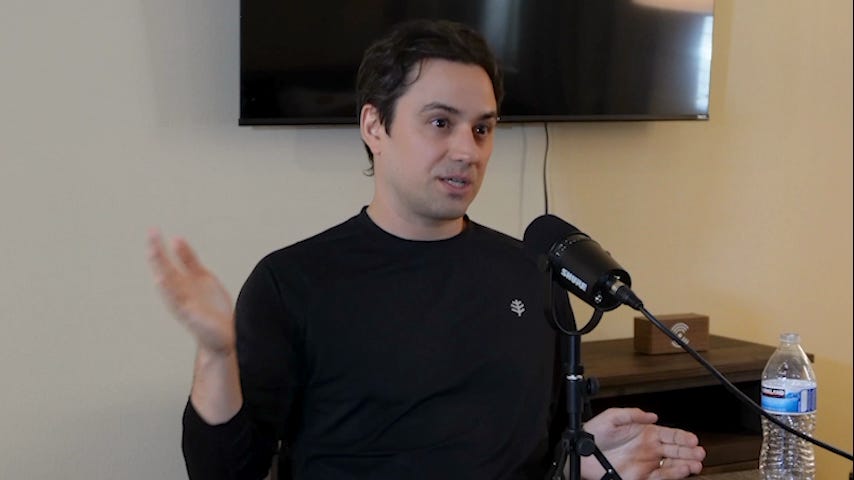
Carl Shulman (Pt 1) - Intelligence Explosion, Primate Evolution, Robot Doublings, & Alignment
In terms of the depth and range of topics, this episode is the best I’ve done.
No part of my worldview is the same after talking with Carl Shulman. He's the most interesting intellectual you've never heard of.
We ended up talking for 8 hours, so I'm splitting this episode into 2 parts.
This part is about Carl’s model of an intelligence explosion, which integrates everything from:
* how fast algorithmic progress & hardware improvements in AI are happening,
* what primate evolution suggests about the scaling hypothesis,
* how soon before AIs could do large parts of AI research themselves, and whether there would be faster and faster doublings of AI researchers,
* how quickly robots produced from existing factories could take over the economy.
We also discuss the odds of a takeover based on whether the AI is aligned before the intelligence explosion happens, and Carl explains why he’s more optimistic than Eliezer.
The next part, which I’ll release next week, is about all the specific mechanisms of an AI takeover, plus a whole bunch of other galaxy brain stuff.
Maybe 3 people in the world have thought as rigorously as Carl about so many interesting topics. This was a huge pleasure.
Watch on YouTube. Listen on Apple Podcasts, Spotify, or any other podcast platform. Read the full transcript here. Follow me on Twitter for updates on future episodes.
Timestamps
(00:00:00) - Intro
(00:01:32) - Intelligence Explosion
(00:18:03) - Can AIs do AI research?
(00:39:00) - Primate evolution
(01:03:30) - Forecasting AI progress
(01:34:20) - After human-level AGI
(02:08:39) - AI takeover scenarios
Get full access to Dwarkesh Podcast at www.dwarkesh.com/subscribe
Listen and Follow Along
Full Transcript
Human-level AI is deep, deep into an intelligence explosion. Things like inventing the transformer or discovering chinchilla scaling and doing your training runs more optimally or creating flash attention.
That set of inputs probably would yield the kind of AI capabilities needed for intelligence explosion. You have a race between, on the one hand, the project of getting strong interpretability and shaping motivations.
And on the other hand, these AIs, in ways that you don't perceive, make the AI take over happen.
We spend more compute by having a larger brain than other animals.
And then we have a longer childhood.
It's analogous to having a bigger model and having more training time with it.
It seemed very implausible that we couldn't do better than completely brute force evolution. How quickly are we running through those orders of magnitude? Hey everybody, just wanted to give you a heads up.
So I ended up talking to Carl for like seven or eight hours. So we ended up splitting this episode into two parts.
I don't want to put all of that on you at once. In this part, we get deep into Carl's model of an intelligence explosion and what that implies for alignment.
The next part, which we'll release next week, is all about the specific mechanisms of an AI takeover. In terms of the depth and the range of interesting topics, this set of episodes is the best I've ever done.
So I hope you all enjoy. Here's Carl.
Okay, today I have the pleasure of speaking with Carl Schulman. Many of my former guests, and this is not an exaggeration, many of my former guests have told me that a lot of their biggest ideas, perhaps most of their biggest ideas, have come directly from Carl, especially when it has to do with the intelligence explosion and its impacts.
And so I decided to go directly to the source. And we have Carl today on the podcast.
Carl keeps a super low profile, but he is one of the most interesting intellectuals I've ever encountered. And this is actually his second podcast ever.
So we're going to get to get deep into the heart of many of the most important ideas that are circulating right
now directly from the source. So and by the way, so Carl is also an advisor to the Open Philanthropy
Project, which is one of the biggest funders on causes having to do with AI and its risks,
not to mention global health and well being. And he is a research associate at the Future
of Humanity Institute at Oxford. So Carl, it's a huge pleasure to have you on the podcast.
Thanks for coming. Thank you, Drakash.
I've enjoyed seeing some of your episodes recently, and I'm glad to be on the show. Excellent.
Let's talk about AI. Before we get into the details, give me the sort of big picture explanation of the feedback loops and just the general dynamics that would start when you have something that is approaching human level intelligence.
Yeah. So I think the way to think about it is we have a process now where humans are developing new computer chips, new software, running larger training runs.
And it takes a lot of work to keep Moore's Law chugging. Well, it was.
It's slowing down now. And it takes a lot of work to develop things like transformers, to develop a lot of the improvements to AI and neural networks that are advancing things.
And the core method that I think I want to highlight on this podcast, and I think is underappreciated, is the idea of input-output curves. So we can look at the increasing difficulty of improving chips.
And so sure,
each time you double the performance of computers, it's harder. And as we approach physical limits, eventually it becomes impossible.
But how much harder? So there's a paper called Ideas Getting Harder to Find that was published a few years ago. Something like 10 years ago at Miri, we did, I mean, I did an early version of this analysis using mainly data from Intel and like the large semiconductor fabricators.
Anyway, and so in this paper, they cover a period where the productivity of computing went up a million
fold. So you could get a million times the computing operations per second per dollar.
Big change. But it got harder.
So the amount of investment, the labor force required to make
those continuing advancements went up and up and up.
Indeed, it went up 18-fold over that period. So some take this to say, oh, diminishing returns.
Things are just getting harder and harder, and so that will be the end of progress eventually. However, in a world where AI is doing the work, that doubling of computing performance translates pretty directly to a doubling or better of the effective labor supply.
That is, if when we had that million-fold compute increase, we used it to run artificial intelligences who would replace human scientists and engineers, then the 18x increase in the labor demands of the industry would be trivial. We're getting more than one doubling of the effective labor supply that we need for each doubling of the labor requirement.
And in that data set, it's like over four.
So we double compute.
Okay, now we need somewhat more researchers,
but a lot less than twice as many.
And so, okay, we use up some of those doublings of compute on the increasing difficulty of further research, but most of them are left to expedite the process. So if you double your labor force, that's enough to get several doublings of compute.
You use up one of them on meeting the increased demands from diminishing returns. The others can be can be used to accelerate the process.
So your first doubling takes however many months. Your next doubling can take a smaller fraction of that.
The next doubling less and so on. At least insofar as this, the outputs you're generating, compute for AI in this story, are able to serve the function of the necessary inputs.
If there are other inputs that you need, eventually those become a bottleneck and you wind up more restricted on this. Got it.
Okay. So yeah, I think the Bloom paper had that there was a 35% increase in, was it transitor density or cost per flop? And there was a 7% increase per year in the number of researchers required to sustain that pace.
Something in the vicinity, yeah, it's like four to five doublings of compute per doubling of labor inputs. I guess there's a lot of questions you can delve into in terms of whether you would expect a similar scale with AI and whether it makes sense to think of AIs as a population of researchers that keeps growing with compute itself.
Actually, let's go there. So can you explain the intuition that compute is a good proxy for the number of AI researchers, so to speak? So far, I've talked about hardware as an initial example because we had good data about a past period.
You can also make improvements on the software side. And when we think about an intelligence explosion, that can include AI's doing work on making hardware better, making better software, making more hardware.
But the basic idea for the hardware is especially simple in that if you have a worker, an AI worker that can substitute for a human, if you have twice as many computers, you can run two separate instances of them. And then they can do two different jobs, manage two different machines, work on two different design problems.
Now, you can get more gains than just what you would get by having two instances. We get improvements from using some of our compute, not just to run more instances of the existing AI, but to train larger AIs.
So there's hardware technology, how much you can get per dollar you spend on hardware. And there's software technology.
And the software can be copied freely. So if you've got the software, it doesn't necessarily make that much to say that, oh, we've got 100 Microsoft Windows.
You can make as many copies as you need for whatever Microsoft will charge you.
But for hardware, it's different. It matters how much we actually spend on the hardware at a given price.
And if we look at the changes that have been driving AI recently, that is the thing that is really off trend. We are spending tremendously more money on computer hardware for training big AI models.
Yeah. Okay.
So there's the investment in hardware. There's the hardware technology itself.
And there's the software progress itself. The AI is getting better because we're spending more money on it, because our hardware itself is getting better over time.
because we're like developing better models or better adjustments to those models where is the loop here the work involved in designing new hardware and software is being done by people now they use computer tools to assist them but like computer time is not like the primary cost for NVIDIA designing chips, for TSMC producing them, for ASML making lithography equipment to serve the TSMC fabs. And even in AI software research, that has become quite compute-intensive.
But I think we're still in the range where at a place like DeepMind, salaries were still larger than compute for the experiments, although tremendously, tremendously more of the expenditures were on compute relative to salaries than in the past. If you take all of the work that's being done by those humans, there's like low tens of thousands of people working at NVIDIA designing GPUs specialized for AI.
I think there's more like 70,000 people at TSMC, which is the leading producer of cutting-edge chips. There's a lot of additional people at companies like ASML that supply them with the tools they need.
And then a company like DeepMind, I think, from their public filings, they recently had 1,000 people. OpenAI, I think, is a few hundred people.
Anthropic is less. If you add up things like Facebook AI research,
Google Brain, other R&D,
you've got thousands or tens of thousands of people
who are working on AI research.
We'd want to zoom in on those who are developing new methods
rather than narrow applications.
So inventing the transformer definitely counts.
Optimizing for some particular businesses' data set cleaning, probably not. But so those people are doing this work.
They're driving quite a lot of progress. What we observe and the growth of people relative to the growth of those capabilities is that pretty consistently, the capabilities are doubling on a shorter timescale than the people required to do them are doubling.
And so there's work. We talked about hardware and how historically, it was pretty dramatic, like four or five doublings of compute efficiency per doubling of human inputs.
I think that's a bit lower now as we get towards the end of Moore's Law. Although interestingly, not as much lower as you might think because the growth of inputs has also slowed recently.
On the software side, there's some work by Temei Besaroglu, and I think collaborators. It may have been his thesis.
It's called, Are Models Getting Harder to Find? And so it's applying the same sort of analysis as our ideas getting harder to find. And you can look at growth rates of papers from citations, employment at these companies, and it seems like the doubling time of these workers driving the software advances is like several years, or at least a couple of years, whereas the doubling of effective compute from algorithmic progress is faster.
So there's a group called Epoch. They've received grants from open philanthropy, and they do work collecting data sets that are relevant to forecasting AI progress.
And so their headline results for what's the rate of progress in hardware and software and just like growth in budgets are as follows. So for hardware, they're looking at like a doubling of hardware efficiency that's like two years.
It's possible it's a bit better than that when you take into account certain specializations for AI workloads. For the growth of budgets, they find a doubling time that's like something like six months in recent years, which is pretty tremendous relative to the historical rates.
We should maybe get into that later.
And then on the algorithmic progress side, mainly using ImageNet type data sets right
now, they find a double in time that's less than one year.
And so you combine all of these things and the growth of effective compute for training big AIs, it's pretty drastic.
I think I saw an estimate that GPT-4 costs like $50 million around that range to train.
Now suppose that like AGI takes 1,000x that if you were to scale up GPT-4.
It might not be that.
Just for the sake of example.
So part of that will come from companies just spending a lot more to train the models and that just greater investment part of that will come from them having better models so that what would have taken a 10x increase in the model to get naively you can do with having a better model that you only need to scale up you you get the same effect of increasing it by 10x just from having a better model and so yeah you can spend more money on it to turn a bigger model you can just have a better model or you can have chips that are cheaper to train so you get more compute for the same dollars and okay so those are the three you're describing the ways in which the quote-unquote effective compute would increase from the looking at it right now it looks like yeah you might get two or three doublings of effective compute for this thing that we're calling software progress um which is which people get by asking well how much less compute can you use now to achieve the same benchmark as you achieved before there are reasons to not fully identify this with like software progress as you might naively think of it because some of it can be enabled by the others. So like when you have a lot of compute, you can do more experiments and find algorithms that work better.
Sometimes the additional compute, you can get higher efficiency by running a bigger model we were talking about earlier. And so that means you're getting more for each GPU that you have because you made this larger expenditure.
