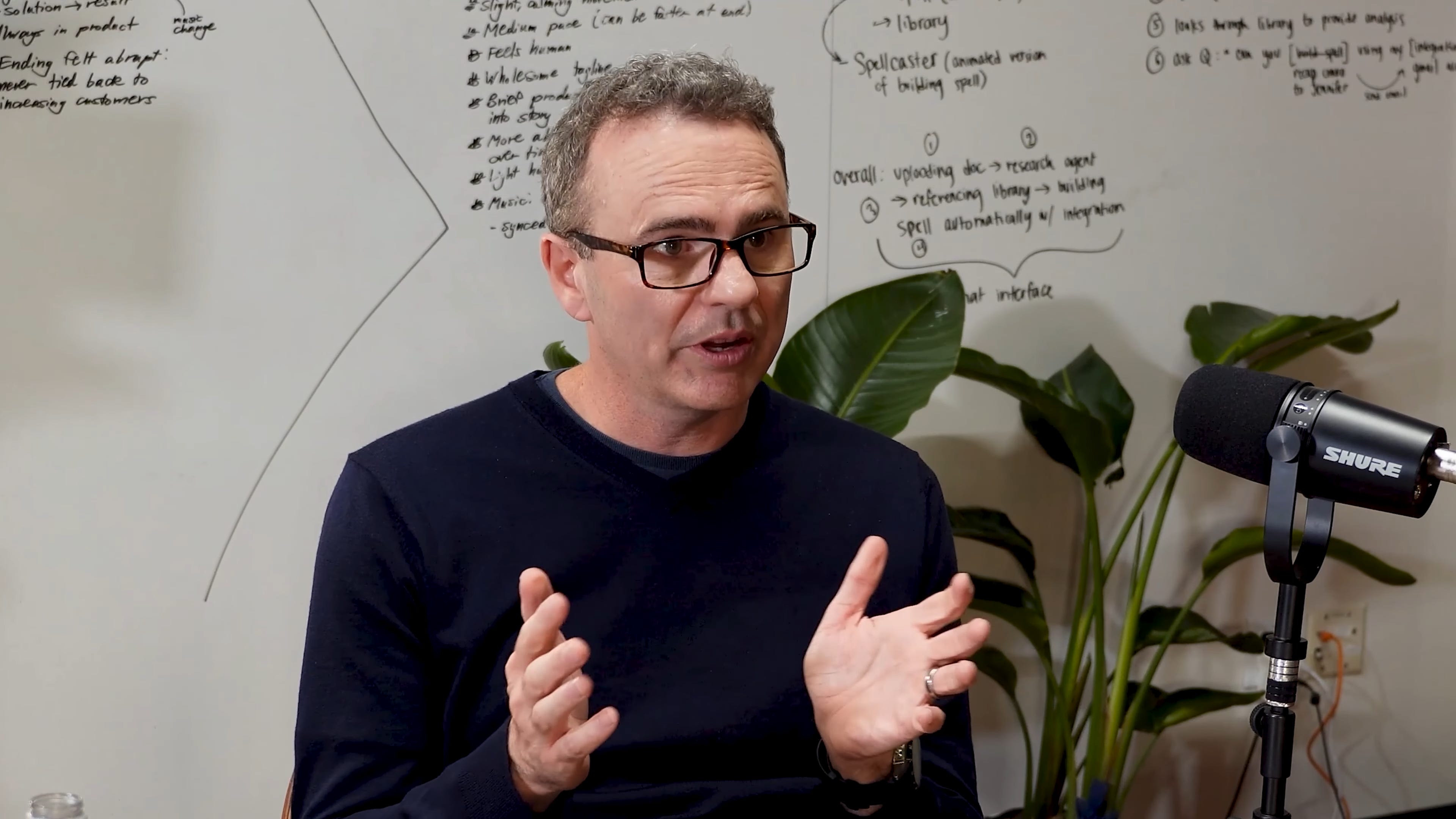
Shane Legg (DeepMind Founder) - 2028 AGI, New Architectures, Aligning Superhuman Models
I had a lot of fun chatting with Shane Legg - Founder and Chief AGI Scientist, Google DeepMind!
We discuss:
* Why he expects AGI around 2028
* How to align superhuman models
* What new architectures needed for AGI
* Has Deepmind sped up capabilities or safety more?
* Why multimodality will be next big landmark
* and much more
Watch full episode on YouTube, Apple Podcasts, Spotify, or any other podcast platform. Read full transcript here.
Timestamps
(0:00:00) - Measuring AGI
(0:11:41) - Do we need new architectures?
