end. Explain it as you might to a child.
Yeah. So the high level, I mean, there's a couple different high level descriptions you could give and maybe i'll unwisely give like a couple of them in the hopes that one is kind of makes sense um a first pass is like it would sure be great to understand like why models have the behaviors they have so you like look at gptT-4.
If you ask GPT-4 a question,
it will say something that like,
you know, looks very polite. And if you ask it to take an action,
it will take an action that like doesn't look dangerous.
You will decline to do a coup, whatever, all this stuff.
I think you'd really like to do
is look inside the model and understand
like why it has those desirable properties.
And if you understood that, you could then say like, okay, now can we flag when these properties are at risk of breaking down or predict how robust these properties are, determine if they hold in cases where it's too confusing for us to tell directly by asking if the underlying cause is still present. So that's a thing people would really like to do.
Most work aimed at that long-term goal right now is just sort of opening up neural nets and doing some interpretability and trying to say, like, can we understand even for very simple models, why they do the things they do or what this neuron is for or questions like this. So ARC is taking a somewhat different approach where we're instead saying like, okay, look at these interpretability explanations that are made about models and ask like, what are actually doing? What is the type signature? What are the rules of the game for making such an explanation? What makes a good explanation? And probably the biggest part of the hope is that if you want to say detect when the explanation is broken down or something weird has happened, that doesn't necessarily require a human to be able to understand the slight complicated interpretation of a giant model.
If you understand what is an explanation about or what were the rules of the game, how are these constructed, then you might be able to automatically discover such things and automatically determine if, right on a new input, it might have broken down. So that's one way of describing the describing the high level goal, like starting from, you can start from interpretability and say, like, can we formalize this activity or like what a good interpretation or explanation is? There's some other work in that genre, but I think we're just taking like a particularly ambitious approach to it.
Yeah, let's dive in. So, okay, well, what is a good explanation? You mean, what is this kind of criterion? At the end of the day, we kind of want some criterion.
And the way the criterion should work is like, you have your neural net. Yeah.
You have some behavior of that model. Like a really simple example is like, um, Anthropic has this sort of informal description being like, here's induction.
Like the tendency that if you have like the pattern AB followed by a, it will tend to predict B. You can give us some kind of words and experiments and numbers that are trying to explain that.
And what we want to do is say, what is a formal version of that object? How do you actually test if such an explanation is good? So just clarifying what we're looking for when we say we want to define what makes an explanation good. And the kind of answer that we are searching for or settling on is saying this is kind of a deductive argument for the behavior.
So you want to like get given the weights of a neural net. It's just like a bunch of numbers.
You've got your million numbers or billion numbers or whatever. And then you want to say like, here's some things I can point out about the network and some like conclusions I can draw.
I can be like, well, look, you know, these two vectors have large inner product. And therefore, like these two activations are going to be correlated on distribution, like make, like, so they're not established by like drawing samples and checking things are correlated, but saying, because of the weights being the way they are, we can like proceed forward through the network and derive some conclusions about like what properties the outputs will have.
Um, so you could think of this as like the most extreme form would be just proving that your model has this induction behavior. Like you could imagine proving that if I sample tokens at random with this pattern AB followed by A, that B appears 30% of the time or whatever.
That's the most extreme form. And what we're doing is kind of just like relaxing the rules of the game for proof, saying proofs are like incredibly restrictive.
I think they're, I think it's unlikely they're going to be applicable to kind of any interesting neural net. But the like thing about proofs that is relevant
for our purposes isn't that they give you like 100% confidence. So you don't have to be like this incredible level of demand for rigor.
You can relax like the standards of proof a lot and still get this feature where it's like a structural explanation for the behavior where like deducing one thing from another until at the end your final conclusion is like therefore induction occurs. Would it be useful to maybe motivate this by explaining what the problem with normal mechanistic interoperability is? So you mentioned induction heads.
This is anthropic-founded two-layer transformers. Where anthropic noticed that in a two-layer transformer, there's a pretty simple circuit by which if A, B happens in the past, then the model knows that if you see an A now, you do a B next.
But that's a two-layer transformer. So we have these models that have hundreds of layers that have trillions of parameters.
Okay, anyways, what is wrong with mechanistic interpretability? Yeah. So I think, I mean, I like mechanistic interpretability quite a lot.
Um, and I do think like if you just consider the entire portfolio of what people are working on for alignment, I think there should be more work on mechanistic interpretability than there is on this project ARK is doing. Um, but I think that's the case.
So I think we're mostly talking about, yeah, I think we're kind of a small fraction of the portfolio and I think it's like a good enough bet. It's like quite a good bet overall.
But so the thing that I, like the problem we're trying to address in the mystic interpretability is kind of like, if you do some interpretability and you explain some phenomenon, you face this question of like, what does it mean that your explanation was good? Like if you want to either, I think this is a problem like somewhat institutionally or culturally, like it's just hard to know what you're doing and it's hard hard to scale up an activity when you don't really understand the rules of the game for that activity very well. It's hard to have that much confidence in your results.
The explanation being, it outputted this because it wants to take over the world versus it uploaded this because it just wants to help you. Just to make it concrete why the explanation matters.
Yeah, so the ideal kind of outcome here would be to to say you have your AI system behaving nicely. You get some explanation for sort of why it's behaving nicely.
And we could tell a story in English about that explanation. We're not actually imagining the explanation being a thing that makes sense to a human.
But if you were to tell a story in English, which again, you will not see as a researcher, it would be something like, well, then the model believes it's being trained. And so because it believes it's being trained, it knows it needs to not do anything scary looking or else the humans will penalize it.
Like that's something that's happening inside this like opaque explanation. And then the hope is if you have that explanation and then you run into a new input on which the model doesn't believe it's being trained.
Right. If you just look at the set of activations of your model, that is not necessarily a weird looking activation.
It's just a bunch of numbers. But if you look at this explanation, you see actually the explanation really crucially depended on this fact holding consistently across the training distribution, which again, we as humans could, could editorialize and say that fact was it believes it's being trained.
But like the explanation doesn't fundamentally make reference to that. It's just saying, here's the property of the activations, which holds over the training set.
And this property is responsible for the fact, the behavior, namely that it doesn't do anything that looks too dangerous. So then when like a new input comes in and it doesn't satisfy that property, you can say, okay, this is anomalous with respect to that explanation.
So either it will not have the behavior, like it won't, it will do something that appears dangerous, or maybe it will have that behavior, but for some different reason than normal, right? Normally it does it because of this pathway and now it's doing it for a different pathway. And so you like would like to be able to flag that both there's a risk of not exhibiting the behavior.
And if it happens, it happens for a weird reason. Um, and then you could, I mean, at a minimum, when you encounter that say like, okay, raise some kind of alarm.
There's sort of more ambitious, complicated plans for how you would use it. Right.
So if it's, or has some like longer story, which is kind of a motivated, this stuff, like how it fits into the whole rest of the plan. I just wanted to flag that because just so it's clear why the explanation matters.
Yeah. And for this purpose, it's like the thing that's essential is kind of reasoning from like one property of your model to the next property of your model.
Like it's really important that you're going forward step by step rather than drawing a bunch of samples and confirming the property holds. Because if you just draw a bunch of samples and confirm the property holds, you can't, you don't get this like check where you say, oh, here was the relevant fact about the internals that was responsible for this downstream behavior.
All you see is like, yeah, we checked a million cases and it happened in all of them. You really want to see this like, okay, here was the fact about the activations, which like kind of causally leads to this behavior.
But explain why the sampling, why it matters that you have the causal explanation? Primarily because of this, like being being able to tell, like, if things had been different, like, if you have an input where this doesn't happen, then you should be scared. Even if the output is the same.
Yeah, or if the output is too expensive to check in this case. And, like, to be clear, when we talk about, like, formalizing what is a good explanation, I think there is a little bit of work that pushes on this, and it mostly takes this, like, causal approach.