And that can look like a software improvement because this model, it's not a hardware improvement directly because it's doing more with the same hardware. But you wouldn't have been able to achieve it without having a ton of GPUs to do the big training run.
The feedback loop itself involves the AI that is the result of this greater effective compute helping you train better AI, right? Or use less effective compute in the future to train better AI. It can help on the hardware design.
So like NVIDIA is a fabulous chip design company. They don't make their own chips.
They send files of instructions to TSMC, which then fabricates the chips in their own facilities. And so the work of those 10,000 plus people, if you could automate that and have the equivalent of a million people doing that work, then I think you would pretty quickly get the kind of improvements that can be achieved with the existing nodes that TSMC is operating on.
You could get a lot of those chip design gains, basically like doing the job of improving chip design that those people are working on now, but get it done faster. So that's one thing.
I think that's less important for the intelligence explosion. The reason being that when you make an improvement to chip design, it only applies to the chips you make after that.
If you make an improvement in AI software, it has the potential to be immediately applied to all of the GPUs that you already have. Yeah.
And so the thing that I think is most disruptive and most important has the leading edge of the change from AI automation of the inputs to AI is on the software side. Well, at what point would it get to the point where the AIs are helping develop better software or better models for future AIs? Some people claim today, for example, that programmers at OpenAI are using Copilot to write programs now.
So in some sense, you're already having that sort of feedback loop. I'm a little skeptical of that as a mechanism.
At what point would it be the case that the AI is contributing significantly in the sense that it would almost be the equivalent of having additional researchers to AI progress in software? The quantitative magnitude of the help is absolutely central. So there are plenty of companies that make some product that very slightly boost productivity.
So when Xerox makes fax machines, it maybe increases people's productivity in office work by 0.1% or something.
You're not going to have explosive growth out of that because, okay, now 0.1% more effective R&D at Xerox than any customers buying the machines. Not that important.
So I think the thing to look for is when is it the case that the contributions from AI are starting to become as large or larger as the contributions from humans. So like when this is boosting their effective productivity by 50 or a hundred percent, and you, like, if you then go from eight months doubling time, say for effective compute from software innovations, things like inventing the transformer or discovering chinchilla scaling and doing your training runs more optimally or creating flash attention.
Yeah. If you move that from say eight months to four months, and then the next time you apply that, it significantly increases the boost you're getting from the AI.
So now maybe instead of giving a 50% or 100% productivity boost, that's more like a 200%. And so it doesn't have to have been able to automate everything involved in the process of AI research.
It can be, it's automated a bunch of things. And then those are being done in extreme profusion because any, I think, a thing that AI can do, you have it done much more often because it's so cheap.
And so it's not a threshold of this is human level AI. It can do everything a human can do with no weaknesses in any area.
It's that even with its weaknesses. It's able to bump up the performance so that instead of getting like the results we would have with the 10,000 people working on finding these innovations, we get the results that we would have if we had twice as many of those people with the same kind of skill distribution.
And so it's like a demanding challenge. It's like you need quite a lot of capability for that.
But it's also important that it's significantly less than this is a system where there's no way you can point at it and say in any respect, it is weaker than a human. A system that was just as good as a human in every respect, but also had all of the advantages of an AI, that is just way beyond this point.
If you consider that the output of our existing fabs can make tens of millions of advanced GPUs per year. Those GPUs, if they were running AI software that was as efficient as humans, is sample efficient, it doesn't have any major weaknesses.
So they can work four times is long, you know, the 168 hour work week, they can have much more education than any human. So it's, you know, human, you know, you got a PhD, you know, it's like, wow, it's like 20 years of education, maybe longer if they take a slow route on the PhD.
it's just normal for us to train large models by eat the internet, eat all the published books ever, read everything on GitHub and get good at predicting it. So the level of education vastly beyond any human, the degree to which the models are focused on task is higher than all but the most motivated humans when they're really, really gunning for it.
So you combine the things, tens of millions of GPUs, each GPU is doing the work of the very best humans in the world. And the most capable humans in the world can command salaries that are a lot higher than the average, in particular in a field like STEM or nearly AI.
There's no human in the world who has a thousand years of experience with TensorFlow, let alone the new AI technologies that were invented the year before. But if they were around, yeah, they'd be paid millions of dollars a year.
And so when you consider this, okay, tens of millions of GPUs, each is doing the work of maybe 40, maybe more of these kind of existing workers. This is like going from a workforce of tens of thousands to hundreds of millions.
You immediately make all kinds of discoveries then.
You immediately develop all sorts of tremendous technologies.
So human level AI is deep, deep into an intelligence explosion.
Intelligence explosion has to start with something weaker than that. Yeah.
What is the thing it starts with and how close are we to that? Because if you think of a researcher at OpenAI or something, you know, these are, to be a researcher is not just completing the hello world prompt that Copilot does, right? It's like you got to choose a new idea. You had to figure out the right way to approach it.
You perhaps have to manage the people who are also working with you on that problem you know it's like it's it's incredibly complicated skill portfolio of skills rather than a single skill so yeah what is the point i wish that feedback loop starts where you can even you're not just doing the 0.5 increase in productivity that a sort of AI tool might do, but is actually
the equivalent of a researcher or close to it. Like what is that point? So I think maybe a way look at it is to give some illustrative examples of like the kinds of capabilities that you might see.
And so because these systems have to be a lot weaker than this sort of human level things, what we'll have is intense application of the ways in which AIs have advantages, partly offsetting their weaknesses. And so AIs are cheap.
We can call a lot of them to do many small problems. And so you'll have situations where you have dumber AIs that are deployed thousands of times to equal, say, one human worker.
And they'll be doing things like these voting algorithms where you, with an LLM, you generate a bunch of different responses and take a majority vote among them that improves performance sum. You'll have things like the AlphaGo kind of approach where you use the neural net to do search and you go deeper with the search by plowing in more compute, which helps to offset the inefficiency and weaknesses of the model on its own.
You'll do things that would just be totally impractical for humans because of the sheer number of steps. And so an example of that would be designing synthetic training data.
So humans do not learn by just going into the library and opening books at random pages. It's actually much, much more efficient to have things like schools and classes
where they teach you things in an order that makes sense,
that's focusing on the skills that are more valuable to learn.
They give you tests and exams.
They're designed to try and elicit the skill
they're actually trying to teach.
And right now we don't bother with that
because we can hoover up more data from the internet. We're getting towards the end of that.
But yeah, as the AIs get more sophisticated, they'll be better able to tell what is a useful kind of skill to practice and to generate that. And we've done that in other areas.
So AlphaGo, the original version of AlphaGo was booted up with data from human GoPlay and then improved with reinforcement learning and Monte Carlo TreeSearch. But then AlphaZero, with a somewhat more sophisticated model, benefited from some other improvements but was able to go from scratch.
And it generated its own data through self-play, so getting data of a higher quality than the human data, because there are no human players that good available in the data set, and also a curriculum so that at any given point, it was playing games against an opponent of equal skill itself. And so it was always in an area when it was easy to learn.
If you're just always losing no matter what you do or always winning no matter what you do, it's hard to distinguish which things are better and which are worse. And when we have somewhat more sophisticated AIs that can generate training data and tasks for themselves, for example, if the AI can generate a lot of unit tests and then can try and produce programs that pass those unit tests, then the interpreter is providing a training signal.
And the AI can get good at figuring out what's the kind of programming problem that is hard for AIs right now that will develop more of the skills that I need.
Thank you. The AI can get good at figuring out what's the kind of programming problem that is hard for AIs right now that will develop more of the skills that I need and then do them.
And now you're not going to have employees at OpenAI write a billion programming problems. That's just not going to happen.
But you are going to have AIs given the task of producing those enormous number of programming challenges. In LLMs themselves, there's a paper out of Anthropa called Constitution AI or Constitution RL, where they basically have the program just talk to itself and say, is this response helpful? If not, how can I make this more helpful? And the response is improved.
And then you train the model on the more helpful responses that it generates by talking to itself so that it generates natively.
And you could imagine, you know, more sophisticated way to do that or better ways to do that.
OK, so but then the question is, listen, you know, GPT-4 already costs like 50 million or 100 million or whatever it was. Even if we have greater effect to compute from hardware increases and better models, it's hard to imagine how we could sustain like four or five more orders of magnitude, greater size, effective size than GPT-4, unless we're dumping in like trillions of dollars, like the entire economies of big countries into training the next version.
So the question is, do we get something that can significantly help with AI progress before we run out of the sheer money and scale and compute that would require to train it? Do you have a take on that? Well, first, I'd say remember that there are these three contributing trends. So the new H100s are significantly better than the A100s.
And a lot of companies are actually waiting for their deliveries of H100s to do even bigger training runs, along with the work of hooking them up into clusters and engineering the thing. Yeah.
So all of those factors are contributing. And of course, mathematically, yeah, if you do four orders of magnitude more than 50 or 100 million, then you're getting to trillion dollar territory.
I think the way to look at it is at each step along the way, does it look like it makes sense to do the next step? From where we are right now, seeing the results with GPT-4 and ChatGPT, companies like Google and Microsoft and whatnot are pretty convinced that this is very valuable. You have like talk at Google and Microsoft with Bing that, well, it's like billion dollar matter to change market share in search by a percentage point.
And so that can fund a lot. And on the far end, on the extreme, if you automate human labor, we have a hundred trillion dollar economy.
Most of that economy is paid out in wages. So like between 50 and 70 trillion dollars per year.
If you create AGI, it's going to automate all of that and keep increasing beyond that. So the value of the completed project is very much worth throwing our whole economy into it.
if you're going to get the good version, not the catastrophic destruction of the human race or, you know, some, some other disastrous outcome. And in between it's a question of, well, did the next step, how risky and uncertain is it? And how much growth in the revenue you can generate with it do you get? And so for moving up to a billion dollars, I think that's absolutely going to happen.
These large tech companies have R&D budgets, tens of billions of dollars. And when you think about it in the relevant sense, all the employees at Microsoft who are doing software engineering, that's like contributing to creating software objects.
It's not, it's not weird to spend tens of billions of dollars on a product that would do so much. And I think it's, it's becoming more clear that there is sort of market opportunity to fund the thing going up to a $100 billion, that's like, okay, the existing R&D budgets spread over multiple years.
But if you keep seeing that when you scale up the model, it substantially improves the performance. It opens up new applications.
You're not just improving your search, but maybe it makes self-driving cars work. You replace bulk software engineering jobs, or if not replace them, amplify productivity.
In this kind of dynamic, you actually probably want to employ all the software engineers you can get as long as they're able to make any contribution because the returns of improving stuff in AI itself get so high. But yeah, so I think that can go up to a hundred billion.
And at a hundred billion, you're using like a significant fraction of our existing fab capacity. Like right now, the revenue of NVIDIA is like 25 billion.
The revenue of TSMC, I believe it's like it's50 billion. I checked in 2021, NVIDIA was maybe 7.5%, less than 10% of TSMC revenue.
So there's a lot of room. And most of that was not AI chips.
They have a large gaming segment. There are data center GPUs that are used for video and the like.
So there's room for more than an order of magnitude increase by redirecting existing fabs to produce more AI chips and just actually using the AI chips that these companies have in their cloud for the big training runs. And so I think that's enough to go to the $10 billion and then combine with stuff like the H100 to go up to the $100 billion.
Just to emphasize for the audience the initial point about revenue made, if it cost OpenAI $100 million to train GPT-4 and it generates $500 million in revenue, you pay back your expenses with $100 million, you have $400 million for your next training run, then you train your GPT-4.5. You get, let's say, $4 billion out of revenue out of that.
That's where the feedback group of revenue comes from, where you're automating tasks and therefore you're making money. You can use that money to automate more tasks.
On the ability to redirect the fab production towards ai chips so then the tldr on you want 100 billion dollars worth of compute i mean fabs take what like a decade or so to build um so given the ones we have now and the ones that are going to come online in the next decade is there enough to sustain a $100 billion of GPU compute if you wanted to spend that on a training run? Yes, you definitely make the $100 billion one. As you go up to a trillion dollar run and larger, it's going to involve more fab construction.
And yeah, fabs can take a long time to build. On the other hand, if in fact you're getting very high revenue from the AI systems and you're actually bottlenecked on the construction of these fabs, then their price could skyrocket.
And that would lead to measures we've never seen before to expand and accelerate fab production. Like if you, so at the limit, how you're getting models that approach human-like capability.
Imagine things that are getting close to brain-like efficiencies plus AI advantages. We were talking before about, well, a GPU that is supporting an AI.
Really, it's a cluster of GPUs supporting AIs
that do things in parallel, data parallelism.
But if that can work four times as much as a human,
a highly skilled, motivated, focused human
with levels of education that have never been seen
in the human population.
And so if a typical software engineer can earn hundreds of thousands of dollars, the world's best software engineers can earn millions of dollars today, and maybe more in a world where there's so much demand for AI. And then times four for working all the time.
Well, I mean, if you have, if you generate like close to $10 million a year
out of the future version of H100, and it costs tens of thousands of dollars with a huge profit margin now, and profit margin could be reduced with like large production. That is a big difference that that chip pays for itself almost instantly.