(0:16:26) - Is search needed for creativity?
(0:19:19) - Superhuman alignment
(0:29:58) - Impact of Deepmind on safety vs capabilities
(0:34:03) - Timelines
(0:41:24) - Multimodality
Get full access to Dwarkesh Podcast at www.dwarkesh.com/subscribe
Listen and Follow Along
Full Transcript
Okay, today I have the pleasure of interviewing Shane Legg, who is a founder and the chief AGI scientist of Google DeepMind. Shane, welcome to the podcast.
Thank you. It's a pleasure to be here.
So first question, how do we measure progress towards AGI concretely? So we have these loss numbers, and we can see how the loss improves from one model to another. But it's just a number.
How do we interpret this? How do we see how much progress we're actually making? That's a hard question, actually. AGI, by its definition, is about generality.
So it's not about doing a specific thing. It's much easier to meet your performance when you have a very specific thing in mind because you can construct a test around that.
Well, maybe I should first of all explain what do I mean by AGI? Because there are a few different notions around. When I say AGI, I mean a machine that can do the sorts of cognitive things that people can typically do, possibly more.
But that's to be an AGI, that's kind of the bar you need to meet. So if we want to test whether we're meeting this threshold or we're getting close to this threshold, what we actually need then is a lot of different kinds of measurements and tests that spans the breadth of all the sorts of cognitive tasks that people can do.
And then to have a sense of what is human performance on these sorts of tasks.
And that then allows us to sort of judge whether or not we're there.
It's difficult because you'll never have a complete set of everything that people do because it's such a large set.
But I think that if you ever get to the point where you have a pretty good range of tests of all sorts of different things that people do, cognitive things people do, and you have an AI system which can meet Hume performance and all those things. And with some effort, you can't actually come up with new examples of cognitive tasks where the machine is below Hume performance.
At that point, it's conceptually possible that there is something that the machine can't do that people can do. But if you can't find it with some effort, I think for practical purposes, you now have an AGI.
So let's get more concrete. And, you know, we measure the performance of these large language models on MMLU or something.
And maybe you can explain what all these different benchmarks are. But the ones we use right now that you might see in a paper, what are they missing? What aspect of human cognition do they not measure adequately? Oh, yeah, another hard grease.
These are quite big areas. So they don't measure things like understanding streaming video, for example, because these are language models and people can do things like understand extreme video.
They don't do things like humans have what we call episodic memory, right? So we have a working memory, which are things that have happened quite recently. And then we had sort of cortical memory.
So these are things that have sort of been, you know, in our cortex that have been, but there's also a system in between, which is episodic memory, which is the hippocampus.
And so this is about learning specific things very, very rapidly.
So some of the things I say to you today,
if you remember them tomorrow,
that'll be your episodic memory hippocampus.
Our models don't really have that kind of thing.
And we don't really test for that kind of thing.
We just sort of try to make the context windows,
which is, I think, more like a working memory,
longer and longer to sort of compensate for this.
But, yeah, we don't really test for that kind of a thing. So there is all sorts of bits and pieces.
But, you know, it is a difficult question because you really need to, as I said, intelligence, the generality of human intelligence is very, very broad. So you really have to start going into the weeds of trying to find if there's specific types of things that are missing from existing benchmarks or different categories of benchmarks that you know aren't don't currently exist or something yeah the thing you're referring to with episodic memory would it be fair to call that sample efficiency or is that a different uh it's it's very much related to sample efficiency it's it's one of the things that enables humans to be very sample efficient.
Right.
Large language models have a certain kind of sample efficiency
because when something's in their context window,
that sort of biases the distribution to behave in a different way.
And so that's a very rapid kind of learning.
So there are multiple kinds of learning,
and the existing systems have some of them but not others. So it's a little bit complicated.
So this kind of memory or we call it sample efficiency, whatever. Is it a fatal flaw of these deep learning models that it just takes trillions of tokens, far more, many more orders of magnitude more than a human will see throughout their lifetime? is this something that just solved over time so the models can learn things immediately when it's in a context window and then they have this sort of this longer process of when you actually train the base model and so on um and that's they're learning over trillions of tokens but they sort of miss something in the middle right right that's sort of what i'm getting at here.
I don't think it's a fundamental limitation.
I think what's happened with large language models is something fundamental has changed. We know how to build models now that have some degree of, I would say, understanding of what's going on.
And that did not exist in the past. And because we've got a scalable way to do this now, that unlocks lots and lots of new things.