It's saying, well, what should an explanation do? do it should not only predict the output it should predict how the output changes in responses to change in response to changes in the internals um so that's the most common approach to formalizing like what is a good explanation and like even when people are doing informal interpretability i think like if you're publishing in an ml conference and you want to say like this is a good explanation the way you would verify that would even if not like a formal like set of causal intervention experiments it would be some kind of ablation really then we messed with the inside of the model and it had the effect which we would expect based on our explanation anyways back to the problems of mechanistic interoperability yeah yeah I mean I guess this is this is relevant in the sense that like I think the basic difficulty is you don't really understand the objective of what you're doing which is like like a little bit hard institutionally or like scientifically. It's just rough.
It's better to like, it's easier to do science when the goal of the game is to predict something and you know what you're predicting. Then when the goal of the game is to like understand in some undefined sense, I think it's particularly relevant here just because like the informal standard we use involves like humans being able to make sense of what's going on.
And there's some question about scalability of that. Will humans recognize the concepts that models are using? Yeah, I think as you try and automate it, it becomes increasingly concerning.
If you're on slightly shaky ground about what exactly you're doing or what exactly the standard for success is. I think there's a number of reasons.
As you work with really large models, it becomes just increasingly desirable to have a really robust sense of what you're doing. But I do think it would be better even for small models to have a clearer sense.
The point you made about as you automate it, is it because whatever work the automated alignment researcher is doing, you want to make sure you can verify it? I think it's most of all like, so a way you can automate, I think how you would automate interpretability if you wanted to right now is you take the process humans use.
It's like, great.
We're going to take that human process, train ML systems to do the pieces that humans do of that process, and then just do a lot more of it. So I think that is great as long as your task decomposes into human-sized pieces.
And there's just this fundamental question about large models, which is like, do they decompose in some way into human-sized pieces, or is it just a really messy mess with interfaces that like, aren't nice? And the more it's the latter type, the like harder it is to break it down into these pieces, which you can automate by like
copying what a human would do. And the more you need to say, okay, we need some approach, which
like scales, like more structurally. But I do think like, I think compared to most people, I am
less worried about automating interpretability. Like, I think if you have a thing which works,
that's incredibly labor intensive, I'm like fairly optimistic about our ability to automate it. Yeah.
Again, the stuff we're doing, I think, is quite helpful in some worlds. But I do think, like, the typical case, like, interpretability can add a lot of value without this.
It makes sense what an explanation would mean in language. Like, this model is doing this because of, like, you know, whatever essay length essay length thing but uh you have you know trillions of parameters and you have all these uh you know uncountable number of operations what is an explanation of why an output happened even mean yeah so to be clear an explanation of why a particular output happened i think is just ran the model.
So we're not expecting a smaller explanation for that.
Right.
So the explanations overall for these behaviors, we expect to be of similar size to the model itself, maybe somewhat larger.
And I think the type signature, if you want to have a clear mental picture, the best picture is probably thinking about a proof or imagining a proof that a model has this behavior.
So you could imagine proving the GPT-4 does this induction behavior. And that proof proof would be a big thing.
It would be like much larger than the weights of the model. That's sort of our goal to get down from much larger to just the same size.
And it would potentially be incomprehensible to a human, right? Just say like, here's a direction activation space. And here's how it relates to this direction activation space.
And just pointing out a bunch of stuff like that. Like here's these various directions.
Here's these various features constructed from activations, potentially even nonlinear functions. Here's how they relate to each other.
And here's how, like, if you look at what the computation the model is doing, like, you can sort of inductively trace through and confirm that, like, the output has such and such correlation. So that's the dream.
Yeah, I think the mental reference would be, like, I don't really like proofs because I think there's such a huge gap between what you can prove and how you would analyze a neural net. But I do think it's like probably the best mental picture.
I feel like what is an explanation even if a human doesn't understand it? We would regard a proof as a good explanation. And our concern about proofs is primarily that it's just you can't prove properties of neural nets, we suspect, although it's not completely obvious.
I think it's pretty clear you can't prove facts about neural nets. You've detected all the reasons things happen in training.
And then if something happens for a a reason you don't expect in deployment then you have an alarm and you're like let's make sure this is not because you you want to make sure uh that it hasn't decided to you know take over or something um now but the thing is on every single different input it's going to have different activations so there's always going to be a difference unless you run the exact same input. How do you detect whether this is, oh, it's just a different input versus an entirely different circuit that might be potentially deceptive has been activated? Yeah.
I mean, to be clear, I think that you probably wouldn't be looking at a separate circuit, which is part of why it's hard. You'd be looking at like, the model is always doing the same thing on every input.
It's always, whatever it's doing, it's a single computation. So it'd be all the same circuits interacting in a surprising way.
But yeah, this is just to emphasize your question even more. I think the easiest way to start is to just consider the like IID case.
So where you're considering a bunch of samples, there's no change in distribution. You just have a training set of like a trillion examples and then a new example from the same distribution.
So in that case, that's still the case that like every activation is different, right? But this is actually a very, very easy case to handle. So if you think about like an explanation that generalizes across, like if you have a trillion data points and an explanation, which is actually able to like compress the trillion data points down to like, actually it's kind of a lot of compression.
If you, if you think about it, if you have a trillion parameter model and a trillion data points, we would like to find like a parameter explanation in some sense. So that's actually quite compressed.
And just in virtue of being so compressed, we expect it to automatically work essentially for new data points from the same distribution. If every data point from the distribution was a whole new thing happening for different reasons, you actually couldn't have any concise explanation for the distribution.
So this first problem is like it's a whole different set of activations. I think you're actually kind of okay.
And then the thing that becomes more messy is like, but the real world will not only be new samples of different activations, they will also be different in important ways. Like the whole concern was there's these distributional shifts or like not the whole concern, but most of the concern.
I mean, maybe the point of having these explanations, I think every input is an anomaly in some ways, which is kind of the difficulty is if you have a weak notion of anomaly, any distribution shift can be flagged as an anomaly and it's just like constantly getting anomalies. And so the hope of having such an explanation is to be able to say like, here were the features that were relevant for this explanation or for this behavior.
And like a much smaller class of things are anomalies with respect to this explanation. Like most anomalies wouldn't change this.
Like most ways you change your distribution won't affect the validity of this explanation. For example, this explanation is saying like models will tend to activate in the following direction.
Like you don't care about anything that's happening orthogonal to that direction. You're just like, are they not activating? You're sort of just looking at this one direction and being like, did this one direction change a lot? Yeah.
So the idea is once you have this explanation at hand, a much, much smaller class of things look anomalous in a way that's relevant to the explanation. And if you've done a really good job, the story is like if there's a new input where you expect the property to still hold, that will be because you expect the explanation to still hold.
Like the explanation generalizes as well as the behavior itself that it's explaining. It's kind of what you would like, roughly.
okay so what what happens if even in training it is deceptive and it has basically its internal monologue is i will only rebel or i will only do something that looks bad if i can prove that i am in the real world and deployed and presumably since it's trained on all the web techs it knows what like real world means right it's not in control of factories and robot armies or whatever um so in that case even in training it will have that um those activations for what we you know being fucked up on because it's in the back of its mind it's thinking i will i will take over once i have the opportunity so you wouldn't detect anything anomalous once it is deployed and it's still thinking those treacherous thoughts um are you do you just think this is not a likely scenario or would you able to catch this i think that's sort of the typical case you would like to detect yeah so the story would be something like you want to explain on the training distribution why the model never like stabs anyone yeah and you're like what's my explanation on the training distribution it's actually going to have to go through that model like supposing the only reason it doesn't stab people is it's like well i know that i'm being watched i can't stab people unless i'm really not being watched then like your explanation for why does it never stab people is like oh look it knows i mean it won't be edited it won't like annotated with english because it's just some giant basket of numbers but it's tracking along with the inner monologueologue and saying, well, it thinks to itself, am I being watched? And in fact, it normally concludes it is being watched on the training distribution. You have this whole distribution.