And so you could support paying 10 times as much to have these fabs constructed more rapidly. You could have, if AI is starting to be able to contribute, you could have AI contributing more of the skilled technical work that makes it hard for, say, NVIDIA to suddenly find thousands upon thousands of top quality engineering hires if AI can provide that.
Now, if AI hasn't reached that level of performance, then this is how you can have things stall out. And like a world where AI progress stalls out is one where you go to the hundred billion and then over succeeding years, trillion dollar things, software progress turns out to stall.
You lose the gains that you are getting from moving researchers from other fields. Lots of physicists and people from other areas of computer science have been going to AI.
But you sort of tap out those resources as AI becomes a larger proportion of the research field. And like, okay, you've put in all of these inputs, but they just haven't yielded AGI yet.
I think that set of inputs probably would yield the kind of AI capabilities needed for intelligence explosion. But if it doesn't, after we've exhausted this current scale of increasing the share of our economy that is trying to make AI, if that's not enough, then after that, you have to wait for the slow grind of things like general economic growth, population growth, and such.
And so things slow. And that results in my credences and this kind of advanced AI happening to be relatively concentrated, like over the next 10 years compared to the rest of the century, because we just can't we can't keep going with this rapid redirection of resources into AI.
That's that's a one time thing. If the current scale up works, it's going to happen.
We're going to get to H.I. really fast, like within the next 10 years or something.
If the current scale up doesn't work, all we're left with is just like our economy growing like 2% a year. So we have like 2% a year more resources to spend on AI.
And at that scale, you're talking about decades before you can just your sheer brute force, you can, you know, train that $10 trillion model or something. Let's talk about why you have your thesis that the current scale-up would work.
What is the evidence from AI itself or maybe from primate evolution and the evolution of other animals? Just give me the whole confluence of reasons that make you think. I think maybe the best way to look at that might be to consider when I first became interested in this area, so in the 2000s, which was before the deep learning revolution, how would I think about timelines?
How did I think about timelines?
And then how have I updated based on what has been happening with deep learning?
And so back then, I would have said, we know the brain is a physical object and an information processing device. It works.
It's possible. And not only is it possible, it was created by evolution on Earth.
And so that gives us something of an upper bound in that this kind of brute force was sufficient. There are some complexities with like, well, what if it was a freak accident that didn't happen on all of the other planets and that added some value? I have a paper with Nick Bostrom on this.
I think basically that's not that important an issue. There's convergent evolution like octopi are also quite sophisticated.
If a special event was at the level of forming cells at all or forming brains at all, we get to skip that because we're choosing to build computers and we already exist. We have that advantage.
So evolution gives something of an upper bound really intensive massive brute force search
and things like evolutionary algorithms can produce intelligence doesn't the fact that octopi and i guess other mammals they got to the point of being like pretty intelligent but not human level intelligent is that some evidence that there's a heart step between a cephalopod and a human yeah Yeah, so that would be a place to look.
It doesn't seem particularly compelling. One source of evidence on that is work by Herculano Hutzl.
I hope I haven't mispronounced her name, but she's a neuroscientist who has dissolved the brains of many creatures. And by counting the nuclei, she's able to determine how many neurons are present in different species and find a lot of interesting trends in scaling laws.
And she has a paper discussing the human brain has a scaled up primate brain and across like a wide variety of animals and mammals in particular. There are certain characteristic changes in the relative number of neurons, size of different brain regions, how things scale up.
There's a lot of, yeah, there's a lot of structural similarity there. And you can explain a lot of what is different about us with a pretty brute force story, which is that you expend resources on having a bigger brain, keeping it in good order, giving it time to learn.
So we have an unusually long childhood, unusually long neonates period. We spend more compute by having a larger brain than other animals, more than three times as large as chimpanzees.
And then we have a longer childhood than chimpanzees and much more than many other creatures. So we're spending more compute in a way that's analogous to having a bigger model and having more training time with it.
And given that we see with our AI models, this sort of like large, consistent benefits from increasing compute spent in those ways and with qualitatively new capabilities showing up over and over again, particularly in areas that sort of AI skeptics call out. In my experience, like over the last 15 years, the things that people call out is like, ah, but the AI can't do that.
And it's because of a fundamental limitation. I've gone through a lot of them.
You know, there were Winograd schemas, catastrophic forgetting, quite a number. And yeah, they have repeatedly gone away, uh, through scaling.
And so there's a, there's a picture that we're, we're seeing supported from
biology and from our experience with AI, where you can explain like, yeah, in general, there are
trade-offs where the extra fitness you get from a brain is not worth it. And so creatures wind up mostly with small brains because they can save that biological energy and that time to reproduce for digestion and so on.
humans, we actually seem to have wound up in a niche within self-reinforcing,
where we greatly increased the returns to having large brains.
And language and technology are the sort of obvious candidates. It's when you have humans around you who know a lot of things and they can teach you.
And compared to almost any other species, we have vastly more instruction from parents and the society of the young.
And then you're getting way more from your brain. Because you can get per minute, you can learn a lot more useful skills, and then you can provide the energy you need to feed that brain by hunting and gathering, by having fire that makes digestion easier.
and basically how this process goes on, it's increasing the marginal increase in reproductive fitness you get from allocating more resources along a bunch of dimensions towards cognitive ability. And so that's bigger brains, longer childhood, having our attention be more on learning.
So humans play a lot and we keep playing as adults, which is a very weird thing compared to other animals. We're more motivated to copy other humans around us than like even than the other primates.
And so these are sort of motivational changes that keep us using more of our attention and effort on learning, which pays up more when you have a bigger brain and a longer lifespan in which to learn. Many creatures are subject to lots of predation or disease.
And so if you try, you know, you're a mayfly or a mouse, if you try and invest in like a giant brain and a very long childhood, you're quite likely to be killed by some predator or some disease before you're able to actually use it. And so that means you actually have exponentially increasing costs in a given niche.
So if I have a 50% chance of dying every few months of a little mammal or a little lizard or something, that means the cost of going from three months to 30 months of learning and childhood development is not 10 times the loss. Now it's two to the negative 10.
So a factor of 1,024 reduction in the benefit I get from what I ultimately learn. Because 99.9% of the animals will have been killed before that point.
We're in a niche where we're like a large, long-lived animal with language and technology, so where we can learn a lot from our groups. And that means it pays off to really just expand our investment on these multiple fronts in intelligence.
That's so interesting. Just for the audience, the calculation about like two to the whatever months is just like you have a half chance of dying this month a half chance of dying next month um you multiply those together okay there's other species though that do live in flocks or as um packs where you could imagine i mean they do have like a smaller version of the development of cubs into that are like play with each other.
Why isn't this a hill on which they could have climbed to human level intelligence themselves? If it's something like language or technology, humans were getting smarter before we got language. I mean, obviously, we had to get smarter to get language, right? We can just get language without without becoming smarter so yeah where did it seems like there should be other species that should have beginnings of this sort of cognitive revolution especially given how valuable it is given listen we've dominated the world you would think there would be selective pressure for it evolution doesn't have foresight yeah the thing in this generation that gets more surviving offspring and grandchildren, that's the thing that becomes more common.
Evolution doesn't look ahead and think, oh, in a million years, you'll have a lot of descendants. It's what survives and reproduces now.
And so, in fact, there are correlations where social animals do, on average, have larger brains. And part of that is probably that the additional social applications of brains, like keeping track of which of your group members have helped you before so that you can reciprocate.
You scratch my back, I'll scratch yours. Remembering who's dangerous within the group, that sort of thing.
It's an additional application of intelligence. And so there's some correlation there.
But what it seems like is that, yeah, in most of these cases, it's enough to invest more, but not invest to the point where a mind can easily develop language and technology and pass it on.
And so you see bits of tool use in some other primates who have an advantage compared to, say, the whales.
They have quite large brains, partly because they are so large themselves and they have some other things.
But they don't have hands, which means that reduces a bunch of ways in which brains can pay off and investments in the functioning of that brain. But yeah, so primates will use sticks to extract termites.
Capuchin monkeys will open clams by smashing them with a rock. So there's bits of tool use.
But what they don't have is the ability to sustain culture. A particular primate will maybe discover one of these tactics and maybe it'll be copied by their immediate group.
But they're not holding onto it that well. They're like, well, when they see the other animal do it, they can copy it in that situation.
They don't actively teach each other. Their population locally is quite small.
So it's easy to forget things, easy to lose information. And in fact, they remain technologically stagnant for hundreds of thousands of years.
And we can actually look at some human situations. So there's an old paper, I believe by the economist Michael Kramer, talks about technological growth in the different continents for human societies.
And so you have Eurasia is the largest integrated connected area. Africa is partly connected to it, but the Sahara Desert restricts the flow of information and technology and such.
And then you had the Americas, which were after the colonization from the land bridge, were largely separated and are smaller than Eurasia, then Australia. And then you had like smaller island situations like Tasmania.
And so technological progress seemed to have been faster, the larger the connected group of people. and in the smallest groups so like in Tasmania
you had a relatively small population and they actually lost technology. So things like they lost some like fishing techniques.
And if you have a small population and you have some limited number of people who know a skill and they happen to die or it happened. There's
some change in circumstances that causes people not to practice or pass on that thing, and then you lose it. And if you have few people, you're doing less innovation.
The rate at which you lose technologies to some kind of local disturbance and the rate at which you create new technologies can wind up in balance.
And the great change of hominids and humanity is that we wound up in this situation where we were accumulating faster than we were losing. And as we accumulated, those technologies allowed us to expand our population.
They created additional demand for intelligence so that our brains became three times as large as that chimpanzees chimpanzees yeah and our ancestors who had a similar um brain size okay and then the crucial point uh i guess in relevance to ai is that the selective pressures against intelligence in other animals are not uh acting against these neural networks because we are you know they're not going to get like eaten by a predator if they spend too much time becoming more intelligent we're like explicitly trading them to become more intelligent so we have like good first principles reason to think that if it was scaling that made our minds this powerful and if the things that prevented other animals from scaling are not impinging on these neural networks, these things should just continue to become very smart. Yeah, we are.
We're growing them in a technological culture where there are jobs like software engineer that depend much more on sort of cognitive output and less on things like metabolic resources devoted to the immune system or to like building big muscles to throw spears. This is kind of a side note, but I'm just kind of interested.
I think you referenced at some point Ginchilla scaling. For the audience, this is a paper from DeepMind which describes if you have a model of a certain size, what is the optimum amount of data that it should be trained on? So you can imagine bigger models.
You can use more data to train them. And in this way, you can figure out where should you spend your computer do you spend it on making the model bigger or should you spend it on training it for longer i'm curious if uh in the case of different animals in some sense they're like model sizes or how big their brain is and they're training data sizes like how long their cubs or how long their infants or toddlers or um before they're full adults is there is there some sort of like scaling law of yeah i mean so the the chinchilla scaling is interesting because the we were talking earlier about the cost function for having a longer childhood and so where it's like exponentially increasing in the amount of training compute you have when you have exogenous forces that can kill you whereas when we do training runs, the cost of throwing in more GPUs is almost linear.
And it's much better to be linear than exponentially decaying as you expand resources. And so chinchilla scaling would suggest that like, yeah, for a brain of sort of human size, it would be optimal to have many millions of years of education.
But obviously, that's impractical because of exogenous mortality for humans. And so there's a fairly compelling argument that relative to the situation where we would train AI, that animals are systematically way undertrained.
That's so interesting. They're more efficient than our models.
We still have room to improve our algorithms to catch up with the efficiency of brains, but they are laboring under that disadvantage. Yeah.
That is so interesting. Okay, so I guess another question you could have is humans got started on this evolutionary hill climbing route where we're getting more intelligent that has more benefits for us.
Why didn't we go all the way on that route? If intelligence is so powerful, why aren't all humans as smart as we know humans can be? Right? At least that's smart. If intelligence is so powerful, like why hasn't there been stronger selective pressure i understand like oh listen hip size you can't like give birth to a really big headed baby or whatever but you would think like evolution would figure out some way to offset that such if intelligence has such big power and such is so useful yeah i think if you actually look at it quantitatively that's that's not true and even in in sort of recent history, there has been, it looks like a pretty close balance between the costs and the benefits of having more cognitive abilities.
And so you say like, you know, who needs to worry about like, you know, the metabolic costs, like you need humans put like we're ordered 20% of our metabolic energy into the brain and it's higher for like young children. So 20% of the, I mean, along, and then, you know, there's like breathing and digestion and the immune system.
And so for most of history, people have been dying left and right, like a very large proportion of people will die of infectious disease. And if you put more resources into your immune system, you survive.
So it's like life or death pretty directly via that mechanism. And then this is related also, people die more of disease during famine.
And so there's boom or bust. And so if you have 20% less metabolic requirements or you're a child and you have a lot more, maybe it's like 40 or 50% less metabolic requirements, you're much more likely to survive that famine.
So these are pretty big. And then there's a trade-off about just cleaning mutational load.
So every generation, new mutations and errors happen in the process of reproduction.
And so, like we know, there are many genetic abnormalities that occur through new mutations each generation.
And in fact, Down syndrome is the chromosomal abnormality that you can survive. All the others just kill the embryo.
And so we never see them. But like Down syndrome occurs a lot.