Now, we can then look at things which are missing, such as this sort of episodic memory type thing, and we can then start to imagine ways to address that. So my feeling is that there are kind of relatively clear paths forwards now to address most of the shortcomings we see in existing models, whether it's about delusions, factuality, the type of memory and learning that they have, or understanding video or all sorts of things like that.
So I don't see there are big blockers here. I don't see big walls in front of us.
I just see there is more research and work, and these things will improve and probably be adequately solved. But going back to the original question of how do you measure when human-level AI is arrived or beyond it? As you mentioned, there's these other sorts of benchmarks you can use and other sorts of traits.
But concretely, what would it have to do for you to be like, okay, we've reached human level? Would it have to beat Minecraft from start to finish? Would it have to get 100% on MMLU? What would it have to do? There is no one thing that would do it because I think that's the nature of it. It's about general intelligence, so I'd have to make sure it could do lots and lots of different things and it didn't have a gap.
We already have systems that can do very impressive categories of things to human level or even beyond. So I would want a whole suite of tests that I felt was very comprehensive.
And then furthermore, when people come in and say, okay, so it's passing a big suite of tests, let's try to find examples. Let's take an adversarial approach to this.
Let's deliberately try to find examples where people can clearly typically do this, but the machine fails. And when those people cannot succeed, I'll go, okay, we're probably there.
A lot of your early research, at least on that, Zach, I find emphasized that AI should be able to manipulate and succeed in a variety of open-ended environments. It kind of sounds like a video game almost.
Is that where your head is still at now, or do you think about it differently? Yeah, it's evolved a bit. When I did my thesis work around universal intelligence and so on, I was trying to come up with an extremely universal, general, mathematically clean framework for defining and measuring intelligence.
And I think there were aspects of that that were successful. I think in my own mind, it clarified the nature of intelligence as being able to perform well in lots of different domains and different tasks and so on.
It's about that sort of capability of performance and the breadth of performance. So I found that was quite helpful and enlightening.
There was always the issue of the reference machine because in the framework, you have a weighting of things according to their complexity. It's like an Occam's razor type of thing where you weight tasks, environments, which are simpler, more highly in this sort of because you've got an infinite, it's a countable space of different computable environments, of semi-computable environments.
And that Combo-Groaf Complexity measure has something built into it,
which is called a reference machine.
And that's a free parameter.
So that means that the intelligence measure
has a free parameter in it.
And as you change that free parameter,
it changes the weighting and the distribution
over the space of all the different tasks and environments.
So this is sort of an unresolved part of the whole problem. So what reference machine should we ideally use? There's no universal, like one specific reference machine.
People will usually put a universal Turing machine in there, but there are many kinds of universal Turing machines. You have to put a universal Turing machine in there, but there are many different ones.
So I think given that it's a free parameter, I think the most natural thing to do is say, okay, let's think about what's meaningful to us in terms of intelligence. I think human intelligence is meaningful to us and the environment that we live in.
We know what human intelligence is. We are human too.
We interact with other people who have human intelligence. We know that human intelligence is possible, obviously, because it exists in the world.
We know that human intelligence is very, very powerful because it's affected the world profoundly in countless ways. And we know if human level intelligence was achieved, that would be economically transformative because the types of cognitive tasks people do in the economy could be done by machines then.
And it would be philosophically important because this is sort of how we often think about intelligence. And I think historically would be a key point.
So I think that human intelligence is actually quite, in a human-like environment, is quite a natural sort of reference point. So you could imagine sort of setting your reference machine to be such that it emphasizes the kinds of environments that we live in, as opposed to some abstract mathematical environment or something like that.
And so that's how I've kind of gone on this journey of let's try to define a completely universal, clean mathematical notion of intelligence to, well, it's got a free parameter. One way of thinking about it is say, okay, let's think more concretely now about human intelligence.
And can we build machines that can match human intelligence? Because we understand what that is. We know that that is a very powerful thing.
And it has economic, philosophical, historical kind of importance. So that's kind of the...
And the other aspect, of course, is that in this pure formulation of commonwealth complexity, it's actually not comput and you know i obviously knew that there was a there was a limitation at the time but it was a it was an effort to say okay can we just even very theoretically come up with a clean definition i think we can sort of get there we have this issue of a of a reference machine which is unspecified so before we move on i do want to ask uh on the original point you made about these machines or these LLMs need episodic memory. You said that these are problems that we can solve.