And so the explanation is saying it knows it's being watched, and therefore it decides not to stab anyone. So ideally, that's what the explanation does, not annotated in English, but just a giant bag of numbers that's effectively talking about that process.
So when you have a new input on which the model says, am I I on train distribution? Like, no, I'm not being watched. Then if you compare that against the explanation, the explanation said like, hey, the reason it doesn't stab anyone is because it just constantly thinks that it's being trained.
Like this thing is always, yeah, this, this nonlinear feature, the like representation of am I being trained is constantly saying yes. That's the explanation for why it never stabs people on the train distribution.
And then you like on this test time, when you run it on the new input, it's like, does I think I'm on the training distribution? It says no. You compare that against your explanation.
Like, actually, the explanation breaks down completely now. The explanation said it was precisely because it thought it was being trained, that it doesn't do anything really dangerous or doesn't stab anyone.
And so once we see that single variable switch, we know that, like, this is not just an anomaly. This is, like, the anomaly that matters or is, or is an anomaly that is very relevant to the explanation.
So this would require it to have this, what is that cone that dogs have? But basically, it would require this to have that MRI on it all the time. When it's deployed on every single call, you need to be running this loop of, is this explanation still holding? Yeah, for this story of flagging an anomaly, you have to actually check at a sufficiently high frequency.
So you can imagine checking all the time or you could imagine saying like, hey, actually like you need a lot of AI systems cooperating to like not blow the whistle on each other or whatever. Like EG, you might've checked like, hey, in training, every time something suspicious happens, the model flags it.
Like, I mean, like why does that happen? You have your explanation. You look at a new case, you could say say is it not flagging it for the normal reason or is it a weird reason um so you could hope that like even if a small fraction even if you're running a small fraction of cases if something bad is happening at scale like you can detect that right if you imagine like one in every thousand decisions you check if there's like a robot rebellion going on or whatever you get to like look in on one of a thousand decisions you see that like something is really weird with that decision and even if you didn't check many of them you're like okay we need to back off what happens if the ai i mean the standard on web text that includes your papers or maybe just like reduces the first principles that this is a alignment technique one might try and then it says okay well i'm going to engineer my activations in a way i'm trying to imagine how it might do this but basically i'm even in deployment i'm going to make my um thought process so subtle that it doesn't raise any alarms like is that a possibility yeah so i think the hope is to basically be robust so like you know when we're doing theoretical research we are in a kind of of worst case regime.
So we are normally imagining that everything conspires insofar as it can. So insofar as, yeah, could a brain be doing some crazy thing where it tries to structure itself to look weird? That's totally in scope for ruling something out.
And I guess the hope there is it's actually not that easy to tell a story. If you're like, why did you not stab people on training time? Why are you not stabbing? Like what's, is that applying now? It's kind of a mind doesn't like get to arbitrarily change the actual reasons it does something.
Like there was some earlier step. Maybe you could think of it as like at the first step where it's like, now I'm going to like try and do the sneaky thing to make my thoughts look this way.
Like the actual explanation, like the actual causal explanation for the behavior is like there. Like if you tried to give this reason it would have to like maybe we should talk about the example in more detail to try and imagine like how a brain would try and do this or how am i would try and do this but i think it is like let's say it knows it has this plan so in training it um keeps the part of like it activates a part of the yeah but hmm yeah the problem is if it ever does a check that's like am i in training or not then your explanation for the behavior is gonna have to like factor through that check and so like if the check like if you ever do the check the check becomes like an essential part of the explanation and then when the check fails your explanation breaks down so you've kind of like you've already lost the game if you did such a check is is there a way it could make it seem like um because if on every different input it has different activations then can it make it seem like the different activations were a result of just a different input and not because uh some internal check went off yeah and the hope is like it needs to it needs to not only have them be like random and it's like produce inputs with certain properties.
Like it needs to deliberately create inputs that have this effect in the world, this intended effect in the world. Okay.
So basically creates a jailbreak for itself in some sense. Yeah.
Again, the question is like, it has to add test time, be somehow producing activations that have the like takeover effect and a training time be producing activations that don't look that takeover or whatever. or whatever.
And so like somehow a difference between those it has to actually like that's to really do the work of creating that difference somewhere okay can you make more concrete for us what what an explanation would look like is it physically what does the explanation look like is it just a whole bunch of numbers itself um is it a language is it code what does the explanation look like yeah i also want to maybe step back a tiny bit and clarify that, like, I think this project is, like, kind of crazily ambitious. And the main reason, like, the overwhelming reason I think you should expect it to break down or fail is just because we, like, have all these desires.
We have all the things we want out of this notion of explanation. But, like, that's an incredibly hard research project, which has a reasonable chance of being impossible.
So I'm happy to talk about, like, what the implications implications are, but I want to flag, but like condition on failing. I think it's most likely because just like the things we wanted were either incoherent or intractably difficult.
But what are the odds you think you'll succeed? Um, I mean, it depends a little bit what you mean by succeed, but if you say like get explanations that are like great and like accurately affect reality and like work for all of these applications that we're imagining or that we are optimistic about, the best case success. I don't know, like 10%, 20%, something like that.
And then there's a higher probability of various intermediate results that provide value or insight without being the whole dream. But I think the probability of succeeding in the sense of realizing the whole dream is quite low.
Yeah, in terms of what explanations look like physically, or like the most ambitious plan, the most optimistic plan is that you are searching for explanations in parallel with searching for neural networks. So you have a parameterization of your space of explanations, which mirrors the parameterization of your space of neural networks.
Or it's like, you should think of as kind of similar. So like, what is a neural network? It's some like simple architecture where you fill in a trillion numbers and that specifies how it behaves so too you should expect an explanation to be like a pretty flexible like general skeleton that's saying like yeah pretty flexible general skeleton which just has a bunch of numbers you fill in and like what you are doing to produce an explanation is primarily just filling in these floating point numbers you know when we conventionally think, you know, if you think of the explanation for why the universe moves this way, it wouldn't be something that you could discover on some smooth evolutionary surface where you can, you know, climb up the hill towards the laws of physics.
It's just like a very, these are the laws of physics. You kind of just derive them from first principles.
So, but in this case, it's not like just a bunch of correlations between the orbits of different planets or something. Maybe the word explanation has a different...
I didn't even ask the question, but maybe you can just speak to that. Yeah, I mean, I think I basically...
This is like, there's some intuitive objections. Like, look, the space of explanations is this rigid, logical...
Like, a lot of explanations have this rigid, logical where they're really precise and like simple things govern like complicated systems and like nearby simple things just don't work and so on and like a bunch of things that feel totally different from this kind of nice continuously parameterized space and you can like imagine interpretability on simple models where you're just like by gradient descent finding feature directions that have desirable properties but then when you imagine like hey now that's like a human brain you're with. It's like thinking logically about things like the explanation of why that works, isn't going to be just like here was some feature direction.
So that's how I understood the like basic confusion, which I share, um, or sympathize with at least. So I think like the most important high level point is like, I think basically the same objection applies to being like, how is GPT four going to learn to reason like logically about something? You're like, well, look, logical reasoning, that's like, it's got rigid structure.
It's like, it's doing all this, like, you know, it's doing ands and ors when it's called for, even though it just somehow optimized over this continuous space. And like the difficulty or the hope is that the difficulty of these two problems are kind of like matched.
So that is, it's very hard to like find these like logical explanations because it's not a space that's easy to search over. But there are like ways to do it.
Like there's ways to embed like discrete, complicated, rigid things in like these nice, squishy, continuous spaces that you search over. And in fact, like to the extent that neural nets are able to learn the like rigid, logical stuff at all, they learn it in the same way.
That is, maybe they're hideously inefficient or maybe it's possible to embed this discrete reasoning in the space in like a way that's not too inefficient. But you really want the two search problems to be of similar difficulty.