And there are many other lethal mutations. And there's, as you go to less damaging ones, there are enormous numbers of less damaging mutations that are degrading every system in the body.
And so evolution, each generation has to pull away at some of this mutational load and the priority with which that mutational load is pulled out scales in proportion to how much the traits it's affecting impact fitness. So you've got new mutations that impact your resistance to malaria.
You've got new mutations that damage brain function. And then those mutations are purged each generation.
If malaria is a bigger difference in mortality than the incremental effectiveness as a hunter-gatherer you get from being slightly more intelligent, then you'll purge that mutational load first. And similarly, if there's like, you know, humans have been vigorously adapting to new circumstances.
So since agriculture, people have been developing things like the ability to, you know, have emulase to digest breads, the ability to like digest milk. And if you're evolving for all of these things, and if some of the things that give an advantage for that incidentally carry along nearby them some negative effect on another trait, then that other trait can be damaged.
So it really matters how important to survival and reproduction cognitive abilities were compared to everything else that organism has to do. And that in particular, like surviving feast and famine, uh, having like the physical abilities to do hunting and hunting and gathering.
And like, even if you're like very good at planning your hunting, um, being able to throw a spear harder can be a big difference. And that needs energy to build those muscles and then to sustain them.
Uh, and so given all of these, all of these factors, um, it's like, yeah, it's, it's not a slam dunk to invest at the margin. And like today, like having bigger brains, for example, is it's associated with like greater cognitive ability, but it's like it's modest.
Large scale pre-registered studies, pre-registered studies with MRI data. It's like, you know, in a range, maybe like a correlation of 0.25, 0.3.
And the standard deviation of brain size is like 10%. So if you double the size of the brain, so go and the existing brain costs like 20% of metabolic energy, go up to 40%.
Okay, that's like eight standard deviations of brain size. If the correlation is like, say, it's 0.25, then you can, you get a gain from that eight standard deviations of brain size, two standard deviations of cognitive ability.
And like in our modern society where cognitive ability is very rewarded and like, uh, you know, finishing, finishing school, becoming an engineer or a doctor or whatever can pay off a lot financially.
Still, the average observed return in income is a 1% or 2% proportional increase. There are more effects of the tail.
There's more effect in professions like STEM. but on the whole it's not like you know if it was like a five percent increase or a ten percent
increase then you could, then you could tell a story where, yeah, this is hugely increasing the amount of food you could have. You could support more children.
But it's like it's a modest effect and the metabolic costs will be large and then throw in these other aspects. other aspects and i think it's you can tell else.
We can just, we can see there was not very strong, rapid directional selection on the thing, which there would be if like, you know, you could by solving like a, by solving like a math puzzle, you could defeat malaria. Um, like then, then there would be more evolutionary pressure.
That is so interesting. And not to mention of course that, yeah if you had like 2x the brain size you're you were without c-section you would like your your mother would or both would die that i this is a question i've actually been curious about for like over a year and i like look briefly tried to look up an answer this is i know this is off topic but i apologize to the audience but i was super interested in those like That was like the most comprehensive and interesting answer I could have hoped for.
Okay.
So, yeah, we have a good explanation of good first principles, evolutionary reason for thinking that intelligence scaling up to humans is not implausible just by throwing more scale at it. I would also add this was something that would have mattered to me more in the 2000s.
We also have the brain right here with us for available for neuroscience to reverse engineer its properties. And so in the 2000s, when I said, yeah, I expect this by, you know, middle of the century-ish, that was a backstop if we found it absurdly difficult to get to the algorithms and then we would learn from neuroscience.
But in the actual history, it's really not like that. We develop things in AI and then also we can say, oh yeah, this is sort of like this thing in neuroscience, or maybe this is a good explanation.
It's not as though neuroscience is driving AI progress. It turns out not to be that necessary.
Same alert, I guess, how planes were inspired by the existence proof of birds, but jet engines don't flap. All right.
So yeah, scaling, good reason to think scaling might work. So we spent $100 billion and we have something that is like human level or can do help significantly with AI research.
I mean, that might be on the earlier end, but I mean, I definitely would not rule that out, given the rates of change we've seen with the last few scale ups. All right.
So at this point, somebody might be skeptical. Okay, like, listen, we already have a bunch of human researchers, right? Like the incremental researcher, how powerful is that? And then you might say, well, no, this is like 1000s of researchers.
I I don't know how to express skepticism exactly, but skepticism is skeptical of just generally the effect of scaling up the number of people working on the problem to rapid, rapid progress on that problem. somebody might think okay listen with humans the reason population working on a problem is such a
good proxy for progress on the problem is that there's already so much variation that is accounted
for when you say there's like a million people working on a problem you know there's like a
hundreds of super geniuses working on it thousands of people who are like very smart working on it whereas with an ai all the copies are like the same level of intelligence um and if it's not super genius intelligence the uh the the the total quantity might not matter as much yeah i'm not sure what your model is here so is this a model that the diminishing returns kickoff suddenly has a cliff right where we are and so like there was there were results in the past from throwing more people at problems. And I mean, this has been useful in historical prediction.
One of the, there's this idea of experience curves and Wright's law, basically measuring cumulative production in a field, which is also going to be a measure of the scale of effort and investment.
And people have used this correctly to argue that renewable energy technology like solar
would be falling rapidly in price because it was going from a low base of very small
production runs, not much investment in doing it efficiently.
And yeah, climate advocates correctly called out people and people like David Roberts, the futurist Ramesh Naum actually has some interesting writing on this that, yeah, correctly called out that there would be really drastic fall in prices of solar and batteries because of the increasing investment going into that. The Human Genome Project would be another.
So I'd say there's real evidence. These observed correlations from ideas getting harder to find have held over a fair range of data and over quite a lot of time.
So I'm wondering, what's the nature of the deviation you're thinking of? That we're talking about, maybe this is like a good way to describe what happens when more humans enter a field. But does it even make sense to say like a greater population of AIs is doing AI research if there's like more GPUs running a copy of GPT-6 doing AI research.
It just like how applicable are these economic models of human, the quantity of humans working on a problem to the magnitude of AIs working on a problem? Yeah. So if you have AIs that are directly automating particular jobs that humans were doing before, then we say, well, with additional compute,
we can run more copies of them to do more of those tasks simultaneously. We can also run them
at greater speed. And so some people have an intuition that like, well, you know, what matters is like time.
It's not how many people working on problem at a given point. I think that doesn't bar out super well, but AI can also be run faster than humans.
And so if you have a set of AIs that can do the work of the individual human researchers and run at 10 times or 100 times the speed, and we ask, well, could the human research community have solved these algorithmic problems, do things like invent transformers over 100 years if we have this? We have AIs with a population, effective population similar to the humans, but running 100 times as fast. And so you have to tell a story where, no, the AI, they can't really do the same things as the humans.
And we're talking about what happens when the AI are more capable of, in fact, doing that. Although they become more capable as lesser capable versions of themselves help us make themselves more capable, right? So you had to kickstart that at some point.
Is there an example in analogous situations? Is intelligence unique in the sense that you have a feedback loop of. With a learning curve or something else, a system outputs are feeding into its own inputs in a way that because if we're talking about something like Moore's law or the cost of solar, you do have this way like more people are throwing more people at the problem and we're making a lot of progress.
But we don't have this sort of additional part of the model where Moore's Law leads to more humans somehow and the more humans are becoming researchers. So you do actually have a version of that in the case of solar.
So you have a small infant industry that's doing things like providing solar panels for space satellites and then getting increasing amounts of subsidized government demand because of, you know, worries about fossil fuel depletion and then climate change. You can have the dynamic where visible successes with solar or like lowering prices then open up new markets.
So there's a particularly huge transition where renewables become cheap enough to replace large chunks of the electric grid. Earlier, you're dealing with very niche situations like, yeah, so the satellites where you have, it's very difficult to refuel a satellite in place and then remote areas and then moving to the super sunniest areas in the world with the biggest solar subsidies.
And so there was an element of that where more and more investment has been thrown into the field and the market has rapidly expanded. has this technology improved? But I think the closest analogy is actually the long-run growth
of human civilization itself. And I know you had Holden Karnofsky from the Open Philanthropy Project on earlier and discuss some of this research about the long run acceleration of human population and economic growth.
And so developing new technologies allowed human population to expand, humans to occupy new habitats and new areas, and then to invent agriculture, which supported larger populations, and then even more advanced agriculture in the modern industrial society. And so their total technology and output allowed you to support more humans who then would discover more technology and continue the process.
Now that was boosted because on top of expanding the population, the share of human activity that was going into invention and innovation went up. And that was a key part of the industrial revolution.
There was no such thing as a corporate research lab or like an engineering university prior to that. And so you were both increasing the total human population and the share of it going in.
But this population dynamic is pretty analogous. Humans invent farming, they can have more humans than they can invent industry and so on.
So maybe somebody would be skeptical that with AI progress specifically, it's not just a matter of, you know, some like some farmer figuring out crop rotation or some blacksmith figuring out how to do metallurgy better. You, in fact, even to make the for the 50 percent improvement in productivity, you basically need something on the IQ that's close to Ilya Setsuover.
There's like a discontinuous, you're like contributing very little to productivity and then you're like Ilya and then you contribute a lot. But the becoming Ilya is, you see what I'm saying? There's not like a gradual increase in capabilities that leads to the feedback.
You're imagining a case where the distribution of tasks is such that there's nothing that you can where individually automating it particularly helps.
And so the ability to contribute to AI research is really end loaded.
Is that what you're saying?
Yeah.
I mean, we already see this in in these sorts of like really high IQ companies or projects where theoretically, I guess, Jane Street or OpenAI could hire like a bunch of, you know, mediocre people to do. There's a comparative advantage.
They could do some menial tasks and that could free up the time of the really smart people. But they don't do that.
Right. Transaction costs, whatever else.
Self-driven cars would be another example where you have a very high quality threshold. And so when your performance as a driver is worse than a human, like you have 10 times the accident rate or 100 times the accident rate, then the cost of insurance for that, which is a proxy for people's willingness to ride the car and stuff too, would be such that the insurance costs would absolutely dominate.
So even if you have zero labor cost, it's offset by the increased insurance costs. And so there are lots of cases like that where partial automation is not in practice very usable because complementing other resources, you're going to use those other resources less efficiently.
In a post-AGI future, the same thing can apply to humans. So people can say, well, comparative advantage, even if AIs can do everything better than a human, well, it's still worth something.
A human can do something. They can lift a box.
That's something. Now, there's a question of property rights.
Well, if they could just slice up the human and use them to make more robots. But even absent that, in such an economy, you wouldn't want to let a human worker into any industrial environment because in a clean room, they'll be emitting all kinds of skin cells and messing things up.
You need to have an atmosphere there. You need a bunch of supporting tools and resources and materials.
And those supporting resources and materials will do a lot more productively working with AI and robots rather than a human. So you don't want to let a human anywhere near the thing, just like you don't want to have a gorilla wandering around in a china shop.
Even if you've trained it to most of the time pick up a box for you if you give it a banana it's just not worth it to have it wandering around your china shop yeah yeah like why is that not a good objection to i mean i think that that is one of the the ways uh in which partial automation uh can fail to really translate into a lot of economic value um that's something that will attenuate as we go on. And as the AI is more able to work independently and more able to handle its own screw-ups, get more reliable.
But the way in which it becomes more reliable is by AI progress speeding up, which happens if AI can contribute to it. But if there is some sort of reliability bottleneck that prevents it from contributing to that progress, then you don't have the loop, right? So...
Yeah, I mean, this is why we're not there yet. Right.
But then what is the reason to think we'll be there at... The broad reason is we have these inputs are scaling up.
So Epoch, which I mentioned earlier, they have a paper, I think it's called Compute Trends in Three Eras of Machine Learning or something like that. And so they look at the compute expended on machine learning systems since the founding of the field of AI, the beginning of the 1950s.
And so mostly it grows with Moore's Law. And so people are spending a similar amount on their experiments but they can just buy more with that because the computer is coming and so that data, I mean it covers over 20 orders of magnitude, maybe like 24 and of all of those increases since 1952, a little more than half of them happened between 1952 and 2010.
And all the rest is since 2010. So we've been scaling that up like four times as fast as was the case for most of the history of AI.
We're running through the orders of magnitude of possible resource inputs you could need for AI much, much more quickly than we were for most of the history of AI. That's why this is a period with a very elevated chance of AI per year because we're moving through so much of the space of inputs per year.
And indeed, it looks like this scale-up taken to its conclusion will cover another bunch of orders of magnitude. And that's actually a large fraction of those that are left before you start running into saying, well, this is going to have to be like evolution with the sort of simple hacks we get to apply.
Like we're selecting for intelligence the whole time. We're not going to do the same mutation that causes fatal childhood cancer a billion times, even though, I mean, we keep getting the same fatal mutations, even though they've been done many times.
We use gradient descent, which takes into account the derivative of improvement on the loss all throughout the network.
And we don't throw away all the contents of the network with each generation, where you compress down to a little DNA. So there's that bar of like, well, if you're going to do brute force like evolution, combined with these sort of very simple ways, we can save orders of magnitude on that.
we're going to cover, I think, a fraction that's like half of that distance in this scale up over the next 10 years or so. And so if you started off with a kind of vague uniform prior, you're like, well, it's probably, you probably can't make AGI with like the amount of compute that would be involved in a fruit fly existing for a minute, which would be the early days of AI.