These are not fundamental impediments. But when you say that, do you think they'll just be solved by scale? Or do each of these need a fine grain specific solution that is architectural in nature i i think it'll be architectural in nature because the well the current architectures they they don't really have what you need to do this they basically have a context window which is very very fluid of course and they have the weights which things get baked into very slowly so to my mind it feels like working memory which is like the the activations in your brain and then the weights, the syn get baked into very slowly.
So to my mind, that feels like working memory, which is like the activations in your brain, and then the weights, the synapses and so on in your cortex. Now, the brain separates these things out.
It has a separate mechanism for rapidly learning specific information because that's a different type of optimization problem compared to slowly learning deep generalities, right? That's sort of, there's a tension between the two, but you want to be able to do both. You want to be able to, I don't know, hear someone's name and remember it the next day.
And you also want to be able to integrate information over a lifetime so you start to see deeper patterns in the world. These these are quite different different um optimization targets different you know processes but a comprehensive system should be able to do both and so i think it's it's conceivable you could build one system does both but you can see because they're quite different things that it makes sense for them to be different i think that's why the brain does it separately i'm curious about how concretely you think that would be achieved and i'm specifically curious um i guess you can answer this as part of the answer you know deep mind has been working on these domain specific reinforcement learning type setups alpha full alpha code and so on how does that fit into what you see as a path to agi have these just been-specific models, or do they feed into the eventual AGI? Things like AlphaFold are not really feeding into AGI.
We may learn things in the process that may end up being relevant, but I don't see them as being likely being on the path to AGI.
But, yeah, we're a big group. We've got hundreds and hundreds and hundreds of PhDs working on lots of different projects.
So, you know, when we find, you know, what we see like opportunities to do something significant like Alpha Fold, we'll go and do it. It's not like we only do AGI type work.
We work on fusion reactors and various things in sustainability, energy. We've got people looking at satellite images of deforestation.
We have people looking at weather forecasting. We've got tons of people looking at lots of things.
On the point you made earlier about the reference class or the reference machine as human intelligence, it's interesting because in your 2008 thesis, one of the things you mentioned almost as a side note is, well, how would you measure intelligence? And you said, well, you could do a compression test and you could see if it fills in words in a sample of text and that could measure intelligence. And funnily enough, that's basically the limbs are trained at the time did it stick out to you as a especially fruitful thing to train for well yeah i mean in a sense what's happened is actually very aligned with um what i write about my thesis which are the ideas from marcus hutter um with aix where uhv Induction, which is this incomputable, but theoretically very elegant and extremely sample-efficient prediction system.
And then once you have that, you can build a general agent on top of it by basically adding search and reinforcement signal. That's what you do with AIXC.
But what that sort of tells you is that if you have a fantastically good sequence predictor, some approximation of solomonas conduction, then going from that to a very powerful, very general AI system, AGI system is just sort of another step. You've actually solved a lot of the problem already.
And I think that's what we're seeing today, actually, that these incredibly powerful foundation models are incredibly good sequence predictors that compressing the world based on all this data. And then you will be able to extend these in different ways and build very, very powerful agents out of them.
Okay, let me ask you more about that. So Richard Sutton's Bitter at Less Than Essay says that there's two things you can scale, search and learning.
And I guess you could say that LLMs are about the learning aspect. The search stuff, which you've worked on throughout your career, where you have an agent that is interacting with this environment?
And is that the direction that needs to be explored again?
Or is that something that needs to be added to alums where they can actually interact with their data or the world or in some way?
Yeah, I think that's on the right track.
I think these foundation models are world models of a kind.
And to do really creative problem solving, you need to start searching. So if I think about something like AlphaGo and the Move 37, the famous Move 37, where did that come from? Did that come from all its data that it's seen of human games or something like that? No, it didn't.
It came from it identifying a move as being quite unlikely, but possible. And then via a process of search, coming to understand that that was actually a very, very good move.
So you need to get real creativity. You need to search through spaces of possibilities and find these sort of hidden gems.
That's what creativity is. I think current language models, they don't really do that kind of a thing.
They really are mimicking the data. They are mimicking all the human ingenuity and everything, which they have seen from all this data that's coming from the internet that's originally derived from humans.
If you want a system that can go truly beyond that and not just generalize in novel ways, so these models can blend things, they can do Harry Potter in the style of a Kanye West rap or something, even though it's never happened, they can blend things together. But to do something that's truly creative that is not just a blending of existing things, that requires searching through a space of possibilities and finding these hidden gems that that are sort of the hidden away in there somewhere and that requires search so i don't think we'll see systems that truly step beyond their training data until we have powerful search in the process so there are rumors that googleMind is training newer models and you don't have to comment on those specifically, but when you do that, if it's the case that search or something like that is required to go to the next level, are you training in a completely different way than say GPT-4 or other transformers are trained? I can't say much about how we're training.