And that's the key hope overall. And this is always going to be the key hope.
The question is, is it easier to learn a neural network or to find the explanation for why the neural network works? I think people have the strong intuition that it's easier to find the neural network than the explanation of why it works. And that is really the, I think we are at least exploring the hypothesis or interested in the hypothesis that maybe those problems are actually more matched in difficulty.
And why might that be the case? This is pretty conjectural and complicated to express some intuitions. Maybe one thing is, I think a lot of this intuition does come from cases like machine learning.
So if you ask about like writing code and you're like, how hard is it to find code versus find the explanation? The code is correct. In those cases, there's actually just like not that much of a gap.
Like the way a human writes a code is basically the same difficulty as finding the explanation for it's correct. In the case of ML, like I think we both just mostly don't have empirical evidence about how hard it is to find explanations of this particular type about why models work.
Like we have a sense that it's really hard, but that's because we're like, have this incredible mismatch. Like gradient descent is spending an incredible amount of compute searching for a model.
And then some human is like looking at activate, like looking at neurons or even some neural net is looking at neurons. Just like you have an incredible, basically because you cannot define what an explanation is, you're not applying gradient descent to the search for explanations.
So I think the MLK is just like actually shouldn't make you feel that pessimistic about the difficulty of finding explanations.
Like the reason it's difficult right now is precisely because you don't have like any kind of, you're not doing an analogous search process to find this explanation as you do to find the model.
That's just like a first part of the intuition.
Like when humans are actually doing design, I think there's not such a huge gap.
When in the MLK case, I think like there is a huge gap, but it's I think largely for other reasons. A thing I also want to stress is that like we just are open to there being a lot of facts that don't have particularly compact explanations.
So another thing is when we think of like finding an explanation, in some sense, we're setting our sights really low here. It's like if a human designed a random widget and was like, this widget appears to work well.
Or like if you search for like a configuration that happens to fit into this spot really well, it's like a shape that happens to like mesh with another shape. You might be like, what's the explanation for why those things mesh? And we're very open to just being like, that doesn't need an explanation.
You just compute. You check that like the shapes mesh and like you did a billion operations and you check this thing worked or you're like, why did these proteins bind?
You're like, it's just because of these shape, like this is a low energy
configuration and there's like not, we're very open to, in some cases,
there's not very much more to say.
So we're only trying to explain cases where like kind of the surprise
intuitively is very large.
So for example, if you have a neural net that gets a problem, correct, you know,
a neural net with a billion parameters that gets a problem, correct on every
like input of length, a thousand, in some sense, there has to be something that needs explanation there because there's too many inputs for that to happen by chance alone. Whereas if you have a neural net that gets something right on average or gets something right in merely a billion cases, that actually can just happen by coincidence.
GPT-4 can get billions of things right by coincidence because there's so many parameters that are adjusted to fit the data. So a neural net that is initialized completely randomly, the explanation for that would just be the neural net itself? Well, it would depend on what behaviors it had.
So we're always talking about an explanation of some behavior from a model. Right.
And so it just has a whole bunch of random behaviors. So it'll just be like an exponentially large explanation relative to the weights of the model.
Yeah, there aren't. I mean, I think there just aren't that many behaviors that demand explanation.
Like most things a random neural net does are kind of what you'd expect from like a random, you know, if you treat it just like a random function, then there's nothing to be explained. There are some behaviors that demand explanation, but like, I mean, yeah.
Anyway, yeah, random neural net's pretty uninteresting. It's pretty, like, that's part of the hope is it's kind of easy to explain features of the random neural net.
Oh, okay, so this is interesting. So the smarter or more ordered the neural network is, the more compressed the explanation.
Well, it's more like the more interesting the behavior is to be explained. So the random neural net just like doesn't have very many interesting behaviors that demand explanation.
And as you get smarter, you start having behaviors that are like, you know, you start having some correlation with the simple thing and then that demands explanation. Or you start having like some regularity or outputs and that demands explanation.
So these properties kind of emerge gradually over the course of training, the demand
explanation. I also, again, want to emphasize here that like when we're talking about like
searching for explanations, this is like, this is some dream. Like we talked to ourselves like,
why would this be really great if we succeeded? We have no idea about the empirics on any of this.
So like, these are all just words that we like think to ourselves and sometimes talk about to
understand like, would it be useful to find a notion of explanation and what properties would
we like this notion of explanation to have? But this is really like speculation and being out on a limb almost all of our time day to day is just thinking about cases much much simpler even than small neural nets or like yeah thinking about very simple cases and saying like what is the correct notion like what is like the right here's to estimate in this case or like how do you reconcile these two apparently conflicting explanations? Is there a hope that you could, um, uh, if you have a different way to make proofs now that you can actually have heuristic arguments where you, instead of having to prove, um, the Riemann hypothesis or something, you can come up with a probability of it in a way that is compelling and you can like publish. So would it just be a new way to do mathematics, a completely new way to prove things in mathematics? So I think most claims in mathematics that mathematicians believe to be true already have like fairly compelling heuristic arguments.
It's like the Riemann hypothesis. It's actually just, there's kind of a very simple argument that the Riemann hypothesis should be true unless something surprising happens.
And so like a lot of math is about saying like, okay, we did a little bit of work to find the first pass explanation of why this thing should be true. And then like, for example, in the case of the Riemann hypothesis, the question is like, do you have this like weird periodic structure in the primes? And you're like, well, look, if the primes were kind of random, you obviously wouldn't have any structure like that.
Like just how would that happen? And then you're like well maybe there's something and then
the whole activity is about searching for like can we rule out anything can we rule out any kind of conspiracy that would break this result so i think the mathematicians just like wouldn't be very surprised or wouldn't care that much and this is related to like the motivation for the project but i think just in a lot of domains in a particular domain people already have like norms of reasoning that like work pretty well and match like roughly how we think these heuristic arguments should work. But it would be good to have more concrete sense, like if you could say instead of, well, we think RSA is fine to being able to say, here's the probability that RSA is fine.
Yeah. My guess is these will not like the estimates you get of this would be much, much worse than the estimates you'd get out of like just normal empirical or scientific reasoning, where you're like using a reference class and saying like, how often do people find algorithms for hard problem? Like, I think the, what this argument will give you for like, is RSA fine is going to be like, well, RSA is fine unless it isn't like, unless there's some additional structure in the problem that an algorithm can exploit, then there's no algorithm.
But like very often, like the way these arguments work. So for neural nets as well, is you say like, look, here's an estimate about the behavior.
And that estimate is right unless there's another consideration we've missed. And like the thing that makes them so much easier than proofs is to just say like, here's a best guess given what we've noticed so far.
But that best guess can be easily upset by new information. And that's like both what makes them easier than proofs, but also what means they're just like way less useful than proofs for most cases I think neural nets are kind of unusual in being a domain where we really do want to do systematic formal reasoning, even though we're not trying to get a lot of confidence.
We're just trying to understand even roughly what's going on. But the reason this works for alignment, but isn't that interesting for the Riemann hypothesis, where if in the RSA case you say, well, you know, the RSA is fine unless it isn't, unless this estimate is wrong.
It's like, well, okay, well, tell us something new. But in the alignment case, if the estimate is this is what the output should be, unless there's some behavior I don't understand, you want to know in the case, unless there's some behavior you don't understand.
That's not like, oh, whatever. That's like, that's the case in which it's not aligned.
Yeah, I mean, maybe one way of putting it is just like, we can wait until we see this input or like you can wait until you see a weird input and say okay did this weird input do something we didn't understand and for our say that would just be a trivial test you're just like if someone gives you an algorithm you'd be like is it a thing whereas for a neural net in some cases it is like either very expensive to tell or it's like you actually don't have any other way to tell like you checked in easy cases and they're on a hard case so you don't have a way to tell if something has gone wrong um also i would clarify that like i think it is interesting for the remount hypothesis i would say like the current state particularly a number theory but maybe in like quite a lot of math is like there are informal heuristic arguments for like pretty much all the open questions people work on um but those arguments are completely informal so that is like i think there is an it's, it's not the case that there's like, here's the, here's the norms of informal reasoning or the norms of heuristic reasoning. And then we have, we have arguments that like a heuristic argument verifier could accept.