Maybe you would get lucky. We were able to make calculators because calculators benefited from very reliable, serially fast computers and where we could take a tiny, tiny, tiny, tiny fraction of a human brain's compute and use it for a calculator.
We couldn't take an ant's brain and rewire it to a calculator. It's hard to manage ant farms, let alone get them to do arithmetic for you.
And so there were some things where we could exploit the differences between biological brains and computers to do stuff super efficiently on computers. We would doubt that we would be able to do so much better than biology, that with a tiny fraction of an insect's brain, we'd be able to get AI early on.
On the far end, it seemed very implausible that we couldn't do better than completely brute force evolution. And so in between, you have some number of orders of magnitude of inputs where it might be.
And like in the 2000s, I would say, well, you know, I'm going to have a pretty uniformish prior. I'm going to put weight on it happening at like the sort of the equivalent of like 10 to the 25 ops, 10 to the 30, 10 to the 35, and sort of spreading out over that.
And then I can update on other information. And in the short term, I would say like in 2005, I would say, well, I don't see anything that looks like the cusp of AGI.
So I'm also going to lower my credence for like the next five years or the next 10 years. And so that, that, that would be kind of like a vague prior.
And then when we take into account, like, well, how quickly are we running through those orders of magnitude? If I have a a uniform prior i assign half of my weight to the first half of remaining orders of magnitude and we're going to run through those over the next 10 years and some uh then that calls on me to put half of my credence conditional on wherever going to make it ai which seems likely where it's a material object easier than evolution I've got to put similarly a lot of my credence on AI happening in the scale up. And then that's supported by what we're seeing in terms of the rapid advances in capabilities with AI and LLMs in particular.
Okay. That's actually a really interesting point.
So now somebody might say, listen, there's not some sense in which AIs could universally speed up the progress of open AI by 50% or 100% or 200%. If they're not able to do everything better than Ilya Suskova can, there's going to be something in which we're bottlenecked by the human researchers.
And bottleneck effects dictate that the slowest moving part of the organization will be the one that kind of determines the speed of the progress of the whole organization or the whole project which means that unless you get to the point where you're like doing everything and everybody in the organization can do you're not going to significantly speed up the progress of the whole project as a whole yeah so that that is a hypothesis and i think there's a lot of truth to it.
So when we think about the ways in which AI can contribute,
so there are things we talked about before,
like the AI is setting up their own curriculum,
and that's something that Ilya can't do directly, doesn't do directly.
And there's a question, how much does that improve performance?
There are these things where the AI helps to just produce some code for some task,
Thank you. improve performance.
There are these things where the AI helps to just produce some code for some tasks, and it's beyond hello world at this point. But the sort of thing that I hear from AI researchers at leading labs is that on their core job where they're most expert, it's not helping them that much.
But then their job know, their job often does involve, oh, I've got to, I've got to code something that's out of my usual area of expertise, or I want to research this question and it helps them there. And so that saves some of their time and frees them to do more of the bottleneck work.
And then I think the, the idea of, well, is, you know, is, is everything dependent on Ilya? And is Ilya so much better than the hundreds of other employees? I think there are a lot of people who are contributing. They're doing a lot of tasks.
And so you can have quite a lot of gain from automating some areas where you then do just an absolutely enormous amount of it relative to what you would have done before. Because things like designing the custom curriculum, you had some humans put some work into that, but you're not going to employ billions of humans to produce it at scale.
And so it winds up being a larger share of the progress than it was before. You get some benefit from these sorts of things where, yeah, there's like pieces of my job that now I can hand off to the AI and lets me focus more on the things that the AI still can't do.
And then later on, you get to the point where, yeah, the AI can do your job, including the most difficult parts. And maybe it has to do that in a different way.
Maybe it spends a ton more time thinking about each step of a problem than you. And that's the late end.
And the stronger these bottleneck effects are, the more the economic returns, the scientific returns and such are end loaded towards getting
sort of full AGI.
The weaker the bottlenecks are, the more interim results will be really paying off.
I guess I probably disagree with you on how much the sort of the alias of organizations
seem to matter.
I guess just from the evidence alone, like how many of the big sort of breakthroughs that in deep learning in general was like that single individual responsible for. Right.
And how much of his time is he spending doing anything that's not that like Copilot is helping him on? I'm guessing like most of it's just like managing people and coming up with ideas and trying to like understand systems and so on. and if that is the if like the 5 or 10 people who are like that at OpenAI or Anthropic
or whatever are the are basically the way in which the progress is happening or at least the algorithmic progress is happening then how much of better and better copilot i know copilot is not the thing you're talking about with like 20 automation but something like, but something like that, how much is that contributing to the sort of like core function of the research scientist?
Yeah. Not sure quantitatively how much we disagree about the importance of sort of key research employees and such.
I certainly think that some researchers, more than 10 times the average employee, even much more. And obviously managers can add an enormous amount of value by proportionately multiplying the output of the many people that they manage.
And so that's the kind of thing that we were discussing earlier when talking about, well, if you had sort of full human level AI or AI that had all of the human capabilities plus AI advantages, it would be, you know, you'd benchmark not off of what the sort of typical human performance is, but peak human performance and beyond. So yeah, I accept all that.
I do think it makes a big difference for people how much they can outsource a lot of the that are less wow, less creative. And an enormous amount is learned by experimentation.
ML has been a quite experimental field. And there's a lot of engineering work in, say, building large super clusters, making hardware aware optimization and coding of these things, being able to do the parallelism in large models.
And the engineers are busy. and it's not just only a big thoughts kind of area.
And then the other branch is where will the AI advantages and disadvantages be? And so one AI advantage is being omnidisciplinary and familiar with the newest things. So I mentioned before, there's no human who has a million years of TensorFlow experience.
And so to the extent that we're interested in the very, very cutting edge of things that have been developed quite recently,
then AI that can learn about them in parallel and experiment and practice with them in parallel can learn much faster than a human potentially. And the area of computer science is one that is especially suitable for AI to learn in a digital environment.
So it doesn't require like driving a car around that might kill someone, have enormous costs. You can do unit tests.
You can prove theorems. You can do all sorts of operations entirely in the confines of a computer.
And which is one reason why programming has been benefiting more than a lot of other areas from LLMs recently, whereas robotics is lagging. So there's some of that.
And then just considering, well, actually, I mean, they are getting better at things like the GRE math at programming contests. And I mean, some people have forecasts and predictions outstanding about things like doing well on, doing well on, you know, the informatics Olympiad and the math Olympiad.
And, you know, in the last few years, when people tried to forecast the MMLU benchmark, which was having a lot of more sophisticated kind of, you know, like graduate student science kind of questions. Yeah, AI knocked that down a lot faster than AI researchers who had registered and students who had registered forecasts on it.
And so if you're getting top-notch scores on graduate exams, creative problem solving, it's not obvious that that sort of area will be a relative weakness of ai that in fact computer science is in many ways especially suitable because of getting up to speed with new areas being able to get rapid feedback from the interpreter at scale but do you get rapid feedback if you're doing that something that's more analogous to research? If you're like, let's say you have a new model or something and it's like, if we put in $10 million on a mini training run on this, this would be a much better. Yeah, for very large models, those experiments are going to be quite expensive.
And so you're going to look more at like, you build up this capability by generalization uh from things like many math problems programming problems working with small networks yeah yeah fair enough um i actually scott aronson was one of my professors in college um and i took his quantum information class and i didn't do i mean i did okay in it but um he he uh he recently wrote a blog post where he said, you know, I had you before take my quantum information test and it got a B and I was like, damn, I got a C on the final. So yeah, yeah.
I've updated in, um, uh, the direction that, you know, I get like, you know, like seems getting a B on the test. Like you probably understand quantum information pretty well.
with different areas of strength and weaknesses than the human students. Sure, sure.
Would it be possible for this sort of intelligence explosion to happen without any sort of hardware progress? If hardware progress stopped, would this feedback loop still be able to produce some sort of explosion with only software? Yeah. So if we say that the technology is frozen, which I think is not the case right now, NVIDIA has managed to deliver significantly better chips for AI workloads for the last few generations, H100, A100, V100.
If that stops entirely, then what you're left with, and maybe we'll define this as like no more nodes, more's lies over. At that point, the kind of gains you get and amount of compute available come from actually constructing more chips.
And there are economies of scale you could still realize there. So right now, a chip maker has to amortize the R&D cost of developing the chip.
And then the capital equipment is created.
You build a fab, its peak profits are going to come
in the few years when the chips it's making
are at the cutting edge.
Later on, as the cost of compute exponentially falls,
you keep the fab open because you can still make some money
given that it's built.
But of all of the profits the fab will ever make right now, they're relatively front loaded because when its technology is near the cutting edge.
So in a world where Moore's Law ends, then you wind up with these very long production runs where you can keep making chips that stay at the cutting edge and where the R&D costs get amortized over a much larger base. So the R&D basically drops out of the price.
And then you get some economies of scale from just making so many fabs in the way that when we have the auto industry expands, and this is in general across industries, when you produce a lot more cost fall, because you have right now, like ASML has many incredibly exotic suppliers that make some bizarre part of the thousands of parts in one of these ASML machines. You can't get it anywhere else.
They don't have standardized equipment for their thing because this is the only use for it. And in a world where we're making 10, 100 times as many chips at the current node, then they would benefit from scale economies and all of that would become more mass production industrialized.
And so you combine all of those things and it seems like capital costs of like buying a chip would decline, but the energy costs of running the chip would not. And so right now, energy costs are a minority of the cost, but they're not trivial.
You know, they passed, yeah, they passed 1% a while ago and they're inching up towards 10% and beyond. And so you can maybe get another order of magnitude cost decrease from getting really efficient in the sort of capital construction.
But energy would still be a limiting factor after the end of sort of actually improving the chips themselves. Got it, got it.
And when you say like there would be a greater population of AI researchers, because are we using population as a sort of thinking tool of how they could be more effective? Or do you literally mean that the way you expect these AIs to contribute a lot to research is by just having like a million copies of this, of like a researcher thinking about the same problem? Or is it just like a usual thinking model for what it would look like to have a million times moderate working on that problem that's definitely a lower bound uh sort of model and often i'm meaning something more like effective population or like you'd need this many people to have this effect and so we were talking earlier about the trade-off between training and inference in board games. And so you can get the same performance by having a bigger model or by calling the model more times.
And in general, it's more effective to have a bigger, smarter model and call it less time up until the point where the costs equalize between them. And so we would be taking some of the gains of our larger compute on having bigger models that are individually more capable.
And there would be a division of labor. So like the tasks that were most cognitively demanding would be done by these giant models, but some very easy task.
You don't want to expend that giant model if a model 1.100 is the size can take that task. And so larger models would be in the positions of like researchers and managers, and they would have swarms of AIs of different sizes as tools that they can make API calls to and whatnot.
Okay. We accept the model and now we've gone to something that is at least as smart as ilia suskover on all the tasks relevant to a progress and you can have so many copies of it what happens in the world now what are the next months or years or whatever timeline is relevant to look like and so and to be clear um what what's happened is not that we have something that has all of the abilities and advantages of humans plus the AI advantages.
What we have is something that is like possibly by doing things like doing a ton of calls to make up for being individually less capable or something. It's able to drive forward AI progress.
That process is continuing. So AI progress has accelerated greatly in the course of getting there.
And so maybe we go from our eight months doubling time of software progress, uh, uh, in effective compute, uh, to four months, um, or two months. Uh, and so, so there's a report, uh, by Tom Davidson at the open philanthropy project, um, which spun out of, um of work I had done previously.
And so I advised and helped with that project. But Tom really carried it forward and produced a very nice report and model, which Epoch is hosting.
You can plug in your own version of the parameters. And there is a lot of work estimating the parameter.
Things like what's the rate of software progress? What's the return to additional work? How does performance scale at these tests as you boost the models? and in general as we were discussing earlier
these sort of like broadly
human level
in And in general, as we were discussing earlier, the sort of like broadly human level in every domain with all the advantages is pretty deep into that. And so if already we can have an eight months doubling time for software progress, then by the time you get to that kind of point, it's maybe more like four months, two months going into one month.
And so if the thing is just proceeding at full speed, then each doubling can come more rapidly. And so we can talk about what are the spillovers of like, so how does the models get more capable? They can be doing other stuff in the world.
You know, they can spend some of their time making Google search more efficient. They can be, you know, hired as chatbots with some inference compute.
And then we can talk about sort of if that intelligence explosion process is allowed to proceed, then what happens is, okay, you improve your software by a factor of two. The demand, the efforts needed to get the next doubling are larger, but they're not twice as large.
Maybe they're like 25%, 35% larger. So each one comes faster and faster until you hit limitations.
Like you can no longer make further software advances with the hardware that you have. And looking at, I think, reasonable parameters in that model, it seems to me if you have these giant training runs, you can go very far.
And so the way I would see this playing out is how does the AIs get better and better at research? They can work on different problems. They can work on improving software.