I think it's fair to say we're doing the sorts of scaling and training roughly that you see many people in the field doing. But we have our own take on it and our own different tricks and techniques.
Okay, maybe we'll come back to it and get another answer on that. But let's talk about alignment briefly.
So what will it take to align human level and superhuman AIs? And, you know, it's interesting because the sorts of reinforcement learning and self-play kinds of setups that are popular now, like Constitution AI or LHF, DeepMind obviously has expertise in it for decades longer. So I'm curious what you think of the current landscape and how DeepMind pursues that problem of safety towards human level models.
So do you want to know about what we're currently doing or do you want me to have a stab at what I think needs to be done? Needs to be done. Needs to be done.
So I mean, in terms of what we're currently doing, we're doing lots of things. We're doing interpretability, we're doing process supervision, we're doing red teaming, we're doing evaluation for dangerous capabilities, we're doing work on institutions and governance and tons of stuff.
There's lots of different things. Anyway, what do I think needs to be done? I think that powerful machine learning, powerful AGI is coming in some time.
And if the system is really capable, really intelligent, really powerful, trying to somehow contain it or limit it is probably not a winning strategy because these systems ultimately will be very, very capable. So what you have to do is you have to align it.
You have to get it so it's fundamentally a highly ethical, value-aligned system from the get-go, right? How do you do that? Well, I have a – maybe this is slightly naive, but this is my take on it. How do people do it, right? If you have a really difficult ethical decision in front of you, what do you do, right? Well, you don't just do the first thing that comes to mind, right? Because, you know, there could be a lot of emotions involved and other things, right? It's a difficult problem.
So what you have to do is you have to calm yourself down. You've got to sit down and you've got to think about it.
You've got to think, well, okay, what could I do? I could do this. I could do this.
I could do this. If I do each of these things, what will happen, right? And then you have to think about, so that requires a model of the world.
And then you have to think about ethically, how do I view each of these different actions and the possibilities and what may happen from it,
right? What is the right thing to do? And as you think about all the different possibilities and
your actions and what can follow from them and how it aligns with your values and your ethics,
you can then come to some conclusion of what is really the best choice that you should be making
if you want to be really ethical about this. I think AI systems need to essentially do the
Thank you. of what is really the best choice that you should be making if you want to be really ethical about this.
I think AI systems need to essentially do the same thing. So when you sample from a foundational model at the moment, it's like it's blurting out the first thing.
It's like system one, if you like, from psychology, from Kahneman, right? That's not good enough. And if we do RLHF or what's it called? I can't remember.
Anyway, it's the AI version without the human feedback. RAIF? Is that what it is? Oh gosh, I'm confusing myself.
Anyway, constitutional AI tries to do that sort of thing. You're trying to fix the underlying system one in a sense, right? And that can shift the distribution and that can be very helpful, but it's a very high dimensional distribution and you're sort of poking it in a whole lot of points.
And so it's not likely to be a very robust solution, right? It's like trying to train yourself out of a bad habit. You know, you can sort of do it eventually.
But what you need to do is you need to have a system too. You need the system to not just sample from the model.
You need the system to go, okay, I'm going to reason this through. I'm going to do step-by-step reasoning.
What are the options in front of me? I'm going to use my world model now, and I'm going to use a good world model to understand what's likely to happen from each of these options, and then reason about each of these from an ethical perspective. So you need a system which has a deep understanding of the world, has a good world model, it has a good understanding of people, it has a good understanding of ethics, and it has robust and very reliable reasoning.
And then you set it up in such a way that it applies this reasoning and this understanding of ethics to analyze the different options which are in front of it and then execute on which is the most ethical way forwards. But I think when a lot of people think about the fundamental alignment problem, the worry is not that it's not going to have a world model necessary to understand its actions, or sorry, to understand the effects of its actions.
I guess it's one worry, but not the main worry. The main worry is that the effects it cares about are not the ones we will care about.
And so even if you improve its systems, you're thinking and do better planning, the fundamental problem of we have this really nuanced values about what we want, how do we communicate those values and make sure they're reinforced in the AI? It needs not just a good model of the world, but it needs a really good understanding of ethics. And we need to communicate to the system what ethics and values it should be following.
And how do we do that in a way that we can be confident that a human level or eventually a superhuman level model will preserve those values or learn them in the first place? Well, it should preserve them because if it's making all its decisions based on a good understanding of ethics and values, and it's consistent in doing this, it shouldn't take actions which undermine that. That would be inconsistent.