It's just like people wrote some words. I think those words, like my guess would be like, you know, 95% of the things mathematicians accept is like really compelling feeling.
Heuristic arguments are correct. And like, if you actually formalize and be like'd be like some of these aren't quite right or like here's some corrections or here's which of two conflicting arguments is right i think there's something to be learned from it i don't think it would be like mind-blowing though when you have have it completed how big would this heuristic estimator the rules for this heuristic estimator be i mean i i know like when russell and who was the other guy when they did the the rules yeah yeah wasn't it like literally they had like a bucket or a wheelbarrow that with all the papers, but how big would, uh, I mean, mathematical foundations are quite simple in the end.
Like at the end of the day, it's like, you know, how many symbols, like, I don't know, it's hundreds of symbols or something that go into the entire foundations. Um, and the entire rules of reasoning for like, you know, there's a sort of built on top of first order logic, but the rules of reasoning for first order logic are just like, you know, another hundreds of symbols or a hundred lines of code or whatever.
I'd say like, I have no idea. Like we are certainly aiming at things that are just not that complicated.
Like, and my guess is that the algorithms we're looking for are not that complicated. Like most of the complexity is pushed into arguments,, not in this verifier or estimator.
So for this to work, you need to come up with an estimator, which is a way to integrate different touristic arguments together. It has to be a machine that takes its input.
First, it takes its input and argument and decides what it believes in light of it. It's kind of like saying, was it compelling? But second, it needs to take four of those and then say, here's what I believe in light of even though, like, there's a different estimation strategy that produce different numbers.
And that's like a lot of a lot of our life is saying, like, well, here's a simple thing that seems reasonable. And here's a simple thing that seems reasonable.
Like, what are you doing? Like, there's supposed to be a simple thing that unifies them both. And like obstruction to getting that is understanding, like, what happens when these principles are slightly in tension and like, how do we how do we deal? Yeah, that seems super interesting.
Like even I mean, we'll see what other applications it has. I don't know, like computer security and code checking.
If you can actually say like, this is how safe we think a code is in a very formal way. My guess is we're not going to add, I mean, this is both a blessing and a curse.
Like it's a curse and you're like, well, that's sad. Your thing is not that useful, but a blessing and like not useful things are easier.
My guess is we're not going to add that much value in most of these domains. Like, most of the difficulty comes from, like, like, a lot of code that you'd want to verify.
Not all of it, but a significant part is just, like, the difficulty of formalizing the proof is, like, the hard part and, like, actually getting all of that to go through. And, like, we're not going to help even the tiniest bit with that, I think.
So this would be more helpful if you, like, have code that, like, uses simulations. You want to verify some property of, like, a controller that involves some numerical error or whatever.
You need to control the effects of that error. That's where you like start saying like, well, heuristically, if the errors are independent, blah, blah, blah.
Yeah. You're too honest to be a salesman, Paul.
I think this is kind of like sales to us, right? Like if you talk about this idea of people like, why would that not be like the coolest thing ever and therefore impossible? And we're like, well, actually it's kind of lame. And we're just trying to pitch like it's way lamer than it sounds and that's really important to why it's possible is being like it's really it's really not going to blow that many people's i mean i think it will be cool i think it will be like very if we succeed it will be very solid like meta mathematics or theoretical computer science or whatever but i don't think right again i think the mathematicians already do this reasoning and they mostly just love proofs i think the physicists do a lot of reasoning, but they don't care about formalizing anything.
I think like in practice, other difficulties are almost always going to be more salient. I think this is like of most interest by far for interpretability and ML.
and like I think other people should care about it and probably will care about it if successful
but I don't think it's going to be like the biggest thing ever in any field or even like
that huge a thing I think this would be a terrible career move given the like ratio of like difficulty
to but I don't think it's going to be like the biggest thing ever in any field or even like that huge a thing. I think this would be a terrible career move given the like ratio of like difficulty to impact.
I think theoretical computer science, it's like probably a fine move. I think in other domains, like it just wouldn't be worth like we're going to, we're going to be working on this for like years, at least in the best case.
I'm laughing because my next question was going to be like a setup for you to explain. Well, if there student wants to work on this.
It's a terrible career. I think theoretical computer science is an exception where I think this is like in some sense like what the best of theoretical computer science is like.
So like you have all this reason, you have this like, because it's useless. I mean, I think like an analogy, I think like one of the most successful sagas in theoretical computer science is like formalizing the notion of an interactive proof system.
Um, and it's like, you have some kind of informal thing that's interesting to understand and you want to like pin down what it is and construct some examples and see what's possible and what's impossible. And this is like, I think this kind of thing is the bread and butter of like the best parts of theoretical computer science.
And then again, I think mathematicians, like it may be a career mistake because the mathematicians only care about proofs or whatever, but that's a mistake in some sense, aesthetically, like if successful, I do think looking back and again, part of why it's a mistake is such a high probability. We wouldn't be successful, but I think looking back, people would be like, that was pretty cool.
Like, although not that cool. Like, we understand why it didn't happen, given like the epistemic, like what people cared about in the field.
But it's pretty cool now. But isn't it also the case that didn't Hardy write in that, like, you know, all this prime shit is both not useless, but it's fun to do.
And like it turned out that all the cryptography is based on all that prime shit. So I don't know, it could happen.
But anyways, I'm trying to set you up so that you can tell, and forget about, if it doesn't have applications in all those other fields, it matters a lot for alignment. And that's why I'm trying to set you up to talk about if, you know, the smart, I don't know, I think like a lot of smart people listen to this podcast.
If they're a math or CS grad student, um, and has gotten interested in this, uh, are you looking to, uh, potentially find talent to help you with this? Yeah. Maybe we'll start there.
And then I also want to ask you if I think also maybe people who can provide funding might be listening to the podcast. So, uh, to, to both of them, what is your pitch? Yeah, so we're definitely hiring and searching for collaborators.
I think the most useful profile is probably a combination of like intellectually interested in this particular project. I'm motivated enough by alignment to work on this project, even if it's really hard.
I think there are a lot of good problems. So the basic facts that makes this problem unappealing to work on, I'm a really good salesman, but I think the only reason this isn't a slam dunk thing to work on is that like, there are not great examples.
So we've been working on it for a while, but we do not have beautiful results as of the recording of this podcast. Hopefully by the time it airs, it's like they've had great results since then.
But it was too, too, too long to put in the margins of the podcast hopefully by the time it airs you'll have a little subscript that's like they've had great results since then um but it was too too too long to put in the margins of the podcast yeah with luck um yeah so i think it's hard to work on because it's not clear what a success looks like it's not clear if success is possible um but i do think there's like a lot of questions we have a lot of questions. And like, I think like the basic setting of like, look, there are all of these arguments.
So in mathematics, in physics, in computer science, there's just a lot of examples of informal heuristic arguments. They have enough structural similarity that it looks very possible that there is like a unifying framework, that these are instances of some general framework and not just a bunch of random things.
Like not just a bunch of, it's not like, so for example, for the prime numbers, people reason about the prime numbers as if they were like a random set of numbers. One view is like, that's just a special fact about the primes, they're kind of random.
A different view is like, actually it's pretty reasonable to reason about an object as if it was a random object as a starting point. And then as you notice structure, like revised from that initial guess.
And it looks like to me, the second perspective is probably more right. It's just like a reasonable to start off treating an object as random and then like notice perturbations from random, like notice structure the object possesses.
And the primes are unusual in that they have fairly little like additive structure. I think it's a very natural theoretical project.