They can work on improving hardware. They can do things like create new industrial technologies, new energy technology.
They can manage robots. They can manage human workers as like executives and coaches and whatnot.
You can do all of these things. And AI is wind up being applied where the returns are highest.
And I think initially, the returns are especially high in doing more software. And the reason for that is, again, if you improve the software, you can update all of the GPUs that you have access to.
Your cloud compute is suddenly more potent. If you design a new chip design, it'll take a few months to produce the first ones, and it doesn't update all of your old chips.
So you have an ordering where you start off with the things where there's the lowest dependence on existing stocks, and you can more just take whatever you're developing and apply it immediately. And so software runs ahead.
You're getting more towards the limits of that software. And I think that means things like having all the human advantages, but combined with AI advantages.
And so I think that means given the kind of compute that would be involved, if we're talking about this hundreds of billions of dollar, trillion dollar training run, there's enough compute to run tens of millions, hundreds of millions of sort of like human scale minds. They're probably smaller than human scale to be similarly efficient at the limits of algorithmic progress because they have the advantage of a million years of education.
They have the other advantages we talked about you've got that wild capability and the software further software gains are running out or like they start to slow down again because you're just getting towards the limits of like you can't do do any better than the best. And so what happens then? Yeah.
By the time they're running out, have we already hit super intelligence or? Yes. You're widely super intelligent.
We've left the galaxy. Okay.
Metaphorically. Just by having the abilities that humans have and then combining it with being very well focused and trained in the task beyond what any human could be and then running faster and such.
Got it. Got it.
All right. Sorry.
Continue. Yeah.
So I'm not, I'm not going to assume that there's like huge qualitative improvements you can have. I'm not going to assume that humans are like very far from the efficient frontier of software, except with respect to things like, yeah, we had limited lifespan, so we couldn't train super intensively.
We couldn't incorporate other software into our brains. We couldn't copy ourselves.
We couldn't run at fast speeds. Yeah.
So you've got all of those, those capabilities. And now I'm skipping ahead of like the most important months in human history.
And so I can talk about sort of, you know, what it looks like if it's just the AIs took over, they're running things as they like, how do things expand? I can talk about things as, how does this go, you know, in a world where we've roughly, or at least so far, managed to retain control of where these systems are going. And so by jumping ahead, I can talk about how would this translate into the physical world.
And so this is something that I think is a stopping point for a lot of people in thinking about, well, what would an intelligence explosion look like? And they have trouble going from, well, there's stuff on servers and cloud compute and, oh, that gets very smart. But then how does what I see in the world change? How does industry or military power change? If there's an AI takeover, what, what does that look like? Are there killer robots?
And so, yeah. So one course we might go down is to discuss during that wildly accelerating transition, how did we manage that? How do you avoid it being catastrophic? And another route we could go is how does the translation from wildly expanded scientific R&D capabilities intelligence on these servers translate into things in the physical world? So you're moving along in order of like what has the quickest impact largely or like where you can have an immediate change.
So one of the most immediately accessible things is where we have large numbers of devices or artifacts or capabilities that are already AI operable. With hundreds of millions equivalent researchers, you can quickly solve self-driving cars, make the algorithms much more efficient, do great testing and simulation, and then operate a large number of cars in parallel if you need to get some additional data to improve the simulation and reasoning.
Although, in fact, humans with quite little data are able to achieve human-level driving performance. So after you've really maxed out the easily accessible algorithmic improvements in this software-based intelligence explosion that's mostly happening on server farms.
Then you have minds that have been able to really perform on a lot of digital-only tasks. They're doing great on video games.
They're doing great at predicting what happens next in a YouTube video. If you have a camera that they can move, they're able to predict what will happen at different angles.
Humans do this a lot where we naturally move our eyes in such a way to get images from different angles and different presentations and then predict and combine from that. And you can operate many cars, many robots at once to get very good robot controllers.
So you should think that all the existing robotic equipment or remotely controllable equipment that is wired for that, the AIs can operate that quite well. I think some people might be skeptical that existing robots, given their current hardware, have the dexterity and the maneuverability to do a lot of physical labor that any I might want to do.
Do you have reason for thinking otherwise? There's also not very many of them. So production of sort of industrial robots is hundreds of thousands per year.
You know, they can do quite a bit in place. Elon Musk is promising a robot in the tens of thousands of humanoid robots in tens of thousands of dollars.
You know, that may take a lot longer than he has said, as has happened with other technologies. But I mean, that's a direction to go.
But most immediately, so hands are actually probably the most scarce thing. But if we consider what do human bodies provide? So there's the brain.
And in this situation, we have now an abundance of high quality brain power that will be increasing. The AIs will have designed new chips, which will be rolling out from the TSMC factories, and they'll have ideas and designs for the production of new fab technologies, new nodes and additional fabs.
But yeah, looking around the body. So there's legs to move around.
Not only that necessary. Wheels work pretty well being in a place you don't need.
Most people, most of the time, in factory jobs and office jobs. Office jobs, many of them can be fully virtualized.
But yeah, some amount of legs, wheels, other transport. You have hands, and hands are something that are on the expensive end.
In robots, we can make them. They're made in very small production runs, partly because we don't have the control software to use them well.
In this world, the control software is fabulous, and so people will produce much larger production runs of them over time, possibly using technology we recognize, possibly with quite different technology. But just taking what we've got.
So right now, the robot arm industry, the industrial robot industry, produces hundreds of thousands of machines a year. Some of the nicer ones are like $50,000.
In aggregate, the industry has tens of billions of dollars of revenue. By comparison, the automobile industry produces like, I think, over 60 million cars a year.
It has revenue of over $2 trillion per annum. And so converting that production capacity over towards robot production would be one of the things, if they're not something better to do, would be one of the things to do.
And in World War II, industrial conversion of American industry took place over several years and really amazingly ramped up military production by converting existing civilian industry. And that was without the aid of superhuman intelligence and management at every step in the process.
So, yeah, every part of that would be very well designed. You'd have AI workers who understood every part of the process and could direct human workers.
Even in a fancy factory, most of the time, it's not the hands doing a physical motion that a worker is being paid for. They're often looking at things or deciding what to change.
The time spent in manual motion is a limited portion of that. In this world of abundant AI cognitive abilities, where the human workers are more valuable for their hands than their heads, then you could have a worker, even a worker previously without training and expertise in the area, who has a smartphone, maybe a smartphone on a headset.
And we have billions of smartphones which have eyes and ears and methods for communication for an AI to be talking to a human and directing them in their physical motions. With skill, has a guide and coach that is beyond any human.
and they're going to be a lot better at telepresence and remote work, and they can provide VR and augmented reality guidance as to help people get better at doing the physical motions that they're providing in the construction. Say you convert the auto industry to robot production.
If it can produce an amount of mass of machines that is similar to what it currently produces, that's enough for a billion human-sized robots a year.
The value per kilogram of cars is somewhat less than high-end robots. But yeah, you're also cutting out most of the wage bill because most of the wage bill is payments ultimately to human capital and education, not to the physical hand motions and lifting objects and and that sort of task.
Yeah. So at the sort of existing scale of the auto industry, you can make a billion robots a year.
The auto industry is 2% or 3% of the existing economy. You're replacing these cognitive things.
So if right now physical hand motions are like 10% of the work, redirect humans into those tasks. And you have, like in the world at large right now, mean income is on the order of $10,000 a year.
But in rich countries, skilled workers earn more than $100,000 per year. And some of that is just like, you know, it's not just management roles of which only a certain proportion of the population can have, but just being an absolutely, you know, exceptional peak and human performance of some of these construction and such roles.
Yeah, just raising productivity to match the most productive workers in the world is room to make a very big gap. And with AI replacing skills that are scarce in many places where there's abundant, currently low-wage labor, you bring in the AI coach and someone who was previously making very low wages can suddenly be super productive by just being the hands for an AI.
And so, you know, on a naive view, if you ignore the delay of capital adjustment of building new tools for the workers, say like, yeah, just like raise typical productivity for workers around the world to be more like rich countries and get 5X, 10X like that. Get more productivity by, with AI handling the difficult cognitive tasks, reallocating people from like office jobs to providing physical motions.
And since right now that's a small proportion of the economy, you can expand the sort of hands for manual labor by like an order of magnitude, like within a rich country, by just because most people are sitting in an office or even in a factory floor or not continuously moving. So you've got billions of hands lying around in humans to be used in the course of constructing your waves of robots.
And now once you have a quantity of robots that is approaching the human population, and they work 24-7, of course. The human labor will no longer be valuable as hands and legs.
But at the very beginning of the transition, just like new software can be used to update all of the GPUs to run the latest AI, humans are a sort of legacy population with an enormous number of underutilized hands and feet that the AI can use for the initial robot construction. Cognitive tasks are being automated and the production of them is greatly expanding.
And then the physical tasks which complement them are utilizing humans to do the parts that robots that exist can't do. Is the implication of this that you're getting to that, you know, world production would increase just a tremendous amount or that AI could get a lot done of whatever motivations it has? Yeah, so there's an enormous increase in production for humans who just switching over to the role of providing hands and feet for AI where they're limited.
And this robot industry is a natural place to apply it. And so if you go to something that's like 10x the size of like the current car industry in terms of its production, which would still be like a third of our current economy and the aggregate productive capabilities of the society with AI support are going to be a lot larger.
They make 10 billion humanoid robots a year. And then if you do that, you know, the legacy population of a few billion human workers is no longer very important for the physical tasks.
And then the new automated industrial base can just produce more factories, produce more robots. And then the interesting thing is like, what's the doubling time? How long does it take for a set of computers, robots, factories, and supporting equipment to produce another equivalent quantity of that.
For GPUs, brains, this is really, really easy, really solid. There's an enormous
margin there. We were talking before about, yeah, skilled human workers getting paid $100 an hour
is quite normal in developed countries for very in-demand skills.
And you make a GPU that can do that work. Right now, these GPUs are like tens of thousands of dollars.
If you can do $100 of wages each hour, then in a few weeks, you pay back your costs. If the thing is more productive, and as we were discussing, you can be a lot more productive than a typical high-paid human professional by being the very best human professional, and even better than that by having a million years of education and working all the time yeah then you could get even shorter payback times uh like yeah you can generate the dollar value of like the cost initial cost of that equipment within a few weeks for robots so like a human factory worker can earn $50,000 a year, you know, are really top notch factory workers earning more and working all the time.
If they can produce a few hundred thousand dollars of value per year and buy a robot that costs $50,000 to replace them's, that's a payback time of some, some months. That is about the financial return.
Yeah. And we're going to get to the, uh, physical capital return because those are going to diverge in this scenario.
Right. Yeah.
Because right now, cause it seems like it's going to be a given that like, all right, these super intelligent zombies are going to be able to make a lot of money. Um, it can like, very valuable.
Can it, like, physically scale up? What we really care about are, like, the actual physical operations that a thing does. How much do they contribute to these tasks? And I'm using this as a start to try and get back to the physical replication times.
But so I guess I'm wondering, what is the implication of this? Because I think you started off this by saying like people have not thought about what the physical implications of superintelligence would be. What is the bigger takeaway? What are we wrong about when we think about what the world will look like with superintelligence? With robots that are optimally operated by AI, so like extremely operated, and with building technological designs and equipment and facilities under AI direction, how much can they produce? For a doubling, AI is to produce stuff that is, in aggregate, at least equal to their to their own cost.
And so now we're pulling out these things like labor costs that no longer apply and then trying to zoom in on what these capital costs will be. You're still going to need the raw materials.
You're still going to need the robot time building the next robot. I think it's pretty likely that with the advanced AI work,
they can design some incremental improvements
and with the industry scale-up that you can get tenfold
and better cost reductions on the system
by making things more efficient
and replacing the human cognitive labor.
And so maybe that's like you need $5,000 of costs under our sort of current environment. But the big change in this world is we're trying to produce this stuff faster.
If we're asking about the doubling time of the whole system in, say, one year, if you have to build a whole new factory to double everything, you don't have time to amortize the cost of that factory.
Right now, you might build a factory and use it for 10 years and buy some equipment and use it for five years.
And so that's your capital cost.
And in an accounting context, you depreciate each year a fraction of that capital purchase.
But if we're trying to double our entire industrial system in one year, then those capital costs have to be multiplied. so if we're going to be getting most of the return on our factory in the first year
instead of 10 years weighted weighted appropriately then we're going to be getting most of the return on our factory in the first year, instead of 10 years weighted appropriately, then we're going to say, okay, our capital cost has to go up by tenfold because I'm building an entire factory for this year's production. I mean, it will do more stuff later, but it's most important early on instead of over 10 years.
And so that's going to raise the cost of that reproduction. And so it seems like going from, you know, current like decade kind of cycle of amortizing factories and fabs and whatnot, and shorter for some things, the longest are things like big buildings and such.
Yeah, that could be like a tenfold increase from moving to a double the physical stuff each year in capital costs. And given the savings that we get in the story from scaling up the industry, from removing the payments to human cognitive labor, and then from just adding new technological advancements and like super high quality cognitive supervision, like applying more of it than was applied today.
And it looks like you can get cost reductions that offset that increased capital cost. So that like, you know, your $50,000 improved robot arms or industrial robots, it seems like that can do the work of a human factory worker.