Right. So then how do we get to the point where it's learned them in the first place? Yeah, that's the challenge.
We need to have systems. The way I think about it is this.
To have a profoundly ethical AI system, it also has to be very, very capable. It needs a really good world model, a really good understanding of ethics, and it needs really good reasoning.
Because if you don't have any of those things, how can you possibly be consistently, profoundly ethical? You can't. So we actually need better reasoning, better understanding of the world and better understanding of ethics in our systems.
Right. So it seems to me the former two would just come along for the ride as these models get more powerful.
Yeah. So that's a nice property because it's actually a capabilities thing.
Right. But then if the third one is a bottleneck or if the third one is a thing that doesn't come along with the AI itself, what is the actual technique to make sure that that happens? The third one, sorry.
The ethical model. What do humans value? Well, we've got a couple of problems.
First of all, we need to decide we should train the system on ethics generally. I mean, there's a lot of lectures and papers and books and all sorts of things.
So it understands human ethics well, right? And we need to make sure it understands human ethics well, right? Because that's important, at least as well as a very good ethicist. And we then need to decide, okay, of this sort of general understanding of ethics, what do we want the system to actually value and what sort of ethics do we want it to apply? Now, that's not a technical problem.
That's a problem for society and ethicists and so on to come up with. Now, I'm not sure there's such a thing as true or correct optimal ethics or something like that, but I'm pretty sure that it's possible to come up with a set of ethics, which is much better than what the so-called doomers worry about in terms of the behavior of these AGI systems.
And then what you do is you engineer the system to actually follow these things. So every time it makes a decision, it does an analysis using a deep understanding of the world and of ethics and very robust and precise reasoning to do an ethical analysis of what it's doing.
And of course, we'd want lots of other things. We'd want people checking these processes of reasoning.
We'd want people, you know, verifying that it's behaving itself in terms of, you know, how it reaches these conclusions. But I still feel like I don't understand how that fundamental problem of making sure it follows that ethic, because presumably, you know, it has Mao's little book, so understands Maoist ethics and understands all these other ethics, you know.
How do we make sure the ethic that we say this is the this is the one we've decided ethicist in society so on today that is the one it ends up following and not the other ones it understands right so you have to specify to the system these are the ethical principles that you should follow and how do we make sure it does that we have to check it as it's doing it we have to assure ourselves that it is consistently following these ethical principles at least i mean there's i'm not sure there's such thing as as optimally but at least as well as a group of human experts are you worried that if you do the default way which is just reinforcing it whenever it seems to be following them you could be training deception as well that is reinforcement has some i aspects to it. I think it's actually more robust to check the process of reasoning and check its understanding of ethics.
So to reassure ourselves that the system has a really good understanding of ethics, it should, grilled for some time to try to really pull apart its understanding and make sure it has a very robust. And then also if it's deployed, we should have people constantly looking for how, you know, the decisions making and the reasoning process that goes into those decisions to try to understand how that is correctly reasoning about these types of things.
Speaking of which, do you at Google DeepMind have some sort of framework for this? This is not so much a Google DeepMind perspective on this. This is my take on how I think we need to do this kind of thing.
There are many different views. And there are different variants on these sorts of ideas ideas as well so then do you personally think there needs to be some sort of framework for as you arrive at certain capabilities these are the concrete safety benchmarks that you must have instated at this point or you should you know pause or slow down or something i i think that's a sensible thing to do it's actually quite hard to do uh and there are some people thinking about i know Anthropics has put out some things like that.
We're thinking about similar things. Actually, you know, putting concrete things down is actually quite a hard thing to do.
So I think it's an important problem, and I certainly encourage people to work on it. Yeah.
So, you know, it's interesting because you have these blog posts that you wrote when you started DeepMind you know back in 2008 where you talk about um the motivation was to accelerate safety on net what do you think the impact of deep mind has been on safety versus capabilities oh interesting I don't know. It's hard to judge, actually.
You know, back in the i've been worried about agi safety for a long time before well before deep mind um but it was it was always really hard to hire people actually particularly in the early days to work on AGI safety. I'm thinking back on 2013 or so, I think we had the first hire and he only agreed to do it part-time because he didn't want to, you know, drop all the capabilities work because, you know, the impact we could have on a career and stuff.