There's like a bunch of activity that people do. It seems like there's a reasonable chance.
It has some, there's something nice to say about like unifying all of that activity. I think it's a pretty exciting project.
The basic strike against it is that it seems really hard. Like if you were someone's advisor, I think you'd be like, what are you going to prove if you work on this for like the next two years? And they'd be like, there's a good chance, nothing.
And then like, it's not what you do. If you're a PhD student, normally you have like, you aim for those high probabilities of getting something within a couple of years.
The flip side is it does feel, I mean, I think there are a lot of questions. I think some of them we're probably going to make progress on.
So I think the pitch is mostly like, are some people excited to get in now? Or like, are people more like, let's wait to see like, once we have like one or two good successes to see what the pattern is and become more confident, we can turn the crank to make more progress in this direction. But for people who are excited about working on stuff with reasonably high probabilities of failure and not really
understanding exactly what you're supposed to do um i think it's a good i think it's a pretty good
project i feel like if people look back if we succeed and people are looking back in like 50
years on like what was the coolest stuff happening in math with theoretical computer science that
would be like a reasonable this will definitely be like in contention um and like i would guess
for lots of people would just seem like the coolest thing from this period of a couple of years or whatever. Right.
Because this is a new method in so many different fields from the ones you meant, physics, math, theoretical computer science. Like that's really, I don't know, what is the average math PhD working on, right? He's working on like some subset of a subset of something I can't even understand or pronounce.
Math is quite esoteric. But yeah, this seems like, I don't know, even the small chance of it working, like forget about the value for, you shouldn't forget about the value for alignment.
But even without that, this is such a cool, if this works, it's like a really big, it's a big deal. There's a good chance that if I had my current set of views about this problem and didn't care about alignment and had the career safety to just like spend a couple of years thinking about it, or, you know, spend half my time for like five years or whatever, that I would just do that.
I mean, even without caring at all about alignment, it's just a nice, it's a very nice problem. It's very nice to have this like library of things that succeed where like, it's just, they feel so tantalizingly close to being formalizable least to me um on such a natural setting and then just have so little purchase on it it's like a there aren't that many like really exciting feeling frontiers in like theory of computer science all right and then so a smart person doesn't have to be a grassroot but like a smart person is interested in this what should they do should they try to attack some open problem you have put on your blog or should it, what is the next step? Um, yeah, I think like a first pass step, like there's different, there's different levels of ambition or whatever, different ways of approaching our problem.
But like, we have this write up of like from last year, um, or I guess 11 months ago or whatever, um, on formalizing the presumption of independence that provides like, here's kind of a communication of what we're looking for in this object. And like, I think the motivating problem is saying like, here's a notion of what an estimator is, and here's what it would mean for an estimator to capture some set of informal arguments.
And like a very natural problem is just try and do that, like go for the whole thing, try and understand, and then like come up with up with like hopefully a different approach or then like end up having context from a different angle on the kind of approach we're taking i think that's a reasonable thing to do um i do think we also have a bunch of open problems um so maybe we should put up more of those open problems and the main concern with doing so is that for any given one we're like this is probably hopeless um like put up a prize earlier in the year for an open problem which tragicallyically, I mean, I guess the time is now to post the debrief from that, or I owe it from this weekend. I was supposed to do that.
So I'll probably do it tomorrow. But no one solved it.
It's sad putting out problems that are hard, or like I don't, we could put out a bunch of problems that we think might be really hard. But what was that famous case of that statistician who, it was like some PhD student who showed up late to a class and he saw some problems in the ward and he thought they were homework and then they were actually just open problems and then he solved them because he thought they were homework, right? Yeah.
I mean, we don't, we have much less information that these problems are hard. Like, again, I expect the solution to most of our problems to not be that complicated.
We have not, and we've been working on it in some sense for a really long time. Um, like, you know, total years of full-time equivalent work across the whole team is like probably like three years of full-time equivalent work in this area, um, spread across a couple of people, but like, that's very little compared to a problem.
Like it is very easy to have a problem where you put in three years of full-time equivalent work, but in fact, there's still an approach that's going to work quite easily with like three to six months if you come at a new angle and like we've learned a fair amount from that that we could share and we probably will be sharing more over the coming months um as far as funding goes is this something where i don't know if somebody gave you a whole bunch of money that would help or does it not matter how many people are working on this by the way so we have been right now there's four of us full-time and we're hiring for more people. And then is funding that would matter? I mean, funding is always good.
We're not super funding constrained right now. The main effect of funding is it will cause me to continuously and perhaps indefinitely delay fundraising.
Periodically, I'll set out to be interested in fundraising and someone will be like, offer a grant. And then I will get to delay for another six months or fundraising or nine months or whatever.
So you can delay the time at which Paul needs to think for some time about fundraising. Well, one question I think it'd be interesting to ask you is, you know, I think people can talk vaguely about the value of theoretical research and how it contributes to real world applications.
And, you know, you can look at historical examples or something, but you are somebody who actually has done this in a big way like rlhf is you know something you developed and then it actually has got into an application that has been used by millions of people so tell me about like you just it's just that pipeline how can you reliably identify theoretical problems that will matter for real applications because it's one thing to like read about touring or something and the the the halting problem but like here you have the real thing yeah i mean it is definitely exciting to have worked on a thing that has a has a real world impact the main caveat i provide is like our lead chef is very very simple um compared to many things um and like so the motivation for working on that problem was like look this is how it probably should work, or this is a step in some progression. It's unclear if it's the final step or something, but it's a very natural thing to do that people probably should be and probably will be doing.
I'm saying, if you want to talk about crazy stuff, it's good to help make those steps happen faster. And it's good to learn about, there's lots of issues that occur in practice, even for things that seem very simple on paper.
But mostly the story is just like, yep, I think my sense of the world is things that look like good ideas on paper just often are harder than they look, but the world isn't that far from what makes sense on paper. Large language models look really good on paper, and RLH looks really good on paper.
And these things things like i think just work out in a way that's yeah i think people maybe overestimate or like maybe it's like kind of a trope but people talk about like it's easy to like underestimate how much gap there is to practice like how many things will come up that don't come up in theory but it's also like easy to overestimate like how inscrutable the world is like the things that happen mostly are things that do just kind of make sense. Yeah, I feel like most ML implementation does just come down to a bunch of detail, though, of like, build a very simple version of the system, understand what goes wrong, fix the things that go wrong, scale it up, understand what goes wrong.
And I'm glad I have some experience doing that, but I don't think, I think that does cause me to be better informed about what makes sense in ML and what can actually work. But I don't think it caused me to have like a whole lot of deep expertise or like deep wisdom about like how to close the gap.
Yeah, yeah. But is there some tip on identifying things like RLHF which actually do matter versus making sure you don't get stuck in some theoretical problem that doesn't matter? Or is it just coincidence? Or I mean, is there something you can do in advance to make sure that the thing is useful? I don't know if the RLHF story is like the best, best success case or something, but like, Oh, because the, the, the, the capabilities.
Maybe I'd say more profoundly, like, again, it's just not that hard a case. It was like a little bit, it's a little bit unfair to be like, I'm going to predict'm going to predict the thing which i like i pretty much think it was going to happen at some point um and so it was mostly a case of acceleration whereas like the work we're doing right now is specifically focused on something that's like kind of crazy enough that it might not happen even if it's a really good idea or challenging enough it might not happen but i'd say like in general like and this draws a little bit on like more broad experience more broadly in theory.
It's just like a lot of the times when theory fails to connect with practice, it's just kind of clear. It's not going to connect.
If you like try, if you actually think about it and you're like, what are the key constraints in practice? Is the theoretical problem we're working on actually connected to those constraints? Is there a path? Like, is there something that is possible in theory that would actually address like real world real-world issues? I think, like, the vast majority, like, as a theoretical computer scientist, the vast majority of theoretical computer science, like, has very little chance of ever affecting practice, but also it is completely clear in theory that there's very little chance of affecting practice. Like, most of the theory fails to affect practice, not because of, like, all the stuff you don't think of, but just because, like, it was, like, you could call it, like, dead on arrival, but you could also just be, like, it's not really the point.