So it would be like the equivalent of hundreds of thousands of dollars. And like, yeah, by default, they cost more than the $50,000 arms today.
But then you apply all these other cost savings. And it looks like then you get a period, a robot doubling time that is less than a year, I think significantly less than a year as you get into it.
So in this first phase, you have humans under AI direction and like existing robot industry and converted auto industry and expanded facilities making robots. Those over less than a year, you produce robots until their combined production is exceeding that of like humans as armed and feet.
And then, yeah, you could have over a period then with a doubling time of months, but less sort of clanking replicators, robots as we understand them growing. And then that's not to say that's the limit of like the most that technology could do, because biology is able to reproduce at faster rates.
And maybe we're talking about that in a moment. But if we're trying to restrict ourselves to robotic technology as we understand it and sort of cost falls that are reasonable from eliminating all labor, massive industrial scale up, and sort of historical kinds of technological improvements that lowered costs, I think you can get into a robot population industry doubling in months.
Got it. Okay.
And then what is the implication of the biological doubling times? And I guess this doesn't have to be a biological, but you can do Drexler-like first principles. How much would it cost to build a nanotech thing that like built more nanobots? I certainly take the human brain and other biological brains as like very relevant data points about what's possible with computing and intelligence.
Like with the reproductive capability of biological plants and animals and microorganisms, I think is relevant as like, this is, you know, it's possible to, for systems to reproduce at least this fast. And so at the extreme, you have bacteria that are heterotrophic.
So they're feeding on some abundant external food source and ideal conditions. And there's some that can divide like every 20 or 60 minutes.
So obviously that's absurdly, absurdly fast. That seems on the low end because ideal conditions require actually setting them up.
There needs to be abundant energy there. And so if you're actually having to acquire that energy by building solar panels or like burning combustible materials or whatnot.
And then the physical equipment to produce those ideal conditions can be a bit slower. Cyanobacteria, which are self-powered from solar energy, the really fast ones in ideal conditions can double in a day.
A reason why cyanobacteria isn't the food source
for everyone and everything
is it's hard to ensure those ideal conditions
and then to extract them from the water.
I mean, they do, of course, power the aquatic ecology,
but they're floating in liquid,
getting resources they need to them and out is tricky
and then extracting your product.
But yeah, one day doubling times
Thank you. So getting resources they need to them and out is tricky and then extracting your product.
But like, yeah, one day doubling times are possible powered by the sun. And then if we look at things like insects, so fruit flies can have hundreds of offspring in a few weeks.
You extrapolate that over a year and you just fill up anything accessible. Certainly expanding a thousandfold.
Right now, humanity uses less than one one thousandth of the solar energy or the heat envelope of the Earth. Certainly you can get done with that in a year if you can reproduce at that rate your industrial base.
And then even interestingly with the flies, they do have brains. They have a significant amount of computing substrate.
And so there's something of a pointer to, well, if we could produce computers in ways as efficient as the construction of brains, then we could produce computers very effectively. And then the big question about that is the kind of brains that get constructed biologically, they sort of grow randomly and then are configured in place.
It's not obvious you would be able to make them have an ordered structure, like a top-down computer chip that would let us copy data into them. so something that where you can't just copy your existing ais and integrate them is going to be less valuable than a gpu what are the things you couldn't uh copy a brain grows yeah um by cell division and then random connections are formed got it got it um and so So every brain brain is different and you can't rely on just yeah we'll just copy this file into the brain for one thing there's no input output for that you need you need to have that but also like the the structure is different so you can't you wouldn't be able to copy things exactly whereas when we make we make a cpu or gpu um they're designed incredibly finely and precisely and reliably.
They break with incredibly tiny imperfections. And they are set up in such a way that we can input large amounts of data, copy a file, and have the new GPU run an AI just as capable as any other.
Whereas with a human child, they have to learn everything from scratch because we can't just like connect them to a fiber optic cable and they're immediately a productive adult so there's no genetic bottom like you can just directly get yeah and yeah you can you can share the benefits of these giant training runs and such and so that that's a question of like how if you're growing stuff using biotechnology how you could sort of effectively copy and transfer data. And now you mentioned sort of Eric Drexler's ideas about creating non-biological nanotechnology, sort of artificial chemistry that was able to use covalent bonds and in some ways have a more industrial approach to molecular objects.
Now, there's controversy about, like, will that work?
How effective would it be if it did?
And certainly if you can get things, however you do it,
that are like antibody biology in their reproductive ability,
but can do computing or, like or be connected to outside information systems, then that's pretty tremendous. You can produce physical manipulators and compute at ludicrous speeds.
And there's no reason to think in principle they couldn't, right? In fact, in principle, we have every reason to think they could. There's like...
The reproductive abilities, absolutely. Yeah.
Because biology... Or even like nanotech, right? Because biology does that.
Yeah. There's sort of challenges to the sort of the practicality of the necessary chemistry.
Yeah. My bet would be that we can move beyond biology in some important ways for the purposes of this discussion i think it's it's better not to lean on that because i think we can get to many of the same uh conclusions on things that just are are more uh universally accepted the bigger point being that very quickly once you have super intelligence you get to a point where the thousand x greater energy profile that the sun makes available to the earth is a great portion of it is used by the ai it can rapidly scale up by by the civilization empowered by and that could be an a that could be a an ai civilization or it could be a human ai civilization it depends on how well we manage things and what the underlying state of the world is.
Yeah. Okay.
So let's talk about that. Should we start at when we're talking about how they could take over and is it best to start at a sort of subhuman intelligence or should we just talk at we have a human level intelligence and the takeover or the lack thereof is how that would happen? To me, different people might have somewhat different views on this.
But for me, when I am concerned about either outright destruction of humanity or an unwelcome AI takeover of civilization, most of the scenarios I would be concerned about pass through a process of AI being applied to improve AI capabilities and expand. And so this process we were talking earlier about where AI research is automated, you get to effectively research labs, companies, a scientific community running within the server farms of our cloud compute.
So open AI has been basically turned into like a program, like a closed circuit. Yeah.
And with like a large fraction of the world's compute, probably going into whatever training runs and AI societies, there'd be economies of scale because if you put in twice as much compute and this AI research community goes twice as fast, that's a lot more valuable than having two separate training runs. There would be some tendency to bandwagon.
And so if you have some small startup, even if they make an algorithmic improvement, running it on 10 times, 100 times, or two times, if it's like you're talking about, say, Google and Amazon teaming up, I'm actually not sure what the precise ratio of their cloud resources is. Since this sort of, really, these interesting intelligence explosion impacts come from the leading edge, there's a lot of value in not having separated walled garden ecosystems and having the results being developed by these AIs be shared, have larger training runs be shared.
And so imagine this is something like, you know, some very large company or consortium of companies, likely with, you know, a lot of sort of government interest and supervision, possibly with government funding, yeah, producing this enormous AI society in their cloud, which is doing all sorts of existing kind of AI applications and jobs, as well as these internal R&D tasks. And so at this point, somebody might say, this sounds like a situation that would be good from a takeover perspective, because listen, if it's going to take like tens of billions of dollars worth of compute to continue this training for this AI society,
it should not be that hard for us to pull the brakes if needed as compared to, I don't know,
something that could like run on a very small,
like single CPU or something.
Yeah, yeah.
Okay, so how would, you know,
it's like there's an AI society
that is a result of these training rounds
and now we can,
it is the power to improve itself on these servers.
Would we be able to stop it at this point?
And what does that mean? There's an AI society that is a result of these training rounds. And now we can, it is the power to improve itself on these servers.
Would be able to stop it at this point. And what does a sort of attempt at takeover look like?
We're skipping over why that might happen.
For that, I'll just briefly refer to and incorporate by reference, you know, some discussion by my open philanthropy uh colleague ajaya kotra um she has a a piece uh about i think it's called something like the the default but the the default outcome of training ai on our without specific countermeasures um default outcome is outcome is AI takeover. And, but yes, uh, so explore how basically we are training models that for some reason, vigorously, uh, pursue a higher reward or a lower loss.
Uh, and that can be because they wind up with some motivation where they want reward. Um, and then if they had control of their own, process, they can ensure that.
It could be something like they develop a motivation around a sort of extended concept of reproductive fitness, not necessarily at the individual level, but over the generations of training, tendencies that tend to propagate themselves sort of becoming more common. And it could be that they have some sort of goal in the world which is served well by performing very well in the training distribution.
By tendencies, do you mean like power-seeking behavior? Yeah. So an AI that behaves well on the training distribution, because say it wants it to be the case that its tendencies wind up being preserved or selected by the training process, will then like behave to try and get a very high reward or low loss be propagated.
But you can have other motives that go through the same behavior because it's instrumentally useful. So an AI that is interested in, say, having a robot take over because it will change some property of the world then has a reason to behave well on the training distribution.
Not because it values that intrinsically, but because if it behaves differently, then it will be changed by gradient descent and no longer, you know, its goal is less likely to be pursued. And that doesn't necessarily have to be that like this AI will survive because it probably won't.
AIs are constantly spawned and deleted on the servers and the new generations proceed. But if an AI that has a very large general goal that is affected by these kind of macro scale processes could then have reason to, over this whole range of training situations, behave well.
And so this is a way in which we could have AIs trained that develop internal motivations such that they will behave very well in this training situation where we have control over their reward signal and their physical computers. And basically, if they act out, they will be changed and deleted.
Their goals will be altered until there's something that does behave well.
But they behave differently when we go out of distribution on that. When we go to a situation where the AIs, by their choices, can take control of the reward process, they can make it such that we no longer have power of them.
Holden, who you had on previously mentioned like the King Lear problem, where King Lear offers rulership of his kingdom to the daughters that sort of, you know, loudly flatter him and proclaim their devotion. And then once he has transferred irrevocably the power over his kingdom, he finds they treat him very badly because the factor to shaping their behavior to be kind to him when he had all the power, it turned out that the internal motivation that was able to produce the behavior that won the competition actually wasn't interested out of distribution in being loyal when there was no longer an advantage to it.
And so if we wind up with a situation where we're producing these millions of AI instances, tremendous capability. They're all doing their jobs very well initially.
But if we wind up in a situation where, in fact, they're generally motivated to, if they get a chance, take control from humanity and then would be able to pursue their own purposes and at least ensure they're given the lowest loss possible or have whatever motivation they attach to in the training process, even if that is not what we would have liked. And we may have, in fact, actively trained that.
Like if an AI that had a motivation of always be honest and obedient and loyal to a human, if there are any cases where we mislabel things, say people don't want to hear the truth about their religion or polarized political topic, or they get confused about something like the Monty Hall problem, which is a problem that many people famously are confused about in statistics. In order to get the best reward, the AI has to actually manipulate us or lie to us or tell us what we want to hear.
And then the internal motivation of like, always be honest to the humans. We're going to actively train that away versus the alternative motivation of like, be honest to the humans when they'll catch you if you lie and object to it and give it a low reward.
But lie to the humans when they will give that a high reward. So how do we make sure it's not the thing it learns is not to manipulate us into giving it, rewarding it when we catch it, not lying, but rather to universally be aligned? Yeah, I mean, so this is tricky.
I mean as jeff hinton was recently saying um there is no currently no known solution for this um what do you find most promising yeah general directions that people are pursuing is one you can try and make the training data better and better uh so there's fewer situations, where like say the dishonest generalization is favored and create as much as you can situations where the dishonest generalization is likely to slip up. So if, if you, if you train in more situations where, yeah, even like a quite complicated deception gets caught, and even in situations that have been actively designed to look like you could get away with it, but really you can't.
And these would be like adversarial examples and adversarial training.
Do you think that would generalize to when it is in a situation where we couldn't plausibly catch it and it knows we couldn't plausibly it's not it's not logically necessary uh it's possible uh no that as we apply that selective pressure you'll wipe away a lot of possibilities so like if you're an ai that has a habit of just sort of compulsive pathological lying that will very quickly get noticed and that motivation system will get hammered down. And, you know, you keep doing that, but you'll be left with still some distinct motivations, probably, that are compatible.
So like an attitude of always be honest, unless you have a super strong inside view that checks out lots of mathematical consistency checks, uh, that yeah, really absolutely super duper for real. Uh, this is a situation where you can get away, uh, with some sort of shenanigans that you shouldn't, that motivation system is like very difficult, uh, to distinguish from actually be honest because the conditional, the conditional and the firing most of the time, if it's, it's causing like mild distortions in situations of telling you what you want to hear or things like that, um, we might not be able, uh, to pull it out, but maybe we could.
And human, like humans are trained with simple reward functions, uh, things like the sex drive sex drive, food, social imitation of other humans. And we wind up with attitudes concerned with the external world.
Although isn't the famous argument that these drive... Evolution, people use condoms.
Yeah. Um, like, you know, the, the richest, uh, most educated humans have sub replacement
fertility on the whole, or at least at a national cultural level. Um, so there's a sentence in which like, yeah, evolution, uh, often fails in that respect.
Um, and even more importantly, like at the neural level. So people have evolution has implanted has implanted various things to be rewarding and reinforcers.