And this was someone who had already previously been publishing in AGI safety. So, yeah, I don know it's hard to hard to know what is the counterfactual if we if we weren't weren't there doing it um i think you know we have been we've been a group that's been um you know talked about this openly i've i've talked about this on many occasions the importance of it um we've been you know hiring people to to work on these topics um you know i know a lot of other people in the area and i've talked to them over many many years i've known dario since 2005 or something or rather you know we've talked on and off about agi safety and so on so i don't know the the impact that deep mind has had you know we i guess we were the first I'd say the first AGI safety and so on.
So I don't know, the impact that DeepMind has had, I guess we were the first, I'd say the first AGI company. And as the first AGI company, we always had an AGI safety group.
We've been publishing papers in this for many years. I think that's lent some credibility to the area when people see, oh, here's a AGI.
I mean, AGI was a, you know, there was a fringe term not that long ago.
And this person's doing AGI safety, not what they're a deep mind.
Oh, OK.
I hope that sort of, you know, creates some space for people.
And where do you think AI progress itself would have been without deep mind?
And this is not just a point that people make about deep mind.
I think this is a general point people make about OpenAI and Anthropic as well, that these people went into
the business to accelerate safety and sort of the net effect might have been to accelerate
capabilities far more. Right, right, right.
I think we have accelerating capabilities, but again,
the counterfactuals are quite difficult. I mean, we didn't do ImageNet, for example,
and ImageNet, I think, was very influential in attracting investment to the field. We did do AlphaGo and that changed some people's minds.
But, you know, the community is a lot bigger than just DeepMind. I mean, we have, well, not so much now, because there are a number of other players with significant resources.
But if you went back more than five years in the future, we were able to do bigger projects with bigger teams and take on more ambitious things than a lot of the smaller academic groups. And so the nature of the type of work we could do was a bit different.
And I think that affected the dynamics in some ways but you know the the community is much much bigger than say DeepMind so maybe we've sped things up a bit but I think a lot of these things would have happened before too long anyway I think I think these often good ideas are kind of in the air and you know as a as a researcher you know when sometimes you publish something or you're about to publish something you see somebody else who's got a very similar idea coming out with some good results um i think often it's the time is right right for things so you know it's i find it very hard to reason about the counterfactuals there speaking of the early early years, it's really interesting that in 2009, you had a blog post where you say my modal expectation of when we get human level AI is 2025, expected value is 2028. And this is before deep learning.
This is when nobody's talking about AI. And it turns out like if you, if the trends continue, this is not an unreasonable prediction.
This was, how did you, I mean, before all these trends came into effect, how did you
have that accurate an estimate? Well, first I'd say it's not before deep learning. Deep learning was getting started around 2008.
Oh, sorry. I meant to say before ImageNet.
Before ImageNet, that was 2012. Yeah.
um so well i first formed those beliefs in about 2001 after reading Ray Kurzweil's The Age of Spiritual Machines. And I came to the conclusion there was two really important points in his book that I came to believe is true.
One is that computational power would grow exponentially for at least a few decades and that the quantity of data in the world would grow exponentially for a few decades. And when you have exponentially increasing quantities of computation and data, then the value of highly scalable algorithms gets higher and higher.
So then there's a lot of incentive to make a more scalable algorithm to harness all this compute and data. And so I thought it would be very likely that we'll start to discover scalable algorithms to do this.
And then there's a positive feedback between all these things, because if your algorithm gets better at harnessing compute and data, then the value of the data in the compute goes up because it can be more effectively used. And so that drives more investment to these areas.
If your compute performance goes up, then the value of the data goes up because you can utilize more data. So there are positive feedback loops between all these things.
So that was the first thing. And then the second thing was just looking at the trends.
If these scalable algorithms were to be discovered, then during the 2020s, it should be possible to start training models on significantly more data than a human would experience in a lifetime. And I figured that that would be a time where big things would start to happen and that would eventually unlock AGI.
So that was my reasoning process.
And I think we're now at that first part. I think we can start training models now where the scale of the data is beyond what a human can experience in a lifetime.
So I think this is the first unlocking step. And so, yeah, I think there's a 50% chance that somebody had 2028.
Now it's just a 50% chance. I mean, I'm sure what's going to happen is going to get to 2029 and someone's going to say, oh, Shane, you were wrong.
It's like, come on, there's 50% chance. So, yeah, I think it's entirely plausible.
Yeah, it's 50% chance it could happen by 2028. But I'm not going to be surprised if it doesn't happen by then maybe you know these you often hit um unexpected problems and in research and sciences and sometimes things take longer than you expect if there was a problem that caused it if we're in 2029 and it hasn't happened yet looking back what would be the most likely reason that would be the kiss i don't know i don't know i at the moment it looks to me like all the problems are likely solvable with a number of years of research that's my current sense and what does the time from here to 2028 look like if the 2028 ends up being the year is it is it just we have trillions of dollars of economic impact in the meantime and would the world gets crazy or what happens i think what you'll see is um the existing models maturing um they'll be less delusional much more factual they'll be more up to date on what's currently going on when they answer questions.
They'll become multimodal much more than they currently are. And this will just make them much more useful.
So I think probably what we'll see more than anything is just loads of great applications for the coming years. I think there can be some misuse cases as well.
I'm sure somebody will come up with, you know, something to do with these models that is quite unhelpful. But my expectation for the coming years is mostly a positive one.
We'll see all kinds of really impressive, really amazing applications for the coming years. Yeah.
And on the safety point, you mentioned these different research directions that are out there and that you are doing internally in deep mind as well interoperability rai f and so on which are you most optimistic about um i don't know i don favorites. It's hard picking favorites.
I know the people
working on all these areas. I think things of the sort of system two flavor.
There's a work we have going on that Jeffrey Irving leads called Deliberative Dialogue, which kind of has the system flavor, where you have this sort of debate takes place about the actions that an agent could take or what's the correct answer to something or something like this. And people then can sort of review these debates and so on.
And they use these sort of AI algorithms to help them judge the correct outcomes and so on.
And so this is sort of meant to be a way
in which to try to scale the alignment
to sort of increasingly powerful systems.
So I think things of that kind of flavor,
I think, have quite a lot of promise in my opinion,
but that's kind of quite a broad category. There are many many different topics within that that's interesting so you mentioned two two areas in which lm doesn't need to improve one is the episodic memory and the other is the system to thinking are those two related or are they two separate are they two separate um drawbacks i think they're fairly separate, but they can be somewhat related.
So you can learn different ways of thinking through problems and actually learn about this rapidly using your episodic memory. So all these different systems and subsystems interact.
So they're never completely separate. But I think conceptually, you can probably think of them as quite separate things.
I think delusions and factuality is another area that's going to be quite important, and particularly important in lots of applications. If you want a model that writes creative poetry, then that's fine, because you want to be able to be very free to suggest all kinds of possibilities and so on.
You're not really constrained by a specific reality. Whereas if you want something that's in a particular application, normally you have to be quite concrete about what's currently going on and what is true and what is not true and so on.
And models are a little bit sort of freewheeling when it comes to truth and creativity at the moment. And that, I think, limits their applications in many ways..
So final question is this, you've been in this field for over a decade, much longer than many others. And you've seen these different landmarks, ImageNet, Transformers.
What do you think the next landmark will look like? I think the next landmark that people will think back to and remember is going much more fully multimodal, I think. Because I think that will open out the sort of understanding that you see in language models into a much larger space of possibilities.
And when people think back, they'll think about, oh, those old-fashioned models they they just did like chat they just did text you know it was just felt like a very narrow thing whereas now they you know they understand when you talk to them and they they understand images and pictures and video and and you can show them things or things like that and they'll they will have much more understanding of what's going on and it will feel like the system's kind of opened up into the world
in a much more powerful way.
Do you mind if I ask you a follow-up on that?
So ChadGPT just released their multimodal feature,
and then you, in DeepMind, you had the Gato paper
where you have this one model, you can images,
even actions, video games, whatever you can throw in there.
And so far, it doesn't seem to have been, it hasn't percolated as much as even like chat GPT initially from GPT-3 or something. What explains that? Is it just that people haven't learned to use multimodality? They're not powerful enough yet? I think it's early days.
I think there's, you can see promise here, understanding images and things more and more. But I think it's, yeah, it's early days in this transition is when you start really digesting a lot of video and other things like that that the systems will start having a much more grounded understanding of the world and all kinds of other aspects and then when that works well that will open up naturally lots and lots of new new applications and all sorts of new possibilities because you're not confined to text chat anymore.
The new avenues of training data as well, right? Yeah, new training data and all kinds of different applications that aren't just purely textual anymore. And what are those applications? Well, probably a lot of them we can't even imagine at the moment because there are just so many possibilities once you can start dealing with all sorts of different modalities in a consistent way.
Awesome. Shane, I think that's an excellent place to leave it off.
All right.
Thank you so much for coming on the podcast.
Thank you.
Hey, everybody. I hope you enjoyed that episode.
As always, the most helpful thing you can do is to share the podcast.
Send it to people you think might enjoy it.
Put it in Twitter, your group chats, etc.
It just splits the world.
Appreciate you listening. I'll see you next time.
Cheers.