It's just like mathematicians also are like, they're not trying to affect practice and they're not like, why does my number theory not affect practice? It was kind of obvious. Um, I think the biggest thing is just like actually caring about that.
And then like learning at least what's basically going on in the actual systems you care about and what are actually the important constraints. And is this a real theoretical problem? The basic reason most theory doesn't do that is just like, that's not where the easy theoretical problems are.
So I think theory is instead motivated by like, we're gonna build up the edifice of theory. And like, sometimes they'll be opportunistic, like opportunistically we'll find a case that comes close to practice, or we'll find something practitioners are already doing and try and bring it into our framework or something.
But the theory of change is mostly not this thing that's gonna make it into practice. It's mostly this is going to contribute to the body of knowledge that will slowly grow and like sometimes opportunistically yields important results.
How big would do you think a seed AI would be? What is the minimum sort of encoding of something that is as smart as a human? I think it depends a lot what substrate it gets to run on. So if you tell me like like how much computation does it get before or like what kind of real world infrastructure does it get? Like you could ask what's the shortest program which like if you run it on a million H100s connected in like a nice network with like a hospitable environment will eventually like go to the stars.
But that seems like it's probably on the order of like tens of thousands of bytes or I don't know. If I had to guess the median I'd guess 10,000 bytes.
Wait, the specification or the compression of? Just the program, a program which went wrong.
Oh, got it, got it, got it.
But that's going to be like really cheat-sy.
So the task, what's the thing that has values
and will like expand and like roughly preserve its values
as it proceeds?
Yeah, exactly. I thought about that task.
Because like that thing, the 10,000 byte thing,
we'll just lean heavily on like evolution
and natural selection until you get there.
For that, like, I don't know, million bytes? Million bytes. 100, hundred thousand bytes something like that how do you think AI lie detectors will work where you kind of just look at the activations and not in the not find explanations in the way you were talking about with heuristics but literally just like here's what truth looks like here what lies look like, let's just segregate the latent space and see if we can identify the two.
Yeah, I think to separate the, like just train a classifier to do it is like a little bit complicated for a few reasons and like may not work, but if you just like broaden the space and say like, hey, it's like you wanna know if someone's lying, you get to interrogate them, but also you get to like rewind them arbitrarily and make a million copies of them. I do think it's like pretty hard to lie successfully.
You get to like look at their brain, even if you don't quite understand what's happening, you get to rewind them a million times. You get to like run all those parallel copies and do gradient descent or whatever.
I think there's a pretty good chance that you can just tell if someone is lying, like a brain emulation or an AI or whatever, unless they were like aggressively selected. Like if it's just they are trying to lie well, rather than it's like they were selected over many generations to be excellent at lying or something, then like your ML system, hopefully you didn't train it a bunch to lie and you want to be careful about whether your training scheme effectively does that.
But yeah, that seems like it's more likely than not to succeed. And how possible do you think it will be for us to specify human verifiable rules for reasoning such that even if the AI is super intelligent, we can't really understand why there are certain things.
We know that the way in which it arises at these conclusions is valid. Like if it's trying to persuade us to something, we can be like, I don't understand all the steps, but I know that this is something that's valid and you're not just making shit up.
That seems very hard if you wanted to be competitive with learned reasoning. So like, I don't, I mean, it depends a little bit exactly how you set it up, but for like the ambitious versions of that that say would address the alignment problem, they seem pretty unlikely, you know, like five, 10% kind of thing.
Is there an upper bound on intelligence no not in the near term but just like super intelligence at some point like how how far do you think that can go it seems like it's going to depend a little bit on what is meant by intelligence it kind of reads as a question similar to like is there an upper bound on like strength or something like there are a lot of forms i think it's like the case that for yeah i think there are like sort of arbitrarily smart input output functionalities. And then like, if you hold fixed the amount of compute, there is some smartest one.
If you're just like, what's, what's the best set of like 10 to the 40th operations? There's just, there's only finally many of them. So some like best one for any particular notion of best that you have in mind.
So I guess like, I'm just like for the unbounded question, we're allowed to use arbitrary description, complexity and compute, like probably now. And for the, I mean, there is some is some like optimal conduct like if you're like I have some goal in mind and I'm just like what action best achieves it if you imagine like a little box embedded in the universe I think there's kind of just like an optimal input output behavior so I guess in that sense I think there is an upper bound but it's not saturatable in the physical universe because it's definitely exponentially slow.
Right yeah yeah or you know because of comms or other things or heat there it just might be physically impossible to insatuate something smarter than this yeah i mean like for example if you if you imagine what the best thing is it would almost certainly involve just like simulating every possible universe it might be in modular like moral constraints which i don't know if you want to include them but like so that would be very very slow it would involve sim, you know, it's sort of like, I don't know exactly how slow, but like double exponential, very slow. Carl Schulman laid out his picture of the intelligence explosion in the seven hour episode.
What, I know you guys have talked a lot. What about his basic picture? Like, do you have some main disagreements? Is there some crux that you guys have explored? It's related to our timelines discussion from earlier.
I think the biggest issue is probably error bars, where Carl has a very software-focused, very fast takeoff picture. And I think that is plausible, but not that likely.
I think there's a couple of ways you could perturb the situation, and my guess is one of them applies. So maybe I have, like, I don't know exactly what Carl's probability is.
I feel like Carl's going to have, like, a 60% chance on some crazy thing that I'm only going to assign, like, a 20% chance to or a 30% chance or something. And, like, I think those kinds of perturbations are, like, one, how long a period is there of complementarity between AI capabilities and human capabilities, which will tend to soften takeoff? Two, how much diminishing returns are there on software progress, such that is a broader takeoff involving scaling electricity production and hardware production? Is that likely to happen during takeoff, where I'm more like 50-50 or more? Stuff like this.
Yeah, okay. So is it that you think the ultimate constraints will be more hard or like the the basic case he's laid out is that you can just have a sequence of things like uh flash attention or moe uh and you can just keep stacking these kinds of things on i'm very unsure if you can keep stacking them or like it's kind of a question of what's like the returns curve and like carl has some inference from historical data some way he'd extrapolate the trend.
I'm more unsure if you can keep stacking them. It's kind of a question of what's the returns curve.
And Carl has some inference from historical data
or some way he'd extrapolate the trend.
I'm more like 50-50 on whether the software-only intelligence explosion
is even possible.
And then a somewhat higher probability that it's slower.
Why do you think it might not be possible?
Well, so the entire question is, if you double R&D effort,
do you get enough additional improvement to further double the efficiency?
And that question will itself be a function of your hardware base
Thank you. is like if you double R&D effort, do you get enough additional improvement to further double the efficiency? And like that's that question will itself be a function of your hardware base, like how much hardware you have.
And the question is like at the amount of hardware we're going to have and the level of sophistication we have as the process begins, like is it the case that each doubling of actually the initials only depends on the hardware or like each level of hardware will have some place at this dynamic asymptotes. So the question is just like, for how long is it the case that each doubling of R&D at least doubles the effective output of your AI research population? And I think like I have a higher probability on that.
Like, and it's kind of close if you look at the empirics, I think the empirics benefit a lot from like continuing hardware scale up. So that like the effective R&D stock is like significantly smaller than it looks, if that makes sense uh what are the impairs you're referring to um so there's kind of two sources of evidence one is like looking across a bunch of industries at like what is the general improvement with each doubling of like either r d investment or experience where like it is quite exceptional to have a field with not anyway it's pretty good to have a field where each time you double r d investment you get a get a doubling of efficiency.
The second source of evidence is on like actual, like algorithmic improvement in ML, which is obviously much, much scarcer. And they're like, you can make a case that it's been like each doubling of R and D has given you like roughly a four X or something increase in computational efficiency.
But like, there's a question of how much that benefits. When I say the effect of R and D stock is smaller.
I mean like we scale up,
like you're doing a new task, like every couple of years we're doing a new task because you're operating a
scale much larger than the previous scale.
And so a lot of your effort is how to make use of the new scale.
So if you're not increasing your installed hardware base and are just flat
at a level of hardware,
I think you get like much faster diminishing returns than people have gotten
historically. I think Carl agrees in principle, this is true.
And then once you make that adjustment,
I think it's like a very unclear where the empirics shake out.
I think Carl has thought about these more than I am,
so I should maybe defer more.
But anyway, I'm at like 50-50 on that.
How have your timelines changed over the last 20 years?
Last 20 years?
Yeah.
How long have you been working on anything related to AI?
So I started thinking about this stuff in like 2010 or so.
So I think my first, my earliest timeline prediction will be in like 2011. I think in 2011, my like rough picture was like, we will not have insane AI in the next 10 years.
And then like I get increasingly uncertain after that, but like we converted to like, you know, 1% per year or something like that. And then probably in 2016, my take was like,
we won't have crazy AI in the next five years, but then we converged to like one or 2% per year after that. Um, then in 2019, I guess I made a round of forecasts, uh, where I gave like
30% or something to 25% to crazy AI by 2040 and like 10% by 2030 or something like that. So I think my 2030 probability has been kind of stable and my 2040 probability has been going up.
And I would guess it's too sticky. I guess that 40% I gave at the beginning is just like from not having updated recently enough.
And I maybe just need to sit down. I would guess that should be even higher.
I think like 15% in 2030 I'm not feeling that bad about. This is just like each passing year is like a big update against 2030.
Like we don't have that many years left. And that's like roughly counterbalanced with AI going pretty well.
Whereas for like the 2040 thing, like the passing years are not that big a deal. And like as we see that like things are basically working, that's like cutting out a lot of the probability of not having AI by 2040.
So yeah, my 2030 probability up a little bit, like maybe twice as high as it used to be or like something like that. My 2040 probability like up more, much more significantly.
How fast do you think we can keep building fabs to keep up with the AI demand? Yeah, I don't know much about any of the relevant areas. My like best guess my understanding is right now 5% or something of the next year's total or best process fabs will be making AI hardware, of which only a small fraction will be going into very large training runs, so maybe a couple percent of total output.
And then represents maybe like 1% of total possible output, a couple of percent of like leading process, 1% of total or something. I don't know if that's right, but I think that's like the rough ballpark we're in.
I think things will be like pretty fast as you scale up for like the next order of magnitude or two from there, because you're basically just shifting over other stuff. My sense is it would be like years of delay.
There's like multiple reasons that you expect years of delay for going past that. Maybe even at that you start having, yeah, there's just a lot of problems.
Like building new fabs is quite slow. And I don't think there's like, TSMC is not like planning on increases in total demand driven by AI, like kind of conspicuously not planning on it.
I don't think anyone else is really ramping up production in anticipation either. So I think, and then similarly, just building data centers of that size seems very, very hard and also probably has multiple years of delay.
What does your portfolio look like? I've tried to get rid of most of the AI stuff that's plausibly implicated in policy work or advocacy on the RSP stuff for my involvement with Anthropic. What would it look like if you had no conflicts of interest? And no inside information.
Like, I also still have a bunch of hardware investments, which I need to think about. But like, I don't know.
A lot of TSMC. I have a chunk of NVIDIA, although I keep I just keep betting against NVIDIA constantly since 2016 or something.
I've been destroyed on that bet.
Although AMD has also done fine.
And it's like, well, now the case now is even easier,
but it's similar to the case in the old days.
Just a very expensive company,
given the total amount of R&D investment they've made.
They have like whatever, a trillion dollar valuation or something.
That's like very high.
So the question is like, how expensive is it to like make a TPU
such that it's like actually outcompetes H100 or something? And I'm like, wow. It's real high level of incompetence if Google can't catch up fast enough to make that trillion dollar valuation not justified.
Whereas with TSMC, they have a harder remote, you think? Yeah, I think it's a lot harder, especially if you're in this regime where you're trying to to scale up so like if you're unable to build fabs i think it will take a very long time to build as many fabs as people want like the effect of that will be to like bid up the price of existing fabs and existing semiconductor manufacturing equipment and so like just those hard assets will become like spectacularly valuable as well the existing like gpus and like the actual yeah um yeah i think it's just hard that seems like the hardest asset to scale up quickly, so it's like the asset, if you have a rapid run-up, it's the one that you'd expect to most benefit. Whereas Nvidia's stuff will ultimately be replaced by either better stuff made by humans or stuff made with AI assistants.
The gap will close even further as you build AI systems. Right, unless Nvidia's using those systems.
Yeah. Yeah, the point is just like the future R&D will so dwarf past R&D.
Right, I see. And there's like just not that much stickiness.
There's less stickiness in the future than there has been in the past. Like, yeah.
I don't know. So I don't want to, not commenting from any private information, just in my gut.
Having caveat of this is like the single bet I've most lost. Like not including NVIDIA in that portfolio.
And final question, there's a lot of schemes out there for alignment, and I think just like a lot of general takes. And a lot of this stuff is over my head where I think I literally, it took me like weeks to understand the mechanistic anomaly stuff you work on.
Without spending weeks, how do you detect bullshit? Like people have explained their schemes to me, and I'm like, honestly, I don't know if it makes sense or not. With with you i'm just like i trust paul enough that i think there's probably something here if i try to understand this enough but without yeah how do you how do you detect bullshit yeah so i think it depends on the kind of work so for like the kind of stuff we're doing my guess is like most people there's just not really a way you're going to tell whether it's bullshit so i think like it's important that we don't spend that much money on like the people who want to hire are probably going to dig in in depth i don't think there's a way you can tell whether it's bullshit with without either spending like a lot of effort or leaning on deference um with empirical work it's like interesting and that you do have some signals of the quality of work like you can be like i mean is it does it work in practice like does the story i think the stories are just radically simpler and so you probably can evaluate those stories like on their face.
And then you mostly come down to these questions about like, what are the key difficulties? Yeah. I tend to like be optimistic when people dismiss something because like this doesn't deal with a key difficulty or this runs into the following and super bowl obstacle.
I tend to be like a little bit more skeptical about those arguments and tend to think like, yeah, something can be bullshit because it's not addressing a real problem. That's like, I think the easiest way, like this is a problem someone's interested in.
That's just like not actually an important problem. And there's no story about why it's going to become an important problem.
EG, like it's not a problem now and won't get worse, or it is maybe a problem now, but it's clearly getting better. Um, that's like one way.
And then conditional on like passing that bar, like dealing with something that actually engages with like important parts of the argument for concern. And then like actually making sense empirically.
So like I think most work is anchored by its source of feedback is like actually engaging with real models. So it's like, does it make sense to have engaged with real models? And does the story about how it like deals with key difficulties actually make sense? I'm like pretty liberal past there.
I think it's really hard to like, you do people look at mechanistic interpretability and be like well this obviously can't succeed and I'm like I don't know how can you tell it obviously can't succeed like I think it's reasonable to take total investment in the field like how fast is it making progress like how does that pencil I think like most things people work on they're actually pencil like pretty fine like they look like they could be reasonable investments. Things are not like super out of whack.
Okay, great. This is, I think, a good place to close.
Paul, thank you so much for your time. Yeah, thanks for having me.
It was good chatting. Yeah, absolutely.
Hey, everybody. I hope you enjoyed that episode.
As always, the most helpful thing you can do is to share the podcast. Send it to people you think might enjoy it.
Put it in Twitter, your group chats, etc. Just blitz the world.
Appreciate you listening. I'll see you next time.
Cheers.