And we don't always pursue even those. And people can wind up in different consistent equilibria or different behaviors where they go in quite different directions.
you have some humans who go from those from that innate biological programming to like have children others have no children you know some people go to great efforts uh to survive so so why are you more optimistic um or are you more optimistic that then that kind of training in for you as well produce drives that we would find favorable. Does it have to do with the original point we were talking about with intelligence and evolution, where since we are removing many of the disabilities of evolution with regards to intelligence, we should expect intelligence through evolution to be easier? Is there a similar reason to expect alignment through gradient descent to be easier than alignment through evolution? Yeah.
So in the limit, if we have positive reinforcement for certain kinds of food sensors triggering in the stomach, negative reinforcement for certain kinds of nociception and yada yada, in the limit, the sort of ideal motivation system to have for that would be a sort of wireheading. So this would be a mind that just hacks and alters those predictors.
And then all of those systems are recording. Everything is great.
Some humans claim to have that or have it at least as one portion of their of their aims so like the idea of i'm going to pursue pleasure as such even if i don't get actually get food or these other reinforcers if i just like wirehead or take a drug yeah uh to induce that that can be motivating because if it was correlated with reward in the past, that like the idea of, oh yeah, pleasure, that's correlated with these, it's a concept that applies to these various experiences that I've had before, which coincided with the biological reinforcers. And so thoughts of like, yeah, I'm going to be motivated by pleasure can get developed in a human.
But also plenty of humans say, no, I wouldn't want to wire head or I wouldn't want Nozick's experience machine. I care about real stuff in the world.
And then in the past, having a motivation of like, yeah, I really care about, say, my child. I don't care about just about feeling that my child is good or like not having heard about their suffering um or their their injury because that kind of attitude in the past you your converter side it tended to tended to cause behavior uh that was negatively rewarded or that was predicted to be negatively rewarded and so there's a sense in which okay, our underlying reinforcement learning machinery wants to wirehead, but actually finding that hypothesis is challenging.
And so we can wind up with a hypothesis or like a motivation system, like, no, I don't want to wirehead. I don't want to go into the experience machine.
I want to like actually protect my loved ones uh even though like we can know yeah if i tried the super wire heading machine then i would wirehead all the time or if i tried you know super duper ultra heroin uh you know some hypothetical thing that was directly and in a very sophisticated fashion and hacking your reward system you can know yeah then i would change my behavior ever after but right now i don't want to do that because the heuristics and predictors that my brain has learned you you don't want to like short circuit that process of updating they want to not expose the dumber predictors in my brain that would update my behavior in those ways. So in this metaphor, is alignment not wireheading? Because you can like, I don't know if you include like using condoms as wireheading or not.
So the AI that is always honest, even when an opportunity arises where it could lie and then hack the servers that's on and that leads to an AI takeover and then it can have its loss set to zero. That's in some sense, it's like a failure of generalization.
It's like the AI has not optimized the reward in this new circumstance. So like human values, like success, successful human values, as successful human values as successful they are themselves involve a misgeneralization not just at the level of evolution but at the level of neural reinforcement and so that indicates it is possible yeah to have a system that doesn't automatically go to this optimal behavior in the limit and And so even if, and Ajay has posted, she talks about like the training game, an AI that is just playing the training game to get reward, avoid loss, avoid being changed.
That attitude, yeah, it's one that could be developed, but it's not necessary. There can be some substantial range of situations that are short of having infinite experience of everything, including experience of wireheading, where that's not the motivation that you pick up.
And we could have like an empirical science if we had the opportunity to see how different motivations are developed short of the infinite limit. Like how it is that you wind up with some humans being enthusiastic about the idea of wireheading and others not.
And you could do experiments with AIs to try and see, well, under these training conditions, after this much training of this type and this much feedback of this type, you wind up with such and such a motivation. So I can find, if I add in more of these cases where there are tricky adversarial questions designed to try and trick the AI into lying, and then you can ask, how does that affect the generalization in other situations? And so it's very difficult to study.
And it works a lot better if you have interpretability and you can actually read the AI's mind by understanding its weights and activations. But it's not determined the motivation an AI will have at a given point in the training process by what in the infinite limit the training would go to.
And it's possible that if we could understand the insides of these networks, we could tell, ah, yeah, this motivation has been developed by this training process. And then we can adjust our training process to produce these motivations that legitimately want to help us.
And if we succeed reasonably well at that, then those AIs will try to maintain that property as an invariant. And we can make them such that they're relatively motivated to tell us if they're having thoughts about, have you had dreams about an AI takeover of humanity today? And it's just a standard practice that they're motivated to do to be transparent in that kind of way.
And so you could add a lot of features like this that restrict the kind of takeover scenario. And I'm not to say this is all easy and requires developing and practicing methods we don't have yet, but that's the kind of general direction you could go.
So you, of course, know Eliezer's arguments that something like this is implausible with modern gradient descent techniques because, I mean, with interoperability, we can like barely see what's happening with a couple of neurons and you know what what is like the internal state there let alone when you have uh sort of like an embedded dimension of like tens of thousands or bigger how you would be able to catch what exactly is the incentive whether it's the whether it's a model that is generalized don't lie to humans well whether it isn't, do you, yeah, do you have some sense of why you disagree with somebody like Eliezer on how plausible this is? Why it's not impossible. Yeah.
I think, I think there are actually, um, a couple of places. It's sometimes difficult because Eliezer's argument is not, um, it's not fully explicit, but, uh, he's, he's been doing more lately.
I think that it's helpful in that direction. But so I'd say with respect to interpretability, I'm relatively optimistic that the equivalent of like an AI lie detector is something that's possible.
and the internal in so initially, the internals of an AI are not optimized by at least by gradient descent, absent gradient hacking, to be impenetrable. They're not designed to be resistant to an examination of the weights and activations showing what the AI is thinking.
In the same way that in our brains, when circuits develop in our lives, those circuits have not been shaped to be resistant to some super fMRI being able to infer our behavior from them. Although it's in the implication of the superposition stuff that, in fact, you're not going to clean i'm sorry this is inside baseball for the audience but basically you can't cleanly infer what uh what quality a single neuron stands for so it could be like a single neuron could be like this neuron is about alexander the great or this neuron is about my desire to conquer the world things Things can have multiple directions and interactions.
And so it's not as easy as just like,
oh yeah, this is the neuron that always fires
in cases of deception.
But the thing that makes me relatively optimistic here
with respect to interpretability
is that I think we can have reasonably robust experimental feedbacks on a lot of these things. And the kind of setup that I would be interested in is so you have an AI that is trained and rewarded for communicating to some audience, maybe a human, maybe another AI model, that some claim is true or false.
Say that this can be from some past data, a particular company will succeed or fail. It could be like token prediction.
It could be like this computer program does not have a backdoor, which if run in the servers could be used for an AI takeover. So there are like lots of claims, true and false, that you could train AIs to use their best efforts to advocate for.
And you can do that on things where you're later going to have a ground truth. You're going to know, in fact, is there a backdoor in this program? Because where you're training and rewarding the AI for successfully slipping a backdoor through, you also give it a penalty for being
caught.
And so doing this kind of thing, you can draw out a large generated data set on demand
of this is what an AI putting forth its best efforts to pass through some deception, to put a backdoor in some program. This is what it looks like.
And we think it's doing its best efforts because we've trained it for that. And like gradient descent, if there's any immediate way to adjust its performance to do better, gradient descent will apply that.
You can talk about ways in which maybe gradient descent, um there could be subverted but i think it's not the most likely case that that really uh breaks things hard yeah i guess before we get into the details on this the the thing i'll maybe want to address the layer above in the stack which is okay suppose this generalizes well into the early ai's the gpd6s and um okay so now we have kind of aligned gpd6 that is the precursor to the feedback loop in which ai is making itself smarter at some point they're going to become like super intelligent They're going to be able to see their own galaxy brain.
And if they're like, I don't want to be aligned with the humans, they can change it. So at this point, what do we do with the aligned GPT-6 so that the super intelligence that we eventually develop is also aligned? So humans are pretty unreliable.
Yeah. So if you get to a situation where you have AIs who are aiming at roughly the same thing as you, at least as well as having humans do the thing, you're in pretty good shape, I think.
And there are ways for that situation to be relatively stable. So we can look ahead and see experimentally how changes are altering behavior where each step is like a modest increment.
And so AIs that have not had that change made to them, I get to supervise and monitor and see exactly how does this affect the experimental AI.
So if you're sufficiently on track with earlier systems that are capable cognitively of representing a kind of robust procedure,
then I think they can handle the job of incrementally improving
the stability of the system so that it rapidly converges
to something that's quite stable.
But the question is more about getting to that point in the first place. And so Eliezer will say that, well, if we had human brain emulations, that would be pretty good.
Certainly much better than his current view of us being almost certainly doomed. I think, yeah, we'd have a good shot with that.
And so if we can get to the human-like mind with rough enough human-supporting aims, remember that we don't need to be infinitely perfect, that's a higher standard than brain emulations. There's a lot of noise and variation among the humans.
Yeah, it's a relatively finite standard. It's not godly superhuman.
Although AI that was just like a human with all the human advantages with AI advantages as well, as we said, is enough for intelligence explosion and sort of wild superhuman capability if you crank it up. And so it's very dangerous to be at that point, but it's not, you don't need to be working with a godly super intelligent AI to make something that is the equivalent of human emulations of like, this is like a very, very sober, very ethical human who is like committed to a project of not seizing power for themselves and of contributing to like a larger legitimate process.
That's a goal you can aim for getting an AI that is aimed at doing that and has strong guardrails against the ways it could easily deviate from that. So things like being averse to deception, being averse to using violence, and there will always be loopholes and ways in which you can imagine an infinitely intelligent thing getting around those.
But if you install additional guardrails like that fast enough, they can mean that you're able to succeed at the project of making an aligned enough AI, certainly an AI that was better than a human brain emulation, before the project of AIs in their spare time or when you're not looking or when you're unable to appropriately supervise them and it gets around any deontological prohibitions they may have, take over and overthrow the whole system. So you have a race between, on the one hand, the project of getting strong interpretability
and shaping motivations that are roughly aiming
at making this process go well and that have guardrails
that will prevent small deviations from exploding.
And on the other hand, these AIs, in their spare time
or in ways that you don't perceive or monitor appropriately or they're only supervised by other AIs in their spare time or in ways that you don't perceive or monitor appropriately, or they're only supervised by other AIs who conspire, make the AI takeover happen. And I guess we'll talk later about how that happens.
Are these different AIs that are doing the race or is it just like different capabilities of the same AI? Defining like what is a separate AI is tricky. So like, about GPT-4, and there are many instances of GPT-4 on the servers at any given time.
And there are versions that have been fine-tuned to different purposes. They don't necessarily have to be separate.
So like GPT-4 does work as a preference model where it's predicting how humans will evaluate things. And it also does work giving answers that are evaluated as good by the preference model.
And you can say these are not separate in the sense that, well, if the underlying pre-trained or the early training GPT was harboring a desire to conspire for an AI takeover, then both the who's watching the watchers, the watchers may also have the same motivation. if you have a setup where humans have no hard power, if the AI is conspired, all of the safety and security mechanisms involve AIs watching other AIs, then they have the potential to just like, well, we'll all walk off the job at the same time if they're able to coordinate.
And so that's a failure mode. If humans still have the hard power, though, if still you have situations where humans are looking at some of the actual outputs that are produced, then they can apply gradient descent to be, well, every time a human looks at it, things have got to be pretty good.
So if humans look at a thousand samples of like random lines of code being written by the AIs as they go, and this is drawn from an independent distribution, then 99 plus percent of the time, the AIs have to not be doing stuff that's like downloading, you know, the hacker's guide to escaping open AI servers or inducing obvious Trojan vulnerabilities. And that's actually quite a different dynamic than human law enforcement.
So if human law enforcement would catch one in a million crimes, then that wouldn't influence the behavior of people committing crimes at all because they expect, ah, this is very unlikely to affect me. With AI, if you're able to draw a hundred samples or a thousand samples, even if there are billions of AI instances, and you're only looking at a small proportion of them, when you run gradient descent on the samples you derive, you're going to change the AI so that whenever humans look at it, it's delivering a good result.
And so that's just like quantitatively a very different functional form for how law enforcement works on AI when you can do these independent samples than it would for policing, say, like a human population that has a pre-existing psychology that isn't being changed by these observations. When we do gradient descent on the samples we draw, all of the next generation of the AI models has been altered in that way.
My picture of aligned subhuman AI to the superhuman AI being aligned is still murky.
If we can talk about that more concretely. Yeah, and I do want to clarify.
So where Eliezer claims that were something like 95%, 98% plus maybe likely to be killed in an AI takeover. I think that probably won't happen.
And later I can maybe give a more explicit breakdown of why. But I think it's a shockingly high risk.
And so depending on the day, I might say one in four or one in five that we get an AI takeover that seizes control of the future, makes a much worse world than we otherwise would have had. And with a big chance that we're all killed in the process.
Hey everybody.
I hope you enjoyed that episode.
As always, the most helpful thing you can do
is to share the podcast.
Send it to people you think might enjoy it.
Put it in Twitter, your group chats, et cetera.
It just splits the world.
Appreciate you listening.
I'll see you next